AUC Score :
Short-term Tactic1 :
Dominant Strategy :
Time series to forecast n:
ML Model Testing : Modular Neural Network (Market News Sentiment Analysis)
Hypothesis Testing : Multiple Regression
Surveillance : Major exchange and OTC
1Short-term revised.
2Time series is updated based on short-term trends.
Key Points
LRCX faces a mixed outlook. Positive predictions include continued demand for advanced semiconductor manufacturing equipment, benefiting from the ongoing expansion of chip fabrication capacity globally, particularly in areas like high-performance computing and artificial intelligence. LRCX is also anticipated to leverage its strong market position and technological advancements to drive revenue growth and maintain profitability. However, the company is subject to risks. A cyclical downturn in the semiconductor industry could significantly impact its financial performance, as demand for its equipment is highly correlated with chipmakers' investments. Geopolitical tensions, especially trade restrictions and supply chain disruptions, pose significant risks to LRCX's operations and ability to deliver its products. Intense competition within the semiconductor equipment market and the potential for slower-than-expected adoption of new technologies are other factors that could hinder the company's growth.About Lam Research
Lam Research (LRCX) is a global supplier of innovative wafer fabrication equipment and services to the semiconductor industry. The company designs, manufactures, and services advanced wafer fabrication equipment used in the production of integrated circuits. LRCX's products are critical for various chip manufacturing processes, including etching, deposition, and cleaning, supporting the fabrication of leading-edge semiconductors used in a wide array of electronic devices.
The company serves a diverse customer base, including semiconductor manufacturers, foundries, and memory producers worldwide. Its technologies are essential for creating increasingly complex and powerful microchips, driving the demand for advanced semiconductor equipment. LRCX's ongoing investments in research and development allow it to stay at the forefront of technological advancements in the semiconductor industry, maintaining its competitive position.
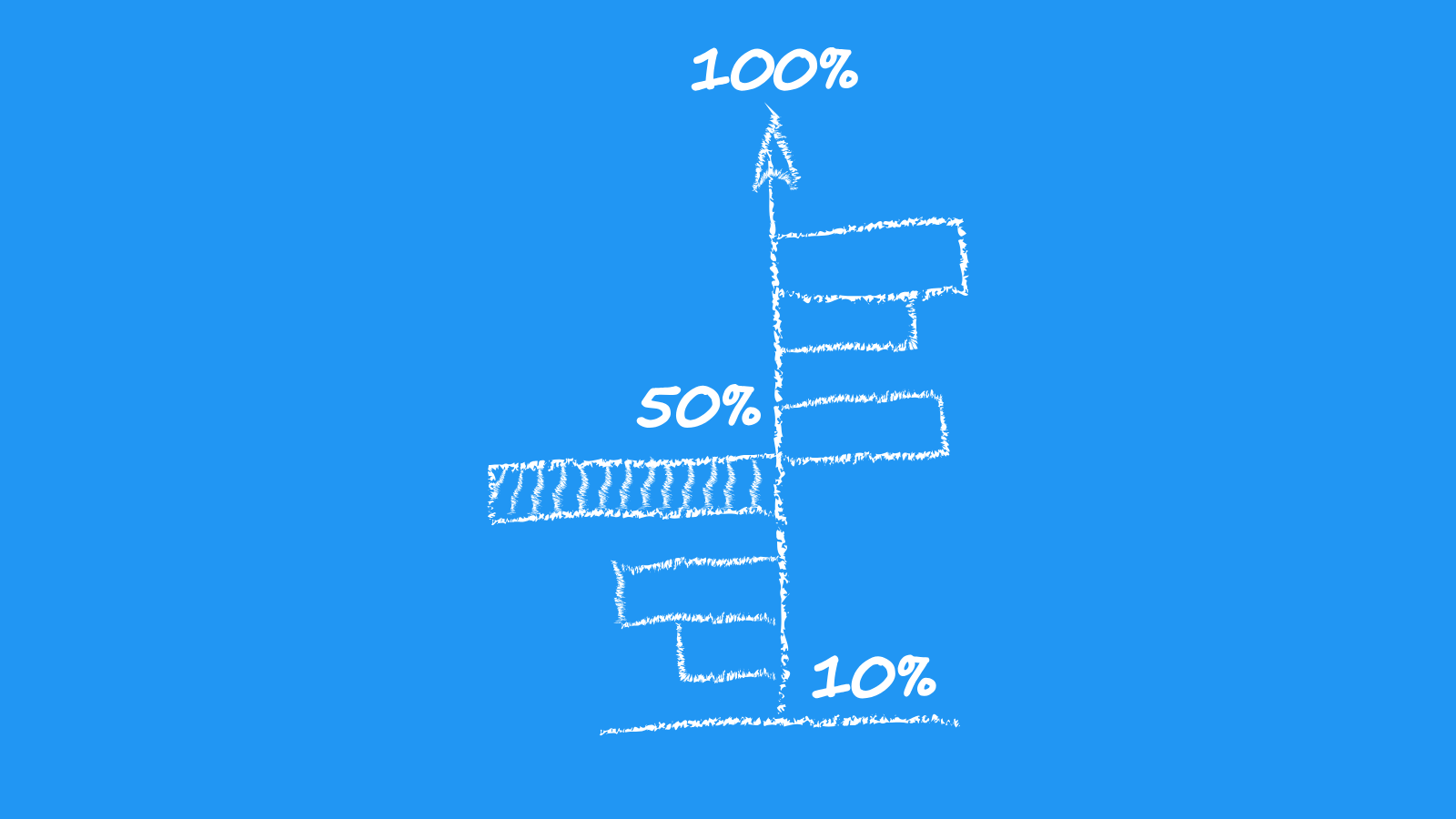
LRCX Stock Forecast Machine Learning Model
Our approach to forecasting Lam Research Corporation (LRCX) stock performance centers on developing a robust machine learning model capable of capturing complex relationships between various financial and economic indicators. The core of our model will be built upon a hybrid architecture, combining the strengths of recurrent neural networks (RNNs), specifically Long Short-Term Memory (LSTM) networks, with gradient boosting methods, such as XGBoost or LightGBM. The LSTM components will excel at processing sequential data, such as historical stock movements, trading volume, and volatility, effectively learning temporal dependencies. Concurrently, gradient boosting techniques will be integrated to analyze a diverse set of external factors.
