AUC Score :
Short-term Tactic1 :
Dominant Strategy :
Time series to forecast n:
ML Model Testing : Multi-Instance Learning (ML)
Hypothesis Testing : Chi-Square
Surveillance : Major exchange and OTC
1Short-term revised.
2Time series is updated based on short-term trends.
Key Points
RVL faces an uncertain future, potentially experiencing moderate growth driven by increased demand for AI-powered rail safety solutions. Expansion into new markets and successful partnerships could positively impact revenue streams, leading to share price appreciation. However, the company's financial performance is heavily dependent on securing large contracts, thus failure to meet project timelines or secure sufficient funding presents significant risks. Competition within the rail technology sector and the need for regulatory approvals in various jurisdictions could pose further challenges, potentially limiting growth and impacting investor confidence.About Rail Vision
Rail Vision Ltd. is an Israeli technology company specializing in the development and implementation of AI-powered, sensor-based systems designed to enhance safety, efficiency, and predictive maintenance in the railway industry. The company's primary focus is on providing advanced solutions for obstacle detection, track inspections, and other critical functions aimed at preventing accidents and reducing operational costs. Their core technology integrates electro-optic sensors, sophisticated image processing, and machine learning algorithms to deliver real-time insights and alerts to railway operators.
RV develops its technology for both rolling stock (trains) and trackside infrastructure. The company's systems are designed to operate in various environmental conditions and can be retrofitted onto existing railway networks. RV's business strategy involves collaborating with railway companies, infrastructure providers, and other industry stakeholders to deploy its solutions globally. The company aims to become a leading provider of comprehensive railway safety and efficiency solutions, helping to modernize rail transportation worldwide.
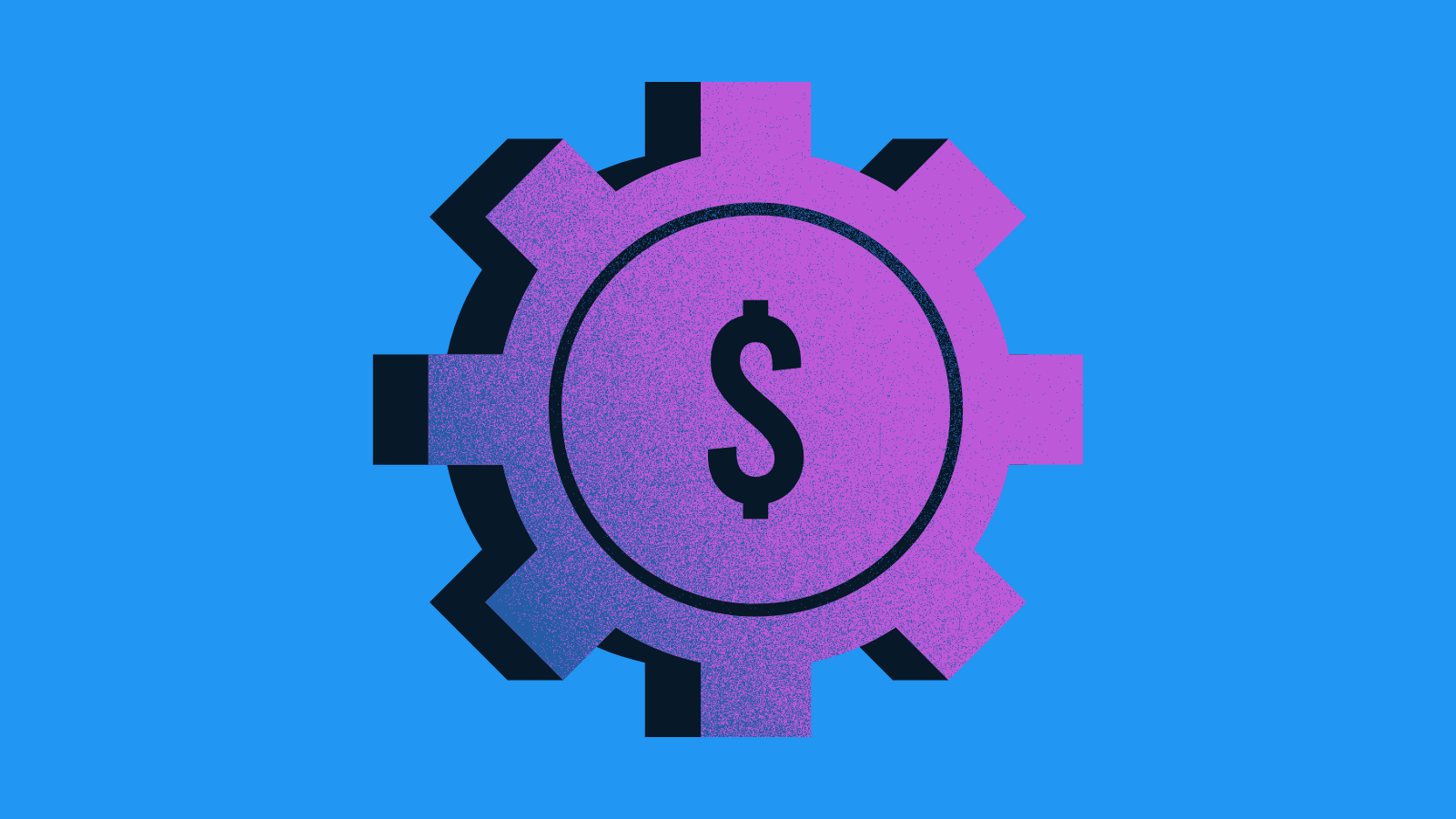
RVSN Stock Forecast Machine Learning Model
Our team proposes a comprehensive machine learning model for forecasting Rail Vision Ltd. (RVSN) stock performance. This model integrates diverse datasets to capture the multifaceted nature of the market. Input features will include technical indicators (e.g., moving averages, RSI, MACD), fundamental data (e.g., financial ratios, earnings reports), and macroeconomic indicators (e.g., inflation rates, interest rates, GDP growth). Crucially, we will incorporate alternative data sources like news sentiment analysis (using natural language processing