AUC Score :
Short-term Tactic1 :
Dominant Strategy :
Time series to forecast n:
ML Model Testing : Supervised Machine Learning (ML)
Hypothesis Testing : Wilcoxon Sign-Rank Test
Surveillance : Major exchange and OTC
1Short-term revised.
2Time series is updated based on short-term trends.
Key Points
The S&P Ethereum index is anticipated to experience continued volatility, potentially exhibiting both significant gains and sharp corrections. This volatility stems from the inherent speculative nature of the cryptocurrency market, as well as external factors such as regulatory developments, macroeconomic trends, and technological advancements within the Ethereum ecosystem. The primary prediction is sustained price fluctuations, with upward movements driven by increased institutional adoption, positive developments in scaling solutions, and growing demand. Conversely, substantial downside risk exists due to potential regulatory crackdowns, negative investor sentiment, security vulnerabilities, and broader economic downturns. The overall trajectory is inherently uncertain, contingent upon a complex interplay of market forces and the evolving landscape of the digital asset space.About S&P Ethereum Index
The S&P Ethereum Index serves as a benchmark designed to track the performance of the Ethereum digital asset. This index provides investors with a standardized and transparent method to gauge the market's overall movement and potentially compare the performance of various Ethereum-related investment products. Its construction methodology typically involves sophisticated processes to ensure accurate representation of the Ethereum market and its characteristics. The index's data is often used for various financial purposes, including but not limited to, the creation of investment products and market analysis.
S&P Dow Jones Indices, known for its reputable and globally recognized indices, is the publisher of the S&P Ethereum Index. The index construction and methodology are transparent and publicly available, following established standards of index design. Periodic reviews and rebalancing are commonly performed to maintain the index's relevance and accuracy. The S&P Ethereum Index, along with other cryptocurrency indices from S&P Dow Jones Indices, offers a robust framework for understanding and navigating the evolving landscape of digital assets.
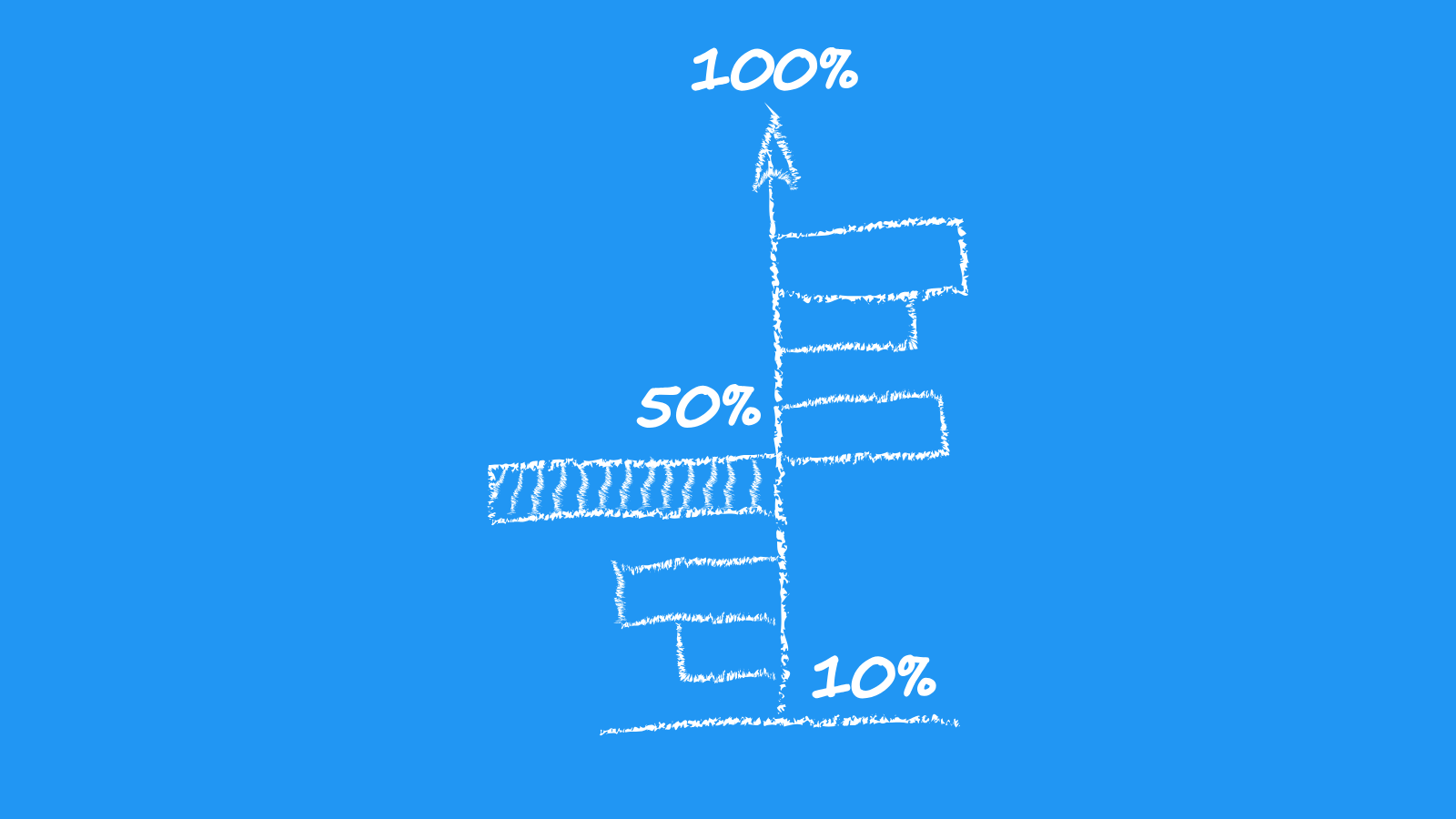
S&P Ethereum Index Forecast Model
