AUC Score :
Short-term Tactic1 :
Dominant Strategy :
Time series to forecast n:
ML Model Testing : Modular Neural Network (Speculative Sentiment Analysis)
Hypothesis Testing : Stepwise Regression
Surveillance : Major exchange and OTC
1Short-term revised.
2Time series is updated based on short-term trends.
Key Points
The S-Net ITG Agriculture USD index is projected to experience moderate growth driven by stable global demand for agricultural products. Factors like supply chain disruptions and geopolitical instability could lead to price volatility, potentially impacting the index negatively. Weather patterns and climate change remain significant risks, capable of triggering substantial price fluctuations. Furthermore, government regulations related to agriculture and trade policies pose both opportunities and potential setbacks.About S-Net ITG Agriculture USD Index
The S-Net ITG Agriculture USD Index is designed to provide investors with exposure to the performance of a diversified basket of agricultural commodity futures contracts. It is a rules-based index, meaning its composition and weighting are determined by a pre-defined methodology, aiming for transparency and replicability. The index focuses on futures contracts traded in US dollars, reflecting the common pricing currency for these commodities globally. It is rebalanced periodically, ensuring the index reflects the current market dynamics and the evolving supply and demand fundamentals within the agricultural sector.
This index offers a potential investment tool to gain exposure to a variety of agricultural products, including grains, oilseeds, and soft commodities. The composition typically includes futures contracts from the Chicago Board of Trade (CBOT), New York Mercantile Exchange (NYMEX), and other relevant exchanges. Its design enables investors to potentially track the overall performance of the agriculture market, acting as a benchmark for portfolio performance or as a component in broader commodity investment strategies. However, the index's performance will be affected by various factors, including weather patterns, global demand, and geopolitical events, as well as the inherent volatility of the underlying commodity futures markets.
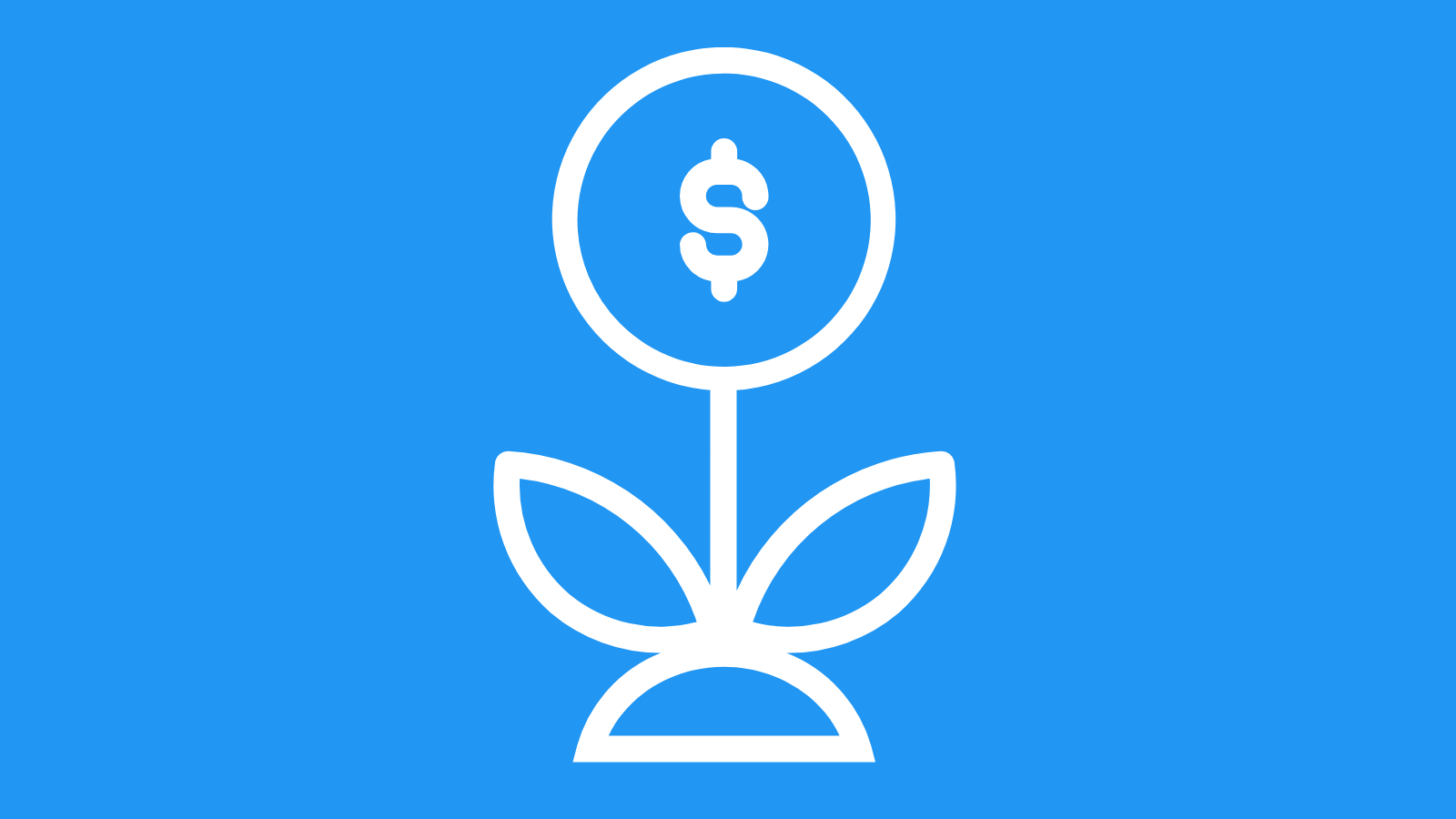
S-Net ITG Agriculture USD Index Forecast Model
Our team of data scientists and economists has developed a comprehensive machine learning model to forecast the S-Net ITG Agriculture USD index. This model employs a hybrid approach, leveraging both time-series analysis and macroeconomic indicators to achieve accurate predictions. The core of the model is built upon a foundation of Recurrent Neural Networks (RNNs), specifically Long Short-Term Memory (LSTM) networks, which are well-suited for capturing temporal dependencies within the index's historical data. We incorporate a range of features into the model, including lagged values of the S-Net ITG Agriculture USD index itself, as well as relevant economic variables such as commodity prices, exchange rates (USD-based), inflation rates, global demand indicators, and supply chain data.
