AUC Score :
Short-term Tactic1 :
Dominant Strategy :
Time series to forecast n:
ML Model Testing : Modular Neural Network (CNN Layer)
Hypothesis Testing : Polynomial Regression
Surveillance : Major exchange and OTC
1Short-term revised.
2Time series is updated based on short-term trends.
Key Points
PolyPid's trajectory is expected to be highly volatile. The company's success hinges on the regulatory approval and commercialization of its products, particularly D-PLEX100. Strong clinical trial data would likely fuel positive market sentiment and drive share price appreciation, while failure to obtain regulatory clearances or disappointing sales figures could lead to significant price declines. Additional risks include competition in the wound care and surgical site infection prevention markets, potential challenges in manufacturing, and the need for continuous funding. Furthermore, any delays in clinical trials or adverse reactions during use could critically impair the company's prospects.About PolyPid Ltd.
PolyPid Ltd. is a clinical-stage biopharmaceutical company focused on developing innovative therapies for unmet medical needs. It specializes in localized drug delivery technologies, aiming to improve treatment efficacy and reduce side effects associated with various medical conditions. The company's proprietary technology platform allows for the controlled release of therapeutic agents directly at the site of action, potentially minimizing systemic exposure.
PolyPid's product pipeline includes a range of candidates targeting areas such as surgical site infections, bone infections, and wound healing. The company conducts clinical trials to evaluate the safety and efficacy of its products, seeking regulatory approvals for commercialization. PolyPid collaborates with healthcare professionals and institutions to advance its research and development efforts. The company's primary goal is to provide better treatment options and improve patient outcomes.
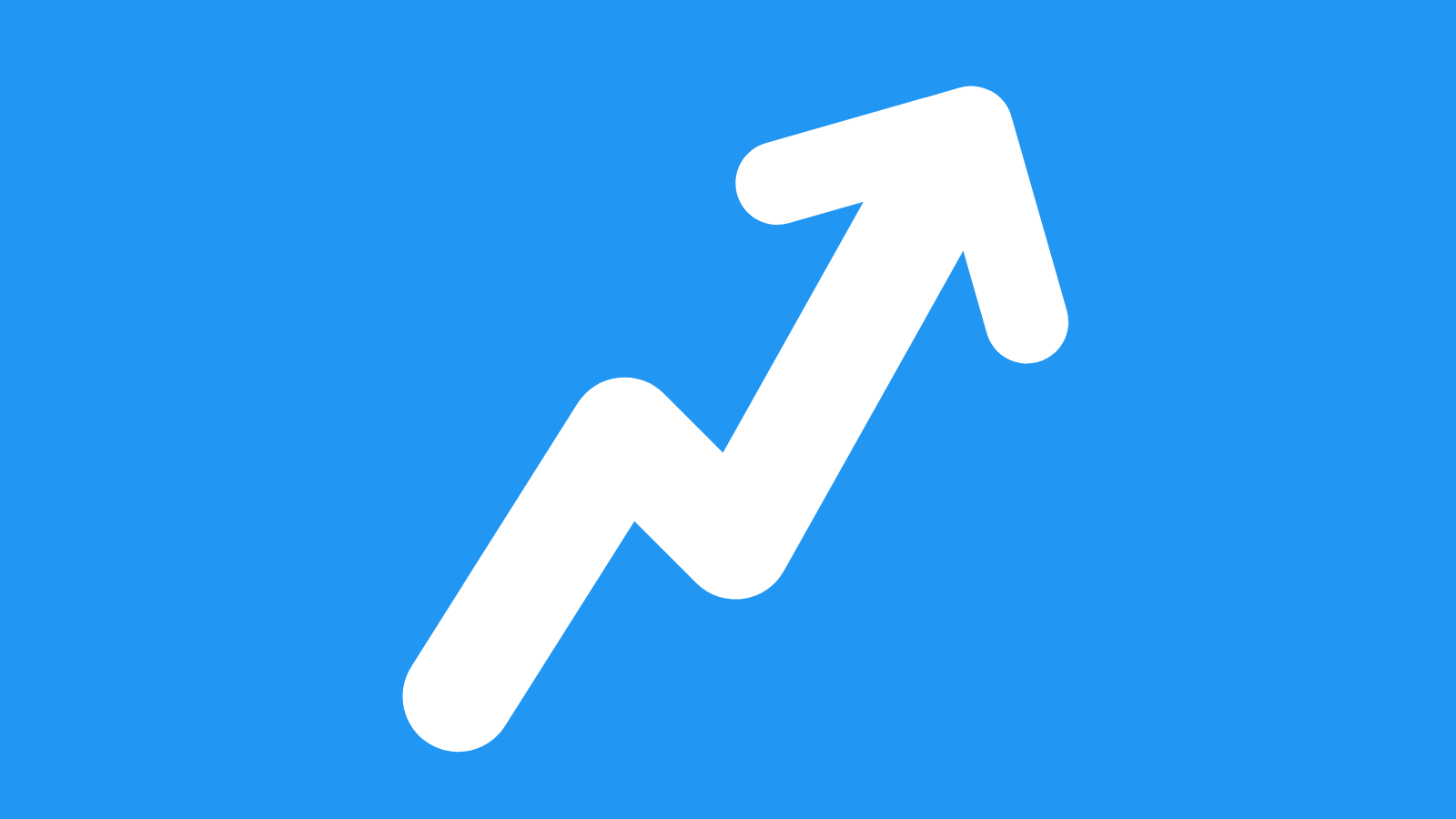
PYPD Stock Forecast Model
Our data science and economics team has developed a machine learning model to forecast the performance of PolyPid Ltd. Ordinary Shares (PYPD). This model utilizes a comprehensive set of features encompassing both internal and external factors. Internal data includes the company's financial statements (revenue, expenses, profitability ratios), management team changes, clinical trial outcomes, and product pipeline advancements. We also incorporate technical indicators such as moving averages, Relative Strength Index (RSI), and trading volume patterns. External data sources comprise macroeconomic indicators like inflation rates, interest rates, and GDP growth, industry-specific information such as competitor performance and market trends, and news sentiment analysis based on press releases and social media chatter concerning PolyPid and the broader pharmaceutical sector. The model is trained on historical data, regularly updated with the latest information to ensure accuracy and relevance.
