AUC Score :
Short-term Tactic1 :
Dominant Strategy :
Time series to forecast n:
ML Model Testing : Modular Neural Network (Market News Sentiment Analysis)
Hypothesis Testing : Beta
Surveillance : Major exchange and OTC
1Short-term revised.
2Time series is updated based on short-term trends.
Key Points
Inotiv's future performance is anticipated to face considerable uncertainty. The company could experience volatility stemming from potential shifts in preclinical and clinical research, impacting contract revenue. Positive predictions include possible gains related to successful drug development programs with the company's clients and increased demand within the pharmaceutical sector. However, significant risks involve regulatory scrutiny, potential litigation, and the inherent unpredictability of drug development timelines, any of which could negatively affect Inotiv's profitability and investor confidence.About Inotiv: Inotiv
Inotiv, Inc. is a contract research organization (CRO) specializing in drug discovery and development services. The company provides a range of preclinical and clinical services to pharmaceutical and biotechnology companies, focusing on areas such as safety pharmacology, toxicology, and analytical chemistry. INVO's operations support the entire drug development process, from initial research and development to regulatory submissions. They offer expertise in various therapeutic areas, including oncology, cardiovascular diseases, and metabolic disorders.
INVO's business model is centered on providing outsourced research services to clients. Its global presence includes laboratories and facilities in North America and Europe. The company aims to assist clients in accelerating their drug development timelines and ensuring the safety and efficacy of new drug candidates. INVO's services are critical in advancing pharmaceutical research, by providing essential data and support needed for the development of new medicines and therapies.
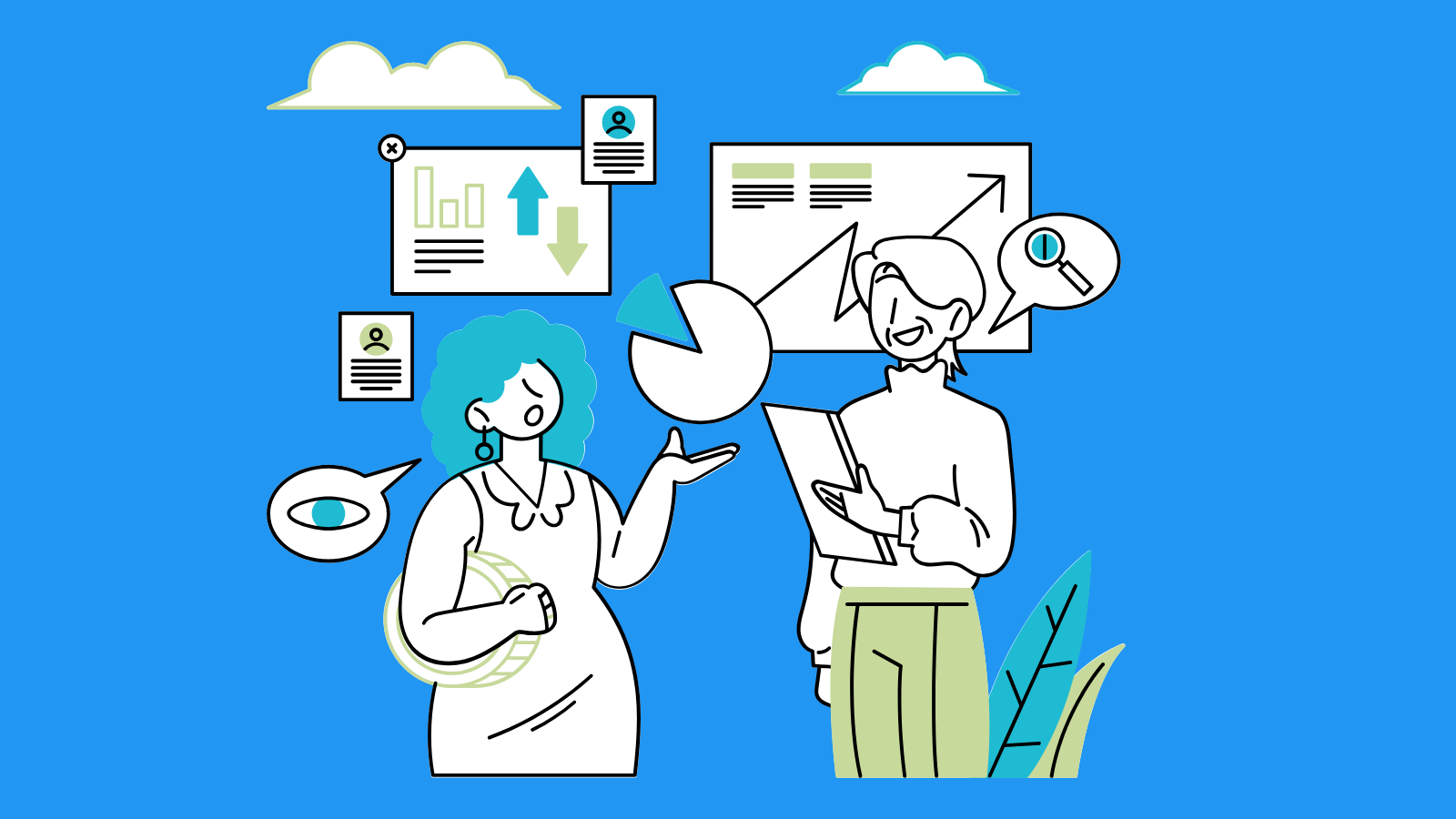
NOTV Stock Prediction Model
As data scientists and economists, we propose a comprehensive machine learning model to forecast the performance of Inotiv Inc. (NOTV) common stock. Our approach centers on a multivariate time series analysis, integrating a diverse range of predictors. Fundamental indicators will be critical inputs, encompassing quarterly and annual financial reports such as revenue, earnings per share (EPS), debt-to-equity ratio, and profit margins. Macroeconomic factors, including inflation rates, interest rates, and GDP growth, will also be incorporated to capture the broader economic environment influencing NOTV's performance. These fundamental and economic variables will establish a solid base to understand the company's long-term value. We will also incorporate market sentiment using data like analyst ratings, news sentiment scores derived from financial news sources, and social media activity to measure investor sentiment which could signal future shifts in demand for the company's stock.