Our team of data scientists and economists proposes a comprehensive machine learning model for forecasting the S&P Ethereum Index. The model leverages a diverse range of time-series data, including historical trading volumes, volatility measures (e.g., realized volatility, implied volatility from options markets), and macroeconomic indicators such as inflation rates, interest rates, and global economic growth data. Furthermore, we incorporate on-chain metrics specific to Ethereum, such as the total value locked (TVL) in DeFi protocols, the number of active addresses, gas fees, and the growth of decentralized applications (dApps). Sentiment analysis derived from social media platforms and news articles related to Ethereum will also be incorporated to capture market sentiment and predict potential shifts in investor behavior. This multifaceted approach is intended to create a robust and accurate forecast.
The model will employ a combination of advanced machine learning algorithms. We plan to utilize a hybrid approach, starting with feature engineering to transform the raw data into meaningful features. These will be fed into Recurrent Neural Networks (RNNs), specifically Long Short-Term Memory (LSTM) networks, which are known for their ability to capture temporal dependencies in time-series data. Additionally, we will experiment with Gradient Boosting Machines (GBMs), like XGBoost and LightGBM, to consider the model's interpretability and handling of complex interactions between variables. The model's performance will be rigorously evaluated using various metrics, including Mean Squared Error (MSE), Root Mean Squared Error (RMSE), and Mean Absolute Error (MAE), alongside backtesting simulations across diverse market conditions. Regularization techniques and cross-validation strategies will be employed to prevent overfitting and ensure the model's generalizability.
The output of the model will provide a predicted index value for a specified future period. The model's forecasts will be regularly updated with fresh data to reflect the most current market information. We intend to provide probabilistic forecasts, i.e., generating predicted values along with confidence intervals. The team plans to continuously refine the model by incorporating newly emerging data sources (such as Layer-2 data), fine-tuning the model parameters, and evaluating alternative machine learning techniques to ensure our predictions are as precise as possible. Regular model performance reviews and stakeholder feedback are crucial to guarantee that our forecasting model continues to add value to the index tracking and its derivative products.
ML Model Testing
n:Time series to forecast
p:Price signals of S&P Ethereum index
j:Nash equilibria (Neural Network)
k:Dominated move of S&P Ethereum index holders
a:Best response for S&P Ethereum target price
For further technical information as per how our model work we invite you to visit the article below:
How do KappaSignal algorithms actually work?
S&P Ethereum Index Forecast Strategic Interaction Table
Strategic Interaction Table Legend:
X axis: *Likelihood% (The higher the percentage value, the more likely the event will occur.)
Y axis: *Potential Impact% (The higher the percentage value, the more likely the price will deviate.)
