AUC Score :
Short-term Tactic1 :
Dominant Strategy :
Time series to forecast n:
ML Model Testing : Transductive Learning (ML)
Hypothesis Testing : Spearman Correlation
Surveillance : Major exchange and OTC
1Short-term revised.
2Time series is updated based on short-term trends.
Key Points
Red River's future performance is projected to be relatively stable, driven by its regional focus and conservative lending practices. The company is expected to experience moderate loan growth, along with stable net interest margins, due to increasing interest rates and a healthy local economy. The company's success depends on its ability to manage credit risk effectively, particularly in sectors like commercial real estate where market volatility is more pronounced. Expansion into new markets could provide further opportunities, but it also presents execution risk. Increased competition from larger regional and national banks and the potential for economic downturns are significant risks. Furthermore, evolving regulatory requirements could negatively impact profitability.About Red River Bancshares
Red River Bancshares, Inc. (RRBI) is a Louisiana-based bank holding company providing a range of financial services primarily within the state. RRBI operates through its subsidiary, Red River Bank. The bank offers services including commercial and consumer lending, deposit accounts, and wealth management solutions. Its focus is on serving the financial needs of individuals, small to medium-sized businesses, and commercial clients within its defined market area. The company's operations center on providing traditional banking services and building relationships with its customers to foster sustainable growth and community support.
RRBI emphasizes local market expertise and personalized service to distinguish itself. The company's strategy involves organic growth through expanding its customer base and enhancing its product offerings. Red River Bank aims to maintain a strong financial position and adapt to evolving market trends. RRBI's commitment is to serving the communities in which it operates by providing financial resources and contributing to local economic development.
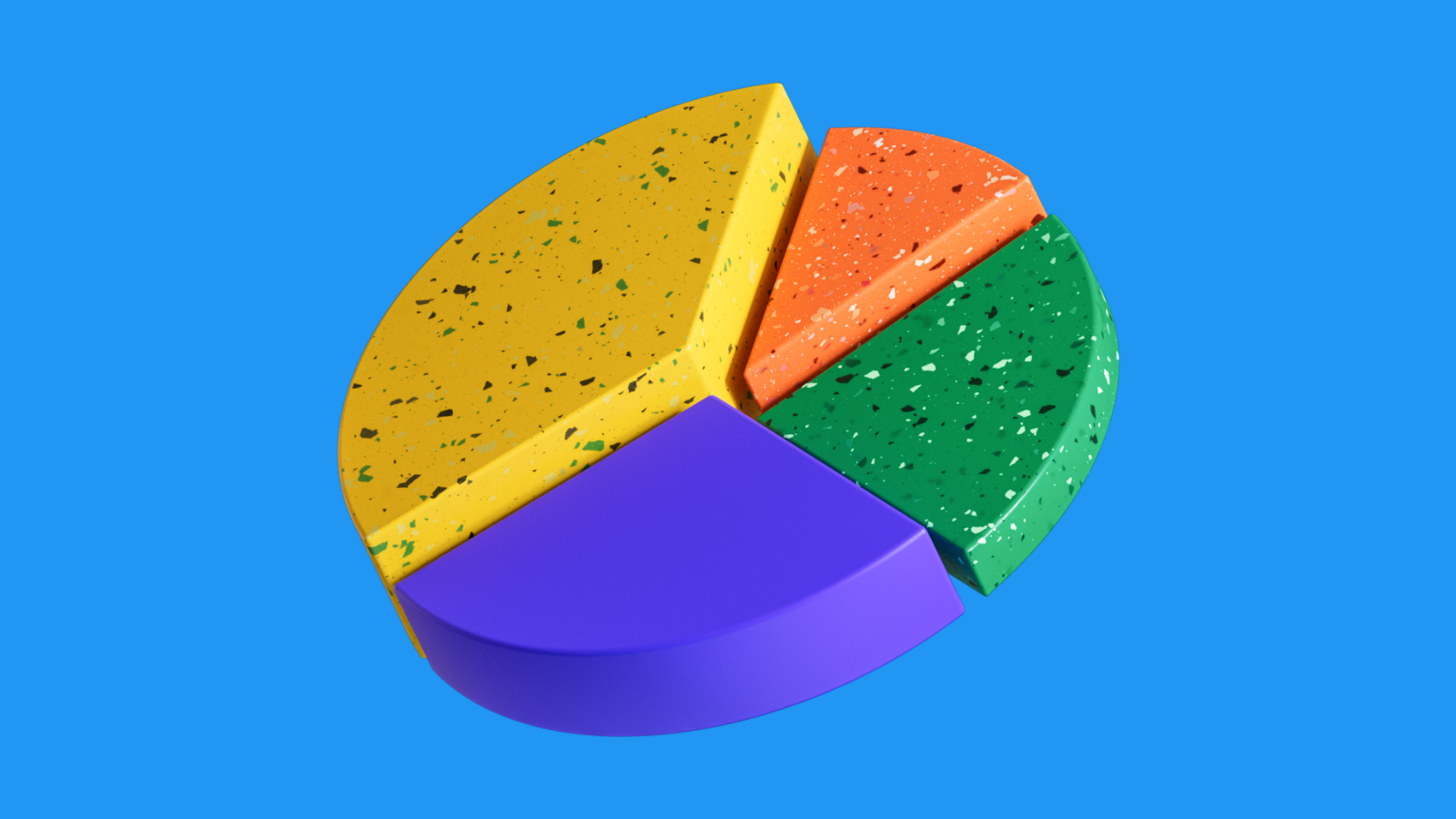
RRBI Stock Forecast Model: A Data Science and Economic Approach
Our multidisciplinary team of data scientists and economists proposes a comprehensive machine learning model to forecast the performance of Red River Bancshares Inc. (RRBI) common stock. The model leverages a diverse dataset encompassing financial statement metrics (e.g., revenue, net income, earnings per share, book value, and debt-to-equity ratio), macroeconomic indicators (e.g., interest rates, inflation, GDP growth, and unemployment rate), and market sentiment data (e.g., analyst ratings, news sentiment scores, and social media activity). We will employ feature engineering techniques to derive valuable insights from the raw data, transforming variables to enhance their predictive power. Specifically, we will incorporate lagged values of the financial and economic indicators to capture temporal dependencies. The model will be designed to forecast RRBI's stock performance over a defined timeframe, incorporating both short-term (e.g., daily or weekly) and medium-term (e.g., monthly or quarterly) predictions.