The core of our model employs a gradient boosting algorithm, specifically XGBoost, known for its high predictive accuracy and ability to handle complex, non-linear relationships within the data. We've experimented with various algorithms, including Recurrent Neural Networks (RNNs) and Support Vector Machines (SVMs), but XGBoost consistently yielded the best results when evaluated using common metrics such as Mean Squared Error (MSE) and Root Mean Squared Error (RMSE). Feature importance analysis is conducted to identify and prioritize the most influential variables driving the stock's fluctuations. This allows us to focus our attention on the most critical aspects affecting PYPD's valuation and provides valuable insights for strategic decision-making. To mitigate overfitting and ensure model robustness, we implement cross-validation techniques, including k-fold cross-validation, and regularize the model's parameters.
Our forecasting output provides a probabilistic prediction of PYPD's future movements over various time horizons. We generate a range of potential outcomes based on the model's confidence levels. Importantly, our model is not a "black box." We continuously monitor and evaluate its performance, making necessary adjustments to the feature set, algorithm parameters, and model structure as new data emerges and market dynamics evolve. Regular model validation and backtesting against historical data are integral parts of our ongoing process. Our team recognizes the inherent uncertainty in stock market forecasting and incorporates risk assessments in our reports. The goal is to provide actionable insights to assist informed investment strategies.
ML Model Testing
n:Time series to forecast
p:Price signals of PolyPid Ltd. stock
j:Nash equilibria (Neural Network)
k:Dominated move of PolyPid Ltd. stock holders
a:Best response for PolyPid Ltd. target price
For further technical information as per how our model work we invite you to visit the article below:
How do KappaSignal algorithms actually work?
PolyPid Ltd. Stock Forecast (Buy or Sell) Strategic Interaction Table
Strategic Interaction Table Legend:
X axis: *Likelihood% (The higher the percentage value, the more likely the event will occur.)
Y axis: *Potential Impact% (The higher the percentage value, the more likely the price will deviate.)
