AUC Score :
Short-term Tactic1 :
Dominant Strategy :
Time series to forecast n:
ML Model Testing : Modular Neural Network (CNN Layer)
Hypothesis Testing : ElasticNet Regression
Surveillance : Major exchange and OTC
1Short-term revised.
2Time series is updated based on short-term trends.
Key Points
Inter's stock could experience moderate growth due to its increasing user base and expansion of financial services in Brazil, driven by its innovative platform and strong brand recognition; however, a potential risk lies in heightened competition from established banks and fintech rivals, potentially impacting profitability and market share. The company's expansion plans could be hampered by regulatory changes and macroeconomic fluctuations in the Brazilian economy, which could lead to unpredictable performance. Furthermore, maintaining high customer satisfaction amid rapid growth is crucial, and any failures in this regard could impact future performance and investor confidence.About Inter & Co.
Inter & Co., Inc. (ITAU) is a prominent Brazilian digital banking platform and technology company. It operates primarily in the financial services sector, providing a range of digital banking solutions to individuals and businesses. These services include checking and savings accounts, credit and debit cards, investment platforms, insurance products, and a marketplace for various services. ITAU aims to provide a comprehensive digital ecosystem that streamlines financial management and simplifies access to financial products.
The company distinguishes itself by offering a user-friendly platform and prioritizing technological innovation. ITAU focuses on expanding its customer base and expanding its offerings. It also places an emphasis on providing tailored financial solutions and leveraging technology to optimize user experience. The company operates within a competitive landscape, it contends with both traditional financial institutions and other digital banking startups in the Brazilian market.
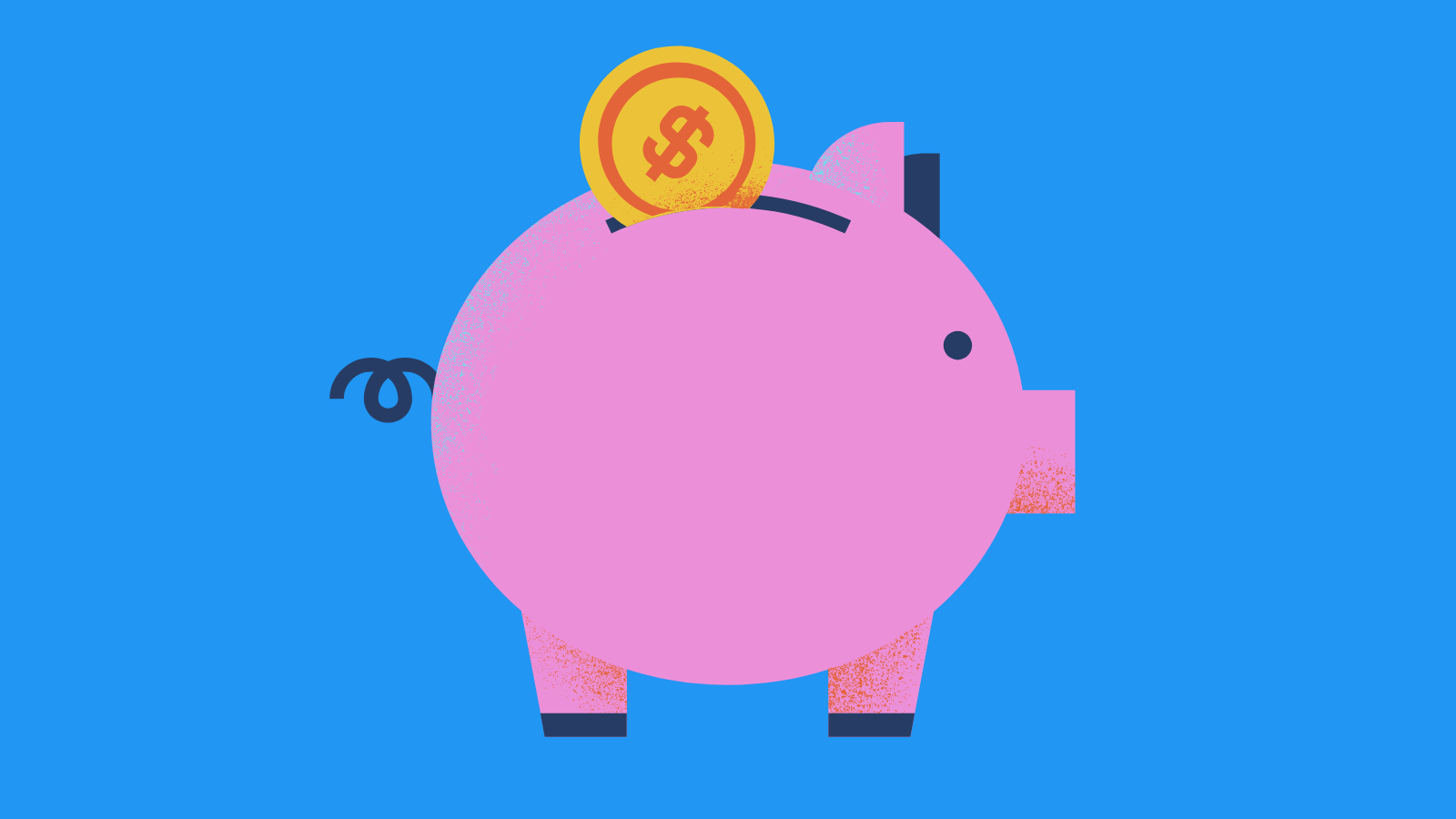
INTR Stock Forecast Model
Our team, composed of data scientists and economists, has developed a machine learning model to forecast the performance of Inter & Co. Inc. Class A Common Shares (INTR). The core of our model utilizes a time series analysis approach, incorporating a variety of relevant features. These features are categorized into financial indicators, market sentiment, and macroeconomic factors. Financial indicators include revenue growth, earnings per share (EPS), debt-to-equity ratio, and operating margins. Market sentiment is gauged by monitoring social media activity, news sentiment analysis related to INTR and the financial sector, and investor confidence indices. Macroeconomic factors encompass interest rates, inflation rates, GDP growth, and exchange rates relevant to the company's operating regions. Data preprocessing is crucial; we handle missing values, perform feature scaling to normalize data ranges, and address potential outliers. We employ a rolling window approach for training and validation, ensuring the model adapts to evolving market conditions.