Z axis (Grey to Black): *Technical Analysis%
S&P Ethereum Index: Financial Outlook and Forecast
The S&P Ethereum Index, representing the performance of the Ethereum cryptocurrency, reflects the financial outlook of a rapidly evolving digital asset market. Analysis of the index necessitates consideration of several key factors. Firstly, the overall sentiment toward cryptocurrencies, driven by regulatory developments, institutional adoption, and macroeconomic conditions, significantly influences the index's trajectory. Secondly, the Ethereum network's own fundamentals play a critical role. This includes the success of the ongoing upgrades (like the "Merge" which has already happened), and the implementation of scaling solutions which reduce the gas fees. In addition, the growth of decentralized finance (DeFi) applications and non-fungible tokens (NFTs) on the Ethereum platform directly impacts the index's performance. Investors will also keep a close eye on the competitive landscape, including other blockchain platforms and how Ethereum manages to maintain its dominant position.
Examining specific aspects of the market reveals additional layers of complexity. Technological advancements within the Ethereum ecosystem are crucial. The successful implementation of upgrades which improve network speed, and reduce energy consumption, will likely boost investor confidence and drive demand. The expansion of DeFi applications and their increasing TVL (Total Value Locked) indicates the growing utility of the platform. The Ethereum index closely reflects the adoption rate of these applications. The supply dynamics of Ethereum, including the effects of "burning" which decreases the supply of the asset, also influences the price. Finally, the global economic outlook must also be observed: inflation, interest rates, and potential economic downturns. They can all impact the appetite of investors towards the riskier assets like Ethereum.
In evaluating the financial outlook for the S&P Ethereum Index, it's essential to consider long-term trends and market cycles. While the volatility inherent in the cryptocurrency market presents short-term risks, the underlying trends suggest promising long-term potential. The increasing institutional interest in Ethereum, as well as the continued development of new DeFi and NFT applications, paints a positive picture. Over the medium to long term, the index's performance is intrinsically linked to the ability of Ethereum to maintain its position as the leading smart contract platform. Ethereum's ability to adapt to changing regulatory landscapes, the competitiveness within the blockchain space, and its ability to integrate innovative technologies will be key to its success. Ethereum faces competition from platforms such as Solana, Cardano, and Polkadot and also technological challenges.
Based on the factors discussed, a positive outlook for the S&P Ethereum Index is predicted, with the expectation of moderate growth over the next 12-24 months. This forecast assumes continued advancements in the Ethereum network, mainstream adoption of DeFi and NFT applications, and a more favorable regulatory environment. However, several risks could undermine this prediction. These risks include the possibility of a significant economic downturn, new regulations that negatively impact the cryptocurrency market, the emergence of more powerful and scalable competing blockchain platforms, the existence of smart contract bugs, and the increased competition from other Layer 2 protocols. Successful mitigation of these challenges is essential for realizing the optimistic forecast.
```
Rating | Short-Term | Long-Term Senior |
---|---|---|
Outlook | B3 | B1 |
Income Statement | C | Ba3 |
Balance Sheet | C | Caa2 |
Leverage Ratios | B3 | Ba2 |
Cash Flow | Baa2 | B1 |
Rates of Return and Profitability | Caa2 | B3 |
*An aggregate rating for an index summarizes the overall sentiment towards the companies it includes. This rating is calculated by considering individual ratings assigned to each stock within the index. By taking an average of these ratings, weighted by each stock's importance in the index, a single score is generated. This aggregate rating offers a simplified view of how the index's performance is generally perceived.
How does neural network examine financial reports and understand financial state of the company?
References
- Greene WH. 2000. Econometric Analysis. Upper Saddle River, N J: Prentice Hall. 4th ed.
- V. Borkar. Stochastic approximation: a dynamical systems viewpoint. Cambridge University Press, 2008
- Mnih A, Hinton GE. 2007. Three new graphical models for statistical language modelling. In International Conference on Machine Learning, pp. 641–48. La Jolla, CA: Int. Mach. Learn. Soc.
- LeCun Y, Bengio Y, Hinton G. 2015. Deep learning. Nature 521:436–44
- Robins J, Rotnitzky A. 1995. Semiparametric efficiency in multivariate regression models with missing data. J. Am. Stat. Assoc. 90:122–29
- P. Milgrom and I. Segal. Envelope theorems for arbitrary choice sets. Econometrica, 70(2):583–601, 2002
- Chen X. 2007. Large sample sieve estimation of semi-nonparametric models. In Handbook of Econometrics, Vol. 6B, ed. JJ Heckman, EE Learner, pp. 5549–632. Amsterdam: Elsevier