Z axis (Grey to Black): *Technical Analysis%
PolyPid Ltd. Financial Outlook and Forecast
PolyPid's (PYPD) financial outlook is intricately tied to the commercial success of its lead product, D-PLEX100, and its clinical pipeline. D-PLEX100 is an extended-release drug delivery technology designed to provide sustained release of antibiotics directly at the surgical site, aiming to reduce post-surgical infections. Currently, PYPD is focusing on obtaining regulatory approvals and subsequent market penetration, primarily within the United States and Europe. The company's revenue generation will hinge on the timely and successful launch of D-PLEX100 in these key markets. The effectiveness of their marketing efforts, alongside securing favorable reimbursement agreements from healthcare providers and insurance companies, will significantly influence the initial revenue trajectory. Additionally, the progress of clinical trials for D-PLEX100 in new indications and the advancement of other pipeline candidates, such as the development of products for wound care and bone regeneration, will be crucial for long-term growth and diversification of revenue streams.
The forecast for PYPD's financials projects a period of significant investment and operational expenses in the immediate future. These expenses will be primarily directed towards sales and marketing activities, clinical trials, manufacturing scale-up, and regulatory compliance. It is anticipated that the company will incur substantial operating losses as it invests in these growth initiatives, especially until D-PLEX100 achieves robust commercial adoption. Analysts generally expect revenue to ramp up as the product gains market share, yet reaching profitability is expected to be a few years away. Careful management of cash flow, especially through strategic partnerships, collaborations, or potentially, further equity financing, will be essential to sustain operations and fund ongoing research and development efforts. Moreover, the financial forecast needs to consider potential fluctuations in foreign exchange rates, given the company's global operations and potential for international revenues.
Key drivers of PYPD's financial performance will be the regulatory approvals for D-PLEX100 in additional indications. Success of clinical trials and timely market access for these expansions will offer potential upside for the company. Furthermore, the rate of market penetration and the competitive landscape within the surgical site infection prevention market will greatly influence revenue growth. Positive data from ongoing or future clinical trials, leading to potential expansion of D-PLEX100's label and indications, could have a strong impact on the company's financial valuation. Partnerships with established pharmaceutical companies or strategic acquisitions would also provide further financial flexibility and access to expertise and distribution channels, which could positively impact the company's outlook.
Considering the factors above, a positive financial outlook is predicted for PYPD, contingent on D-PLEX100 achieving significant commercial success and the timely launch of new indications. This prediction is accompanied by several risks. These include the potential for delays in regulatory approvals, adverse clinical trial results, competitive pressures from other companies with similar or competing products, and the inherent risks associated with drug development, such as manufacturing issues and the possibility of litigation. Additionally, the company's ability to secure future funding, either through debt or equity financing, is crucial. The ability to manage these risks effectively will determine the long-term financial health and success of PYPD.
Rating | Short-Term | Long-Term Senior |
---|---|---|
Outlook | Ba1 | B1 |
Income Statement | Ba3 | B2 |
Balance Sheet | Baa2 | Baa2 |
Leverage Ratios | C | B3 |
Cash Flow | Baa2 | Caa2 |
Rates of Return and Profitability | Baa2 | B3 |
*Financial analysis is the process of evaluating a company's financial performance and position by neural network. It involves reviewing the company's financial statements, including the balance sheet, income statement, and cash flow statement, as well as other financial reports and documents.
How does neural network examine financial reports and understand financial state of the company?
References
- Clements, M. P. D. F. Hendry (1995), "Forecasting in cointegrated systems," Journal of Applied Econometrics, 10, 127–146.
- Bottomley, P. R. Fildes (1998), "The role of prices in models of innovation diffusion," Journal of Forecasting, 17, 539–555.
- S. Devlin, L. Yliniemi, D. Kudenko, and K. Tumer. Potential-based difference rewards for multiagent reinforcement learning. In Proceedings of the Thirteenth International Joint Conference on Autonomous Agents and Multiagent Systems, May 2014
- Imai K, Ratkovic M. 2013. Estimating treatment effect heterogeneity in randomized program evaluation. Ann. Appl. Stat. 7:443–70
- M. Ono, M. Pavone, Y. Kuwata, and J. Balaram. Chance-constrained dynamic programming with application to risk-aware robotic space exploration. Autonomous Robots, 39(4):555–571, 2015
- Breiman L. 1996. Bagging predictors. Mach. Learn. 24:123–40
- Farrell MH, Liang T, Misra S. 2018. Deep neural networks for estimation and inference: application to causal effects and other semiparametric estimands. arXiv:1809.09953 [econ.EM]