The core of our model will involve the implementation of an ensemble methodology to improve prediction accuracy and robustness. We will utilize a combination of algorithms to mitigate the limitations of any single model. These will include but are not limited to Recurrent Neural Networks (RNNs) with Long Short-Term Memory (LSTM) units to recognize the inherent temporal dependencies in time-series data. Gradient Boosting Machines (GBMs) will capture complex, non-linear relationships between predictor variables and the stock's future performance. Additionally, we will employ a Support Vector Machine (SVM) as a supplementary method to offer a more unique perspective of data. Each model's predictions will be weighted and aggregated to produce the final forecast. Crucially, extensive backtesting and validation will be employed, using techniques such as cross-validation and walk-forward optimization to evaluate the model's performance and prevent overfitting.
Model outputs will consist of a probability distribution of potential stock price movements, providing buy/sell/hold recommendations with an estimated confidence level. The model's forecasts will be periodically recalibrated using up-to-date data and the latest market insights to ensure its accuracy and reliability. Regular model performance evaluations, including the calculation of accuracy metrics like Mean Absolute Error (MAE), Root Mean Squared Error (RMSE), and Sharpe ratios, will be undertaken to proactively identify the model's potential deficiencies. The model's interpretation, and recommendations, will be carefully considered in the context of prevailing market dynamics, always emphasizing a cautious approach with an understanding of market risk.
```
ML Model Testing
n:Time series to forecast
p:Price signals of Inotiv: Inotiv stock
j:Nash equilibria (Neural Network)
k:Dominated move of Inotiv: Inotiv stock holders
a:Best response for Inotiv: Inotiv target price
For further technical information as per how our model work we invite you to visit the article below:
How do KappaSignal algorithms actually work?
Inotiv: Inotiv Stock Forecast (Buy or Sell) Strategic Interaction Table
Strategic Interaction Table Legend:
X axis: *Likelihood% (The higher the percentage value, the more likely the event will occur.)
Y axis: *Potential Impact% (The higher the percentage value, the more likely the price will deviate.)
Z axis (Grey to Black): *Technical Analysis%
Inotiv Inc. (NOTV) Financial Outlook and Forecast
The financial outlook for Inotiv presents a complex picture, characterized by significant growth potential intertwined with inherent challenges. The company, focused on drug discovery and development services, benefits from the increasing demand for preclinical research and testing. This trend is primarily driven by the burgeoning pharmaceutical and biotechnology industries, which require robust data to support their drug pipelines.
