AUC Score :
Short-term Tactic1 :
Dominant Strategy :
Time series to forecast n:
ML Model Testing : Modular Neural Network (Market News Sentiment Analysis)
Hypothesis Testing : Pearson Correlation
Surveillance : Major exchange and OTC
1Short-term revised.
2Time series is updated based on short-term trends.
Key Points
Rezolve AI shares are projected to experience moderate volatility in the near term, driven by its innovative AI technology and market adoption. The company's expansion plans and strategic partnerships are expected to contribute to positive growth, with potential for significant upside if it successfully penetrates new markets. However, risks include intense competition in the AI sector, the possibility of slower-than-anticipated revenue growth, and the need for substantial capital investment to scale its operations. Regulatory changes and the impact of economic downturns also pose potential challenges.About Rezolve AI Limited
Rezolve AI Limited (Rezolve), is a technology company specializing in artificial intelligence solutions. The company develops and deploys AI-powered platforms designed to enhance customer engagement and streamline business processes. Their offerings span across various sectors, focusing on areas such as mobile commerce, customer interaction, and data analytics. Rezolve's core technology revolves around providing businesses with tools to understand consumer behavior, personalize experiences, and drive sales through its AI-driven applications. They aim to create seamless and intelligent interactions for both businesses and their customers.
Rezolve primarily serves businesses seeking to leverage AI for improved efficiency and customer relationship management. The company focuses on delivering innovative solutions that enable companies to adapt to the evolving digital landscape. Their services include the development and implementation of AI-driven platforms to improve marketing strategies, optimize sales performance, and enhance customer service operations. Rezolve strives to empower businesses to make data-driven decisions, personalize customer experiences, and gain a competitive edge in their respective industries.
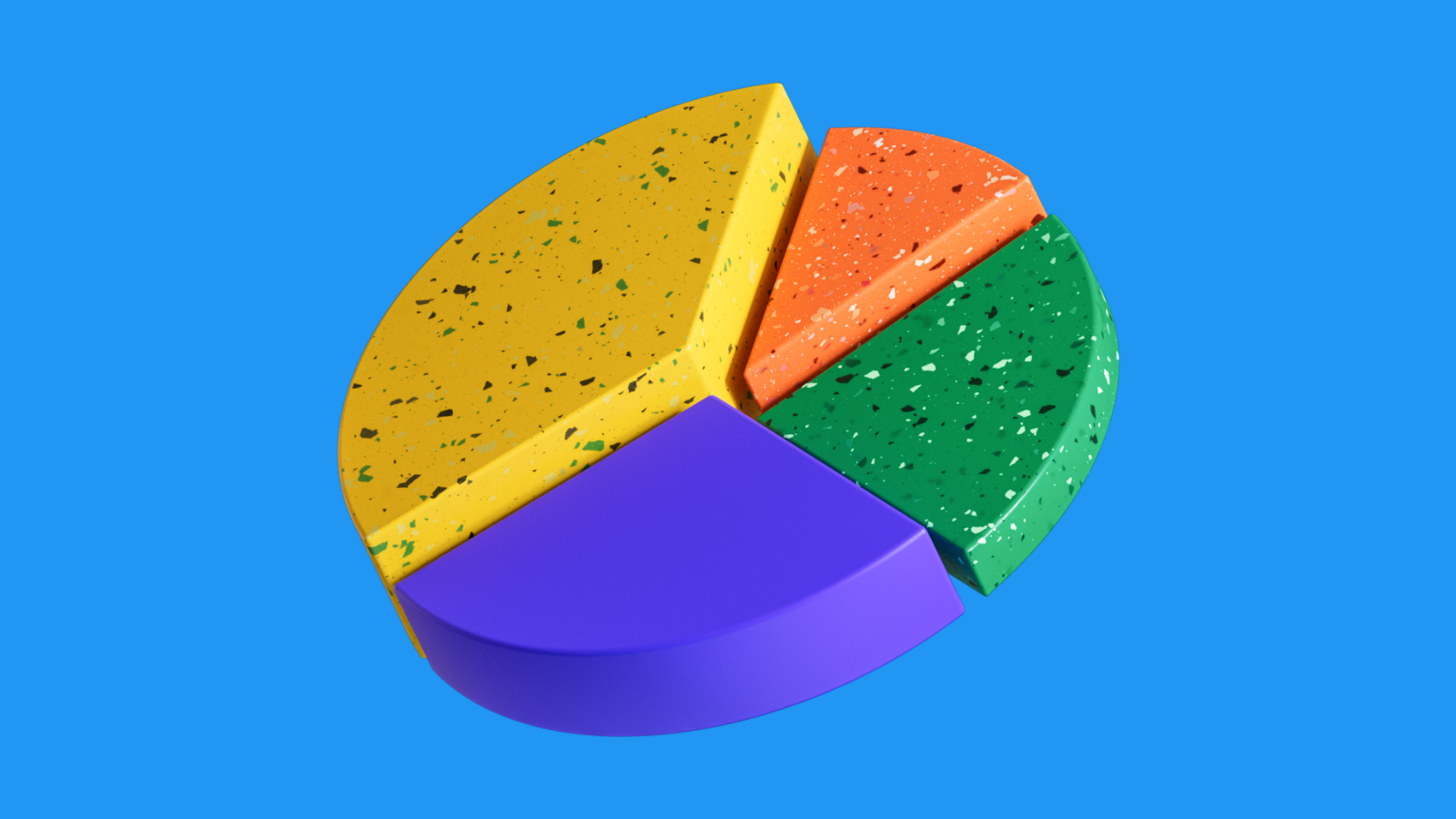
RZLV Stock Forecast Model