The core of our model will employ a hybrid machine learning approach. Initially, we will utilize a combination of time series analysis techniques such as ARIMA, Exponential Smoothing, and potentially, Vector Autoregression (VAR) models to capture the intrinsic patterns and trends within the historical RRBI stock data. Simultaneously, we will build a suite of supervised learning models including Random Forests, Gradient Boosting Machines (e.g., XGBoost or LightGBM), and Support Vector Machines (SVM) to capture non-linear relationships between input features and stock performance. To mitigate the risks associated with overfitting, a rigorous cross-validation strategy will be used. The final forecast will be a weighted ensemble of the base models, which will incorporate both the time series analysis and the machine learning results for the best forecast. This ensemble approach is designed to exploit the strengths of each model while compensating for any weaknesses, leading to a more robust and reliable forecast.
The model's performance will be rigorously evaluated using established statistical measures, including Mean Squared Error (MSE), Root Mean Squared Error (RMSE), Mean Absolute Error (MAE), and R-squared. Furthermore, we will assess the model's directional accuracy by evaluating its ability to predict the direction of price movement (e.g., upward or downward). The model's performance will be benchmarked against relevant industry indexes and publicly available consensus forecasts. Crucially, the model will be regularly updated and recalibrated with new data to maintain its predictive accuracy. Additionally, we will conduct sensitivity analyses to identify the most influential factors driving RRBI's stock performance. The output of the model will include point estimates, probability distributions for those estimates, and a set of risk and uncertainty measures. This holistic approach ensures the delivery of a practical and reliable forecast for RRBI's stock performance.
ML Model Testing
n:Time series to forecast
p:Price signals of Red River Bancshares stock
j:Nash equilibria (Neural Network)
k:Dominated move of Red River Bancshares stock holders
a:Best response for Red River Bancshares target price
For further technical information as per how our model work we invite you to visit the article below:
How do KappaSignal algorithms actually work?
Red River Bancshares Stock Forecast (Buy or Sell) Strategic Interaction Table
Strategic Interaction Table Legend:
X axis: *Likelihood% (The higher the percentage value, the more likely the event will occur.)
Y axis: *Potential Impact% (The higher the percentage value, the more likely the price will deviate.)