For model selection, we evaluated several machine learning algorithms, including Recurrent Neural Networks (RNNs), specifically Long Short-Term Memory (LSTM) networks, known for their proficiency in capturing temporal dependencies in time series data. We also considered Support Vector Machines (SVMs) and Gradient Boosting algorithms, analyzing their performance based on established metrics like Mean Squared Error (MSE) and Mean Absolute Percentage Error (MAPE). Hyperparameter tuning is performed using techniques like grid search and cross-validation, optimizing the model's predictive accuracy. This iterative process allows us to identify the optimal configuration for the chosen algorithms, tailored to the unique characteristics of INTR's stock behavior. The model will incorporate a combination of features to enhance predictive performance.
The final model will provide forecasts, and its outputs will be interpretable, offering insights into the factors driving predicted performance. The model's success will be measured by backtesting it against historical data and regularly monitoring its performance using real-time market data. The model will be updated on a regular basis to account for the dynamic nature of the financial markets and new data availability. We are also developing a risk management framework to incorporate model uncertainties into investment decisions, ensuring a cautious and robust approach. This framework will serve as a valuable tool for understanding the likely behavior of INTR stock, and it must be used with other investment strategies to inform the investor of the stock. The model is continuously reviewed and enhanced as new data becomes available.
ML Model Testing
n:Time series to forecast
p:Price signals of Inter & Co. stock
j:Nash equilibria (Neural Network)
k:Dominated move of Inter & Co. stock holders
a:Best response for Inter & Co. target price
For further technical information as per how our model work we invite you to visit the article below:
How do KappaSignal algorithms actually work?
Inter & Co. Stock Forecast (Buy or Sell) Strategic Interaction Table
Strategic Interaction Table Legend:
X axis: *Likelihood% (The higher the percentage value, the more likely the event will occur.)
Y axis: *Potential Impact% (The higher the percentage value, the more likely the price will deviate.)