to gauge market perception of RVSN), social media sentiment, and analyst ratings. We will also consider industry-specific factors, such as railway infrastructure investment trends and technological advancements in railway safety systems, which directly impact Rail Vision's business. The model will be trained and validated using historical data, spanning several years, to ensure robustness and generalizability.
The core of our model will leverage an ensemble approach, combining the strengths of multiple machine learning algorithms. We intend to utilize a blend of time series models (e.g., ARIMA, Prophet) and advanced machine learning techniques (e.g., Random Forest, Gradient Boosting Machines, and potentially, Neural Networks) to capture both the linear and non-linear relationships within the data. We will implement a careful hyperparameter tuning process using techniques like cross-validation to optimize each individual model and the ensemble's overall performance. Feature importance will be assessed to identify the most influential variables and refine the model. The outputs will provide predicted directional movement (e.g., increase, decrease, or no change) for the stock, alongside a confidence level representing the model's certainty in its prediction.
Our model's evaluation will focus on both statistical accuracy and practical utility. We will use standard metrics like precision, recall, F1-score, and area under the ROC curve (AUC) to assess predictive power. Furthermore, we will incorporate real-world backtesting, simulating trading strategies based on the model's forecasts to assess its ability to generate profits. We will regularly retrain the model with new data and continuously monitor its performance, adapting to market dynamics and evolving factors. The output of the model is designed to inform investment decisions. It is important to acknowledge, this model does not guarantee profit and requires risk management. We are committed to transparency and will provide regular reports detailing the model's performance and any limitations to stakeholders.
ML Model Testing
n:Time series to forecast
p:Price signals of Rail Vision stock
j:Nash equilibria (Neural Network)
k:Dominated move of Rail Vision stock holders
a:Best response for Rail Vision target price
For further technical information as per how our model work we invite you to visit the article below:
How do KappaSignal algorithms actually work?
Rail Vision Stock Forecast (Buy or Sell) Strategic Interaction Table
Strategic Interaction Table Legend:
X axis: *Likelihood% (The higher the percentage value, the more likely the event will occur.)
Y axis: *Potential Impact% (The higher the percentage value, the more likely the price will deviate.)