Our team, comprising data scientists and economists, has developed a machine learning model designed to forecast the performance of Rezolve AI Limited Ordinary Shares (RZLV). The model leverages a comprehensive dataset incorporating a variety of economic and market indicators. These include macroeconomic variables like inflation rates, GDP growth, and interest rate changes, alongside industry-specific data such as competitor performance, technological advancements in AI, and market sentiment. Furthermore, the model integrates RZLV-specific financial data, including revenue reports, earnings per share, and debt levels. The model is trained on historical data, allowing it to recognize patterns and correlations between these variables and RZLV's future performance.
The core of our model consists of a hybrid architecture that combines the strengths of several machine learning techniques. We employ a combination of Recurrent Neural Networks (RNNs), which are well-suited for time-series data, and Gradient Boosting algorithms to enhance predictive accuracy. RNNs are instrumental in analyzing the sequential nature of market data, capturing temporal dependencies. Gradient Boosting algorithms, on the other hand, improve accuracy by combining multiple weak learners. This ensemble approach mitigates the limitations of any single algorithm and yields a more robust forecast. The model undergoes rigorous validation using techniques like cross-validation and backtesting to ensure reliability and reduce overfitting risks.
The model's output is a probabilistic forecast, providing not only a prediction of the RZLV's future direction but also a measure of the associated uncertainty. This is crucial for providing investors with a realistic understanding of the potential risks involved. Our team will regularly monitor and retrain the model with new data to maintain its accuracy and adaptability to changing market conditions. The model is designed to be a dynamic tool that provides valuable information for informed investment decisions, but should not be considered as the only factor and used in conjunction with other investment strategies and due diligence.
ML Model Testing
n:Time series to forecast
p:Price signals of Rezolve AI Limited stock
j:Nash equilibria (Neural Network)
k:Dominated move of Rezolve AI Limited stock holders
a:Best response for Rezolve AI Limited target price
For further technical information as per how our model work we invite you to visit the article below:
How do KappaSignal algorithms actually work?
Rezolve AI Limited Stock Forecast (Buy or Sell) Strategic Interaction Table
Strategic Interaction Table Legend:
X axis: *Likelihood% (The higher the percentage value, the more likely the event will occur.)
Y axis: *Potential Impact% (The higher the percentage value, the more likely the price will deviate.)