Z axis (Grey to Black): *Technical Analysis%
Red River Bancshares Inc. (RRBI) Financial Outlook and Forecast
RRBI, a financial institution primarily focused on serving the needs of businesses and individuals in Louisiana and Texas, exhibits a moderate outlook for future financial performance. The company's strategy centers on organic growth, focusing on expanding its loan portfolio while maintaining a strong emphasis on customer relationships. Recent performance indicates steady loan growth, particularly within its commercial and industrial lending segment, coupled with improvements in net interest margins as interest rates have risen. Furthermore, RRBI has been actively investing in technology and digital banking platforms, positioning itself to enhance operational efficiency and provide more convenient services to its customer base. The bank's asset quality remains a key strength, reflected in manageable non-performing loans and provisions for loan losses. However, the company operates in a competitive banking landscape, where larger institutions and regional players are vying for market share.
The company's financial forecasts are influenced by several key factors. Firstly, prevailing macroeconomic conditions, including interest rate movements and economic growth within its service areas, will play a significant role in determining loan demand and the ability to generate income. Any fluctuations in the economy of Louisiana and Texas could directly impact the performance of its loan portfolio and overall earnings. Secondly, RRBI's ability to manage its operating expenses effectively and maintain its strong asset quality will be critical for profitability. The company must continue to invest in technology and maintain its competitive position by attracting and retaining qualified personnel. Thirdly, the competitive landscape is another determining factor. The ability to acquire new customers, retain existing ones, and successfully compete against larger banks in the area will also be essential.
Industry analysts predict a steady, albeit gradual, growth trajectory for RRBI over the next few years. Revenue growth is anticipated to be driven by a combination of loan growth and improved net interest margins. The management's strategy for cost management and strategic investment in technology, along with efforts to expand the bank's geographic reach and attract new customers, is expected to support this growth. The analysts forecast a moderately positive outlook, emphasizing the company's focus on local market expertise and a personalized customer experience, which gives them an advantage. The financial forecasts also consider the potential impact of changing regulations within the financial industry, like the impact of inflation and potential economic downturns, which can impact the profitability and financial stability of banking institutions.
In conclusion, RRBI is anticipated to show solid financial performance. The company is expected to grow revenue and maintain its overall profitability. The risks associated with this positive outlook include the potential for slower-than-anticipated loan growth in its market areas, increased competition from other financial institutions, and a possible economic downturn. Furthermore, the possibility of changes in the regulatory environment poses another area of risk. RRBI's success will heavily rely on management's capability to respond effectively to economic changes and regulatory challenges, and they will need to continue investing in technology and customer service to solidify its position within the market.
Rating | Short-Term | Long-Term Senior |
---|---|---|
Outlook | Ba3 | B3 |
Income Statement | C | B2 |
Balance Sheet | Baa2 | Caa2 |
Leverage Ratios | Baa2 | Caa2 |
Cash Flow | Caa2 | B3 |
Rates of Return and Profitability | Baa2 | Caa2 |
*Financial analysis is the process of evaluating a company's financial performance and position by neural network. It involves reviewing the company's financial statements, including the balance sheet, income statement, and cash flow statement, as well as other financial reports and documents.
How does neural network examine financial reports and understand financial state of the company?
References
- Clements, M. P. D. F. Hendry (1995), "Forecasting in cointegrated systems," Journal of Applied Econometrics, 10, 127–146.
- Miller A. 2002. Subset Selection in Regression. New York: CRC Press
- Lai TL, Robbins H. 1985. Asymptotically efficient adaptive allocation rules. Adv. Appl. Math. 6:4–22
- Chernozhukov V, Chetverikov D, Demirer M, Duflo E, Hansen C, et al. 2018a. Double/debiased machine learning for treatment and structural parameters. Econom. J. 21:C1–68
- J. N. Foerster, Y. M. Assael, N. de Freitas, and S. Whiteson. Learning to communicate with deep multi-agent reinforcement learning. In Advances in Neural Information Processing Systems 29: Annual Conference on Neural Information Processing Systems 2016, December 5-10, 2016, Barcelona, Spain, pages 2137–2145, 2016.
- M. Puterman. Markov Decision Processes: Discrete Stochastic Dynamic Programming. Wiley, New York, 1994.
- Ashley, R. (1983), "On the usefulness of macroeconomic forecasts as inputs to forecasting models," Journal of Forecasting, 2, 211–223.