Z axis (Grey to Black): *Technical Analysis%
Financial Outlook and Forecast for RVL
Rail Vision Ltd. (RVL), a technology company focused on AI-powered safety systems for railway operations, presents a financial outlook shaped by its nascent market position and technological innovation. The company's revenue generation hinges on securing contracts for the installation and maintenance of its systems across various railway networks. Future revenue streams will likely be driven by recurring revenue from data analytics services, software updates, and system support, creating a more stable financial footing over time. RVL's success is directly tied to its ability to demonstrate the efficacy of its technology in improving safety and operational efficiency, which will translate into tangible cost savings and minimized risk for railway operators. Key factors influencing RVL's financial trajectory include the adoption rate of its systems, the successful execution of current and future contracts, and the competitive landscape, particularly in the rapidly evolving field of railway safety technology.
The financial forecasts for RVL are contingent upon several growth drivers. The global push for increased railway safety and infrastructure modernization provides a significant market opportunity. Securing strategic partnerships with established railway companies and rolling stock manufacturers is crucial for accelerating market penetration. The company's financial model will largely depend on the pace of its expansion efforts, its effectiveness in securing pilot projects that demonstrate the value of its technology, and its capability to manufacture and deploy its systems cost-effectively. RVL's ability to scale its operations, manage its cash flow effectively, and attract further investment will be important in determining its financial performance. The company will need to make calculated investments in research and development to maintain its technological edge and remain competitive within this quickly changing industry.
RVL's future financial success is contingent on managing expenses. The company must carefully manage its operational costs to ensure profitability. While the high initial investment costs can impact short-term profitability, the recurring revenue potential from service contracts can significantly improve its long-term profitability and cash flow. Also, there are many governmental regulations and approvals needed which can also impact the project. RVL is also required to navigate these regulations, to accelerate its project timelines and maintain a competitive advantage. Also, RVL's ability to maintain a healthy balance sheet will be critical for attracting investor confidence and supporting its expansion plans.
Based on the current dynamics, RVL is predicted to have a positive outlook. The rising interest in railway safety and the technological innovations provided by RVL position it to capitalize on a large market opportunity. However, there are risks to consider. The competitive field can be hard, as some of these established competitors will provide many similar features. Furthermore, the success is heavily dependent on the adoption rate of its technology, which can be influenced by factors such as market acceptance, economic conditions, and the regulatory framework. There is always the risk of project delays, technical challenges, or difficulties in securing future funding. Investors should carefully evaluate these risks and consider their potential impact on RVL's financial prospects.
```
Rating | Short-Term | Long-Term Senior |
---|---|---|
Outlook | B1 | B1 |
Income Statement | B1 | C |
Balance Sheet | Ba3 | B2 |
Leverage Ratios | Ba2 | C |
Cash Flow | C | Baa2 |
Rates of Return and Profitability | Ba3 | Baa2 |
*Financial analysis is the process of evaluating a company's financial performance and position by neural network. It involves reviewing the company's financial statements, including the balance sheet, income statement, and cash flow statement, as well as other financial reports and documents.
How does neural network examine financial reports and understand financial state of the company?
References
- Breiman L. 1996. Bagging predictors. Mach. Learn. 24:123–40
- Dietterich TG. 2000. Ensemble methods in machine learning. In Multiple Classifier Systems: First International Workshop, Cagliari, Italy, June 21–23, pp. 1–15. Berlin: Springer
- Bottou L. 2012. Stochastic gradient descent tricks. In Neural Networks: Tricks of the Trade, ed. G Montavon, G Orr, K-R Müller, pp. 421–36. Berlin: Springer
- Dimakopoulou M, Zhou Z, Athey S, Imbens G. 2018. Balanced linear contextual bandits. arXiv:1812.06227 [cs.LG]
- Wu X, Kumar V, Quinlan JR, Ghosh J, Yang Q, et al. 2008. Top 10 algorithms in data mining. Knowl. Inform. Syst. 14:1–37
- M. Sobel. The variance of discounted Markov decision processes. Applied Probability, pages 794–802, 1982
- Alexander, J. C. Jr. (1995), "Refining the degree of earnings surprise: A comparison of statistical and analysts' forecasts," Financial Review, 30, 469–506.