To enhance the model's predictive power, we employ feature engineering techniques to transform raw data into informative features. This includes creating moving averages, volatility measures, and ratio variables to capture underlying trends and patterns. Furthermore, we utilize external data sources, such as agricultural production reports, weather patterns, and geopolitical events, to incorporate exogenous shocks that can significantly impact the index. The model undergoes rigorous training and validation using a robust dataset spanning several years, employing techniques like cross-validation to mitigate overfitting and ensure generalization to unseen data. Hyperparameter tuning is performed to optimize the model's performance, considering metrics like Mean Absolute Error (MAE), Root Mean Squared Error (RMSE), and R-squared.
The final output of the model is a forecast of the S-Net ITG Agriculture USD index, including point estimates and confidence intervals, over a specified time horizon. We have designed the model to provide forecasts ranging from short-term (e.g., daily or weekly) to medium-term (e.g., monthly or quarterly) horizons. To further improve reliability, we implement ensemble methods, combining the predictions of multiple models with different architectures and feature sets. This approach mitigates the risk of any single model's limitations. Regular monitoring and recalibration of the model are performed to ensure its continued accuracy and adaptability to changing market conditions. The model will be continuously updated with new data and insights to maintain its efficacy, providing valuable insights for investors, policymakers, and other stakeholders.
```
ML Model Testing
n:Time series to forecast
p:Price signals of S-Net ITG Agriculture USD index
j:Nash equilibria (Neural Network)
k:Dominated move of S-Net ITG Agriculture USD index holders
a:Best response for S-Net ITG Agriculture USD target price
For further technical information as per how our model work we invite you to visit the article below:
How do KappaSignal algorithms actually work?
S-Net ITG Agriculture USD Index Forecast Strategic Interaction Table
Strategic Interaction Table Legend:
X axis: *Likelihood% (The higher the percentage value, the more likely the event will occur.)
Y axis: *Potential Impact% (The higher the percentage value, the more likely the price will deviate.)