The feature engineering process is crucial to the model's performance. We will incorporate a comprehensive set of features, including financial ratios derived from LRCX's quarterly and annual reports (e.g., price-to-earnings ratio, debt-to-equity ratio, gross margin), macroeconomic indicators (e.g., GDP growth, inflation rates, interest rates, semiconductor industry sales data), and sentiment analysis based on news articles and social media mentions related to LRCX and the semiconductor industry. Moreover, we will consider technical indicators such as moving averages, Relative Strength Index (RSI), and the Moving Average Convergence Divergence (MACD) to capture market trends and momentum. Model training will involve splitting the historical data into training, validation, and testing sets. We will apply cross-validation techniques to ensure the model's generalizability and minimize overfitting.
The model's evaluation will prioritize several key metrics: Mean Squared Error (MSE) and Root Mean Squared Error (RMSE) to assess the accuracy of the forecasted values, and the R-squared coefficient to evaluate the goodness of fit. Furthermore, we will employ a backtesting strategy using historical data to assess the model's performance in a simulated trading environment. This strategy will allow us to evaluate the model's ability to generate profitable trading signals by simulating buying and selling decisions based on the model's predictions. We will also incorporate risk management protocols to mitigate potential losses. Finally, the model will be continuously monitored and retrained using the latest available data to maintain its accuracy and adapt to changing market conditions. The final output will be a probabilistic forecast, presenting the likelihood of future price movements, enabling informed investment decisions.
ML Model Testing
n:Time series to forecast
p:Price signals of Lam Research stock
j:Nash equilibria (Neural Network)
k:Dominated move of Lam Research stock holders
a:Best response for Lam Research target price
For further technical information as per how our model work we invite you to visit the article below:
How do KappaSignal algorithms actually work?
Lam Research Stock Forecast (Buy or Sell) Strategic Interaction Table
Strategic Interaction Table Legend:
X axis: *Likelihood% (The higher the percentage value, the more likely the event will occur.)
Y axis: *Potential Impact% (The higher the percentage value, the more likely the price will deviate.)