Z axis (Grey to Black): *Technical Analysis%
Inter & Co. Inc. Class A Common Shares: Financial Outlook and Forecast
The financial outlook for Inter & Co. (INTR) Class A Common Shares presents a mixed picture, contingent on several factors. The company, a digital banking platform, has demonstrated strong growth in its user base and transaction volume, particularly within the burgeoning Brazilian financial market. This growth is primarily driven by its attractive product offerings, including commission-free services, competitive interest rates, and a user-friendly digital interface. These advantages position INTR favorably to capture a significant share of the rapidly digitizing financial landscape in Brazil. Furthermore, the company's expansion into other areas, such as insurance and credit, offers additional avenues for revenue diversification and sustained growth. The focus on customer acquisition and retention is a key indicator of the company's ambition and potential. Continued operational efficiency and scalability will be critical for maintaining profitability as the company expands its operations.
The forecast for INTR is significantly influenced by macroeconomic trends within Brazil. The overall economic health of the country, including inflation rates, interest rate movements, and consumer spending, will directly impact the company's financial performance. A period of sustained economic growth, coupled with controlled inflation, would likely fuel further expansion, increasing transaction volumes and demand for its products. Government policies and regulatory frameworks related to digital banking and financial technology (FinTech) also play a vital role. Supportive regulations could accelerate the adoption of digital banking, benefiting INTR and its peers. Conversely, any economic downturn or unfavorable regulatory shifts could pose challenges to its growth trajectory. It is also necessary to consider the competitive landscape. Established financial institutions and other FinTech companies are actively competing for market share, intensifying competition.
INTR's capacity to execute its growth strategy effectively will be crucial for its future financial performance. The company has been actively investing in its technology infrastructure, marketing efforts, and human capital to support its expansion plans. The successful integration of these investments and the effective management of operational costs will determine the profitability of future expansions. The company's ability to maintain a strong capital position and access to funding will also be important for supporting its strategic initiatives. Careful management of credit risk is crucial for maintaining the quality of its loan portfolio, especially if economic conditions deteriorate. The company's success relies on a strong focus on the customer experience. Furthermore, the diversification of its product offerings will be key to driving revenue growth and expanding the platform's value proposition.
Considering these factors, the outlook for INTR is cautiously optimistic. The company's strong market position, coupled with the favorable growth prospects of the Brazilian digital banking market, indicates a positive trajectory. It is predicted that INTR will experience continued expansion in the coming years, driven by user acquisition, increased transaction volume, and product diversification. However, this positive forecast is not without risk. Economic volatility in Brazil, increased competition, and regulatory changes could significantly impact the company's performance. Furthermore, the ability to manage credit risk effectively and integrate new technologies remains essential. The successful execution of INTR's strategy, and its resilience in navigating potential challenges, will ultimately determine the long-term financial outcome.
Rating | Short-Term | Long-Term Senior |
---|---|---|
Outlook | Ba3 | Ba3 |
Income Statement | B3 | C |
Balance Sheet | Baa2 | Baa2 |
Leverage Ratios | Caa2 | C |
Cash Flow | Baa2 | Baa2 |
Rates of Return and Profitability | B2 | Baa2 |
*Financial analysis is the process of evaluating a company's financial performance and position by neural network. It involves reviewing the company's financial statements, including the balance sheet, income statement, and cash flow statement, as well as other financial reports and documents.
How does neural network examine financial reports and understand financial state of the company?
References
- Athey S, Imbens GW. 2017a. The econometrics of randomized experiments. In Handbook of Economic Field Experiments, Vol. 1, ed. E Duflo, A Banerjee, pp. 73–140. Amsterdam: Elsevier
- Mazumder R, Hastie T, Tibshirani R. 2010. Spectral regularization algorithms for learning large incomplete matrices. J. Mach. Learn. Res. 11:2287–322
- Lai TL, Robbins H. 1985. Asymptotically efficient adaptive allocation rules. Adv. Appl. Math. 6:4–22
- Hirano K, Porter JR. 2009. Asymptotics for statistical treatment rules. Econometrica 77:1683–701
- Akgiray, V. (1989), "Conditional heteroscedasticity in time series of stock returns: Evidence and forecasts," Journal of Business, 62, 55–80.
- Bessler, D. A. S. W. Fuller (1993), "Cointegration between U.S. wheat markets," Journal of Regional Science, 33, 481–501.
- Athey S, Blei D, Donnelly R, Ruiz F. 2017b. Counterfactual inference for consumer choice across many prod- uct categories. AEA Pap. Proc. 108:64–67