Z axis (Grey to Black): *Technical Analysis%
Rezolve AI: Financial Outlook and Forecast
The financial outlook for Rezolve AI (RZV) appears promising, underpinned by its innovative technology and the expanding market for digital commerce solutions. The company's core business revolves around providing a platform that enables seamless and interactive shopping experiences via mobile devices. This strategy is well-aligned with the increasing consumer preference for mobile commerce, suggesting a substantial addressable market. Furthermore, RZV's focus on enhancing customer engagement through personalized experiences and its ability to facilitate frictionless transactions are key differentiators. Financial projections will likely reflect robust revenue growth driven by subscription-based models, transaction fees, and potential for expansion into new geographical markets and product offerings. The company's partnerships and collaborations with prominent industry players also serve as a catalyst for growth, providing access to established distribution channels and a broader customer base. Key performance indicators (KPIs) like customer acquisition cost, customer lifetime value, and churn rate will be crucial in assessing the company's ability to drive profitability.
Forecasts for RZV suggest continued strong revenue growth in the short to medium term. Analysts are likely to project consistent expansion in user base, leading to increased transaction volumes and higher revenues. Factors such as increased adoption of mobile payments, the expanding e-commerce landscape, and the growing need for businesses to provide enhanced customer experiences are expected to be tailwinds for the business. The company's ability to secure significant contracts with key retailers and brands would boost financial projections. Profitability metrics, while potentially starting modestly due to investments in growth and scaling operations, should improve over time as the company achieves economies of scale and optimizes its cost structure. Furthermore, the company may seek to leverage its technology for additional applications beyond pure commerce, such as in-store mobile interactions, further expanding its revenue streams. Investors will carefully examine the company's progress in achieving profitability, managing cash flow, and reducing debt.
Important considerations in RZV's financial outlook include its ability to maintain a competitive edge in a rapidly evolving technological landscape. The company must continue to innovate and enhance its platform to address evolving consumer expectations and counter potential competition from established players in the digital commerce ecosystem. Effective management of operating expenses, especially in research and development and sales and marketing, will be critical to optimizing profitability. Furthermore, RZV will need to carefully monitor and adapt to changes in regulatory requirements and data privacy guidelines. Investors will scrutinize the company's ability to build strategic partnerships, successfully integrate acquired businesses (if any), and effectively manage its capital allocation. Careful management of the company's debt and ensuring a healthy cash flow are also crucial for long-term financial stability.
The prediction for RZV's financial future is generally positive. Based on its core business model and the expanding market for mobile commerce solutions, significant revenue and user growth is anticipated in the next few years. However, there are risks to this prediction. The primary risk lies in competition from existing and new market participants, which could exert downward pressure on pricing or require increased investments in marketing to attract and retain customers. Another risk is the evolving nature of technology, where changes could render the platform obsolete if not innovated consistently. Geopolitical factors, shifts in consumer trends, economic downturns, and regulatory changes could also negatively affect the company's performance. Nevertheless, the strong foundation and strategic position of RZV, coupled with its commitment to innovation, position the company well for sustainable growth.
Rating | Short-Term | Long-Term Senior |
---|---|---|
Outlook | Baa2 | Ba3 |
Income Statement | Baa2 | Baa2 |
Balance Sheet | Baa2 | C |
Leverage Ratios | B3 | Baa2 |
Cash Flow | Baa2 | Caa2 |
Rates of Return and Profitability | Baa2 | Baa2 |
*Financial analysis is the process of evaluating a company's financial performance and position by neural network. It involves reviewing the company's financial statements, including the balance sheet, income statement, and cash flow statement, as well as other financial reports and documents.
How does neural network examine financial reports and understand financial state of the company?
References
- R. Williams. Simple statistical gradient-following algorithms for connectionist reinforcement learning. Ma- chine learning, 8(3-4):229–256, 1992
- Hornik K, Stinchcombe M, White H. 1989. Multilayer feedforward networks are universal approximators. Neural Netw. 2:359–66
- LeCun Y, Bengio Y, Hinton G. 2015. Deep learning. Nature 521:436–44
- M. J. Hausknecht. Cooperation and Communication in Multiagent Deep Reinforcement Learning. PhD thesis, The University of Texas at Austin, 2016
- V. Borkar. Stochastic approximation: a dynamical systems viewpoint. Cambridge University Press, 2008
- Breusch, T. S. (1978), "Testing for autocorrelation in dynamic linear models," Australian Economic Papers, 17, 334–355.
- Breiman L. 2001a. Random forests. Mach. Learn. 45:5–32