Z axis (Grey to Black): *Technical Analysis%
Lam Research Corporation Financial Outlook and Forecast
The financial outlook for LRCX remains robust, driven by the ongoing demand for advanced semiconductor manufacturing equipment. The company is well-positioned to capitalize on the expanding global semiconductor market, fueled by trends like artificial intelligence, data centers, and the Internet of Things. LRCX's strong position in crucial segments such as deposition, etching, and cleaning technologies allows it to maintain its leading edge, supporting the production of smaller, faster, and more energy-efficient chips. Furthermore, LRCX's ongoing research and development initiatives, particularly in areas like EUV lithography support, signal a dedication to sustaining its technological prowess. The company's diversified customer base and global presence further provide stability against regional economic fluctuations, allowing for consistent revenue generation and earnings growth. Its focus on providing not only equipment but also services and support creates a recurring revenue stream, enhancing financial predictability.
Forecasts indicate a continued upward trajectory for LRCX's financial performance. Analysts anticipate sustained revenue growth, particularly as the demand for advanced nodes grows. LRCX's strong order backlog provides increased visibility into future revenues and supports confidence in its near-term financial results. Gross margins are expected to remain healthy, bolstered by product mix, pricing strategies, and cost control measures. Investments in its service business are also set to generate increased revenue and improved profitability. The strategic allocation of capital, including share repurchases, indicates management's commitment to shareholder value and reinforces the positive outlook. These financial projections are generally positive, with analysts projecting continued earnings per share growth as the company remains a critical supplier of advanced semiconductor equipment.
The company's management has demonstrated a solid track record of effectively navigating cyclical semiconductor markets. LRCX's commitment to innovation and new product introductions is also projected to translate into market share gains and enhanced revenue streams. This allows the company to support its long-term growth objectives and maintain its competitive advantage. LRCX's consistent revenue growth and robust free cash flow generation enable the company to allocate resources to R&D, debt reduction, and share buybacks. LRCX's strong capital allocation strategy, including stock buybacks and dividend distributions, supports its valuation and strengthens its investment case. With a well-structured financial model and an effective management team, LRCX is very likely to outperform the market.
In conclusion, the financial outlook for LRCX is positive. The company is likely to benefit from strong secular trends driving the semiconductor industry's growth. Its strong technology portfolio, healthy order backlog, and capital allocation strategies make it a compelling investment. However, there are associated risks. The semiconductor industry is cyclical, and any downturn in end-market demand could impact revenue. Geopolitical tensions and supply chain disruptions may also present risks to the company's operations and financial performance. Lastly, strong competition from rival suppliers might compress margins. Despite these risks, LRCX's positioning within the industry makes it well-placed for future growth.
Rating | Short-Term | Long-Term Senior |
---|---|---|
Outlook | B1 | Ba3 |
Income Statement | Baa2 | B3 |
Balance Sheet | B3 | Baa2 |
Leverage Ratios | Ba3 | Caa2 |
Cash Flow | C | C |
Rates of Return and Profitability | Baa2 | Baa2 |
*Financial analysis is the process of evaluating a company's financial performance and position by neural network. It involves reviewing the company's financial statements, including the balance sheet, income statement, and cash flow statement, as well as other financial reports and documents.
How does neural network examine financial reports and understand financial state of the company?
References
- L. Panait and S. Luke. Cooperative multi-agent learning: The state of the art. Autonomous Agents and Multi-Agent Systems, 11(3):387–434, 2005.
- P. Marbach. Simulated-Based Methods for Markov Decision Processes. PhD thesis, Massachusetts Institute of Technology, 1998
- S. Bhatnagar, R. Sutton, M. Ghavamzadeh, and M. Lee. Natural actor-critic algorithms. Automatica, 45(11): 2471–2482, 2009
- S. Bhatnagar. An actor-critic algorithm with function approximation for discounted cost constrained Markov decision processes. Systems & Control Letters, 59(12):760–766, 2010
- Candès E, Tao T. 2007. The Dantzig selector: statistical estimation when p is much larger than n. Ann. Stat. 35:2313–51
- Breiman L, Friedman J, Stone CJ, Olshen RA. 1984. Classification and Regression Trees. Boca Raton, FL: CRC Press
- Y. Chow and M. Ghavamzadeh. Algorithms for CVaR optimization in MDPs. In Advances in Neural Infor- mation Processing Systems, pages 3509–3517, 2014.