NOTV's strategic acquisitions, aimed at expanding its service offerings and geographical reach, are expected to contribute positively to its revenue stream. Specifically, the integration of these acquired entities should lead to a broader service portfolio and enhanced capabilities, attracting a wider client base. Furthermore, the company's emphasis on providing integrated solutions, encompassing various stages of the drug development process, is poised to differentiate it from competitors and capture a larger market share. The continued investment in advanced technologies and research infrastructure would also assist to strengthen its competitive edge.
Regarding financial performance, a trajectory of revenue growth is anticipated. This optimistic forecast is based on the expected increase in demand for NOTV's services and successful integration of the acquired businesses. However, profitability remains a key area of focus. The company's operating margins are sensitive to fluctuations in research project timelines, contract wins, and the efficiency of its operational activities. The need to manage a growing workforce and maintain strict compliance with regulatory standards introduces another layer of cost pressure. It is important to monitor
NOTV's ability to control costs, optimize pricing strategies, and efficiently manage its expanding operational infrastructure. Moreover, the company's financial health is also dependent on its ability to secure new contracts and retain its existing client relationships.
A healthy backlog of projects could bolster its financial stability.
Further examination into the company's fundamentals is warranted, including its debt position, cash flow generation, and investment in research and development.
A manageable debt load is critical for financial flexibility, especially given the capital-intensive nature of the drug development services sector. Positive cash flow generation is also essential to enable the company to reinvest in its operations, fund strategic acquisitions, and return value to shareholders. Moreover, NOTV's continued investment in research and development is crucial for innovation, enhancing its service offerings, and maintaining its competitive edge. The company's management's strategic decisions and operational execution would greatly impact the company's future trajectory. Its success will hinge on its capacity to adapt to changes in the market, mitigate challenges, and drive operational efficiencies, all while effectively managing its financial resources.
In summary, Inotiv is predicted to be on a positive trajectory, due to its capacity to cater to the drug discovery and development services. The predicted growth, however, must be viewed with a degree of caution.
The key risk factors include the dependence on clients, contract management, and operational efficiency. Furthermore, any delay in projects, regulatory scrutiny, or economic downturn could significantly impact the company's financial results. Therefore, investors should carefully consider these risks and diligently monitor the company's progress against its stated financial goals.
Rating | Short-Term | Long-Term Senior |
---|---|---|
Outlook | B1 | B3 |
Income Statement | Baa2 | Caa2 |
Balance Sheet | Baa2 | Baa2 |
Leverage Ratios | B3 | C |
Cash Flow | Caa2 | C |
Rates of Return and Profitability | Caa2 | C |
*Financial analysis is the process of evaluating a company's financial performance and position by neural network. It involves reviewing the company's financial statements, including the balance sheet, income statement, and cash flow statement, as well as other financial reports and documents.
How does neural network examine financial reports and understand financial state of the company?
References
- Tibshirani R. 1996. Regression shrinkage and selection via the lasso. J. R. Stat. Soc. B 58:267–88
- Jorgenson, D.W., Weitzman, M.L., ZXhang, Y.X., Haxo, Y.M. and Mat, Y.X., 2023. Can Neural Networks Predict Stock Market?. AC Investment Research Journal, 220(44).
- J. N. Foerster, Y. M. Assael, N. de Freitas, and S. Whiteson. Learning to communicate with deep multi-agent reinforcement learning. In Advances in Neural Information Processing Systems 29: Annual Conference on Neural Information Processing Systems 2016, December 5-10, 2016, Barcelona, Spain, pages 2137–2145, 2016.
- M. J. Hausknecht and P. Stone. Deep recurrent Q-learning for partially observable MDPs. CoRR, abs/1507.06527, 2015
- H. Khalil and J. Grizzle. Nonlinear systems, volume 3. Prentice hall Upper Saddle River, 2002.
- Burkov A. 2019. The Hundred-Page Machine Learning Book. Quebec City, Can.: Andriy Burkov
- Van der Vaart AW. 2000. Asymptotic Statistics. Cambridge, UK: Cambridge Univ. Press