Z axis (Grey to Black): *Technical Analysis%
S-Net ITG Agriculture USD Index: Financial Outlook and Forecast
The S-Net ITG Agriculture USD Index, which tracks the performance of a basket of agricultural commodities priced in US dollars, presents a nuanced financial outlook characterized by both opportunities and challenges. Several key factors are driving this outlook. Global population growth, particularly in developing economies, continues to increase demand for food staples, supporting prices for many of the commodities within the index. Concurrently, shifting dietary preferences towards more resource-intensive foods, such as meat and dairy, are further boosting demand for grains and oilseeds used in animal feed. Furthermore, geopolitical instability, climate change, and weather patterns influence agricultural production. These factors impact supply chains, creating volatility in commodity markets. The index's performance is sensitive to these global events.
The financial forecast for the S-Net ITG Agriculture USD Index will depend on several key dynamics. Production levels in major agricultural producing regions will be crucial, as droughts, floods, and other extreme weather events can significantly disrupt harvests. Furthermore, the strength of the US dollar will affect the index's performance. A weakening dollar tends to make dollar-denominated commodities more attractive to international buyers, potentially boosting prices. The index is influenced by trends in agricultural technology, such as precision farming and biotechnology, that can improve yields and efficiency. Government policies related to agricultural subsidies, trade regulations, and biofuel mandates also significantly influence the index's components.
Analyzing the underlying drivers of the S-Net ITG Agriculture USD Index allows investors to assess its potential prospects. The index's diversified structure, encompassing a range of agricultural commodities, can provide valuable diversification benefits. The inherent demand inelasticity of food products during times of geopolitical instability and economic uncertainty, tends to make the agriculture sector more stable than other sectors. Investors should consider that agricultural investments can be volatile. This volatility is influenced by factors, such as commodity prices which respond to global events and weather patterns. The influence of sustainability factors is growing, with investors increasingly interested in environmental impacts and ethical sourcing.
Overall, the outlook for the S-Net ITG Agriculture USD Index is moderately positive. We anticipate continued demand growth for agricultural commodities driven by global population trends and evolving consumption patterns. The index's performance will be subject to volatility from unforeseen weather events. It is important for investors to consider the potential for price fluctuations. Investors should also be aware of geopolitical risks that could disrupt supply chains, impacting the index's performance. Additionally, trade policies and tariffs are significant risk factors. The index could encounter significant headwinds if global trade barriers are erected. As such, investors should conduct due diligence before investing.
```
Rating | Short-Term | Long-Term Senior |
---|---|---|
Outlook | B2 | B1 |
Income Statement | B2 | Baa2 |
Balance Sheet | Caa2 | Baa2 |
Leverage Ratios | C | Caa2 |
Cash Flow | Baa2 | C |
Rates of Return and Profitability | B2 | Caa2 |
*An aggregate rating for an index summarizes the overall sentiment towards the companies it includes. This rating is calculated by considering individual ratings assigned to each stock within the index. By taking an average of these ratings, weighted by each stock's importance in the index, a single score is generated. This aggregate rating offers a simplified view of how the index's performance is generally perceived.
How does neural network examine financial reports and understand financial state of the company?
References
- Holland PW. 1986. Statistics and causal inference. J. Am. Stat. Assoc. 81:945–60
- Chernozhukov V, Newey W, Robins J. 2018c. Double/de-biased machine learning using regularized Riesz representers. arXiv:1802.08667 [stat.ML]
- G. J. Laurent, L. Matignon, and N. L. Fort-Piat. The world of independent learners is not Markovian. Int. J. Know.-Based Intell. Eng. Syst., 15(1):55–64, 2011
- E. Altman. Constrained Markov decision processes, volume 7. CRC Press, 1999
- Varian HR. 2014. Big data: new tricks for econometrics. J. Econ. Perspect. 28:3–28
- V. Mnih, K. Kavukcuoglu, D. Silver, A. Rusu, J. Veness, M. Bellemare, A. Graves, M. Riedmiller, A. Fidjeland, G. Ostrovski, S. Petersen, C. Beattie, A. Sadik, I. Antonoglou, H. King, D. Kumaran, D. Wierstra, S. Legg, and D. Hassabis. Human-level control through deep reinforcement learning. Nature, 518(7540):529–533, 02 2015.
- Chernozhukov V, Chetverikov D, Demirer M, Duflo E, Hansen C, Newey W. 2017. Double/debiased/ Neyman machine learning of treatment effects. Am. Econ. Rev. 107:261–65