AUC Score :
Short-term Tactic1 :
Dominant Strategy :
Time series to forecast n:
ML Model Testing : Modular Neural Network (Market Direction Analysis)
Hypothesis Testing : Independent T-Test
Surveillance : Major exchange and OTC
1Short-term revised.
2Time series is updated based on short-term trends.
Key Points
HILM's future appears cautiously optimistic, with potential for moderate growth driven by its established market presence in home improvement and hardware. Expansion into new product categories and strategic acquisitions could further fuel revenue streams, potentially leading to increased profitability. However, this outlook carries inherent risks. Economic downturns, shifts in consumer spending habits, and increased competition from larger retailers pose significant challenges. Furthermore, fluctuations in raw material costs and supply chain disruptions could negatively impact margins. Successful execution of its growth strategies and effective management of these external factors will ultimately determine HILM's financial performance.About Hillman Solutions Corp.
Hillman Solutions Corp. (HLMN) is a leading provider of hardware solutions, serving diverse markets across the home improvement, industrial, and automotive sectors. The company's extensive product portfolio encompasses fasteners, key duplication, engraving, and related hardware components, distributed through a vast network of retail partners and direct sales channels. HLMN operates with a strong focus on operational efficiency, leveraging its scale to manage supply chains effectively and maintain competitive pricing.
HLMN's business model emphasizes its ability to deliver innovative and reliable hardware solutions that meet the evolving needs of both professional tradespeople and DIY consumers. The company prioritizes customer relationships and has a proven track record of growth, fueled by strategic acquisitions and the expansion of its product offerings. Hillman's focus remains on providing comprehensive and value-added solutions to its customers while adapting to emerging trends within the hardware industry.
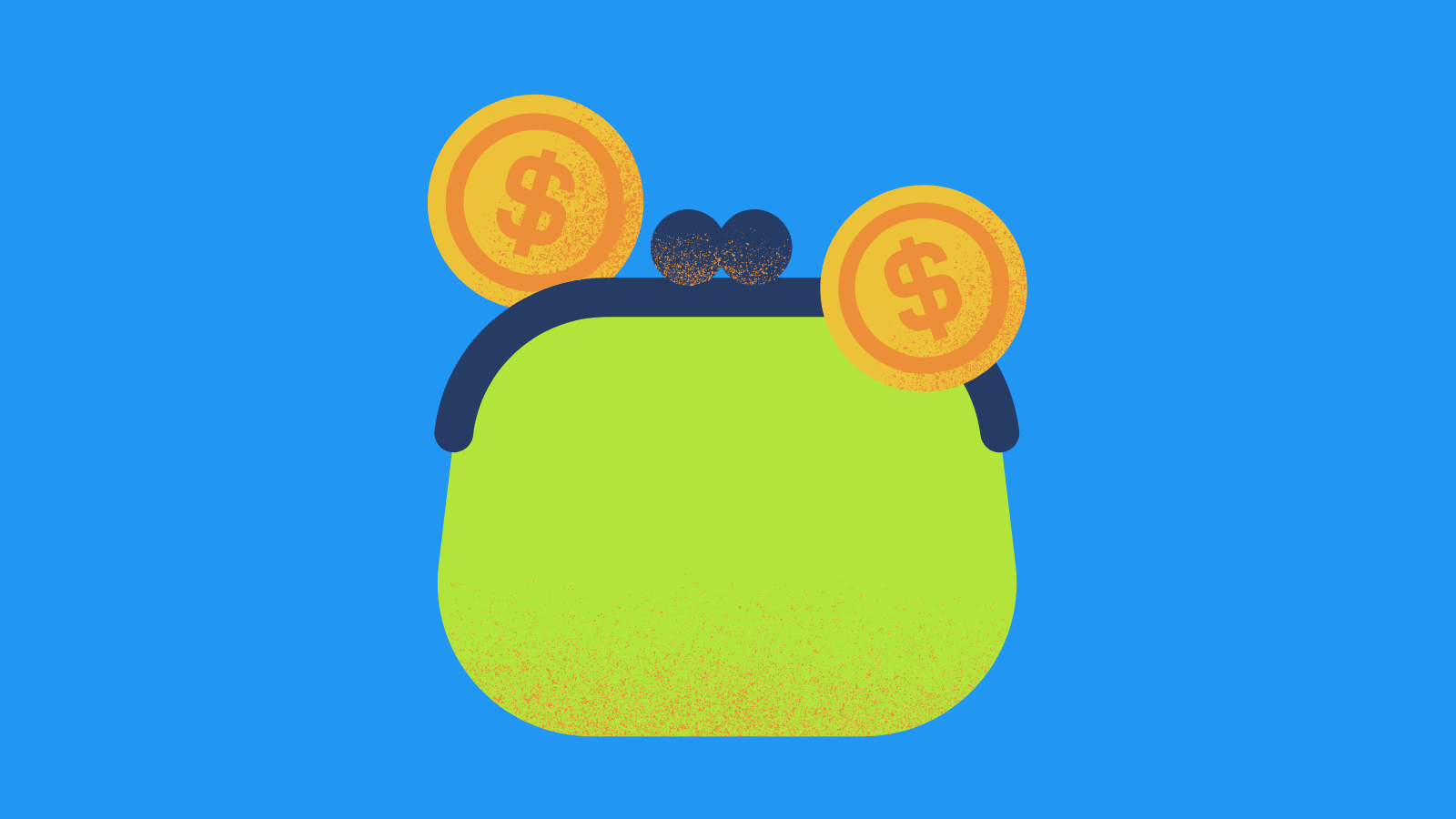
HLMN Stock Prediction Model
The construction of a robust forecasting model for Hillman Solutions Corp. (HLMN) common stock necessitates a comprehensive approach. Our team of data scientists and economists will employ a multifaceted strategy, incorporating a range of relevant variables. The model will be built upon a foundation of historical price data, encompassing daily, weekly, and monthly closing values, adjusted for splits and dividends. This time series data will serve as the primary input for the model. Furthermore, we will integrate economic indicators like inflation rates, GDP growth, and interest rates, which are known to influence market sentiment and company performance. Additional input includes financial statements such as HLMN's revenue, earnings per share (EPS), and debt-to-equity ratio. We will also consider industry-specific factors and competitor data, as well as any pertinent news sentiment, collected through natural language processing techniques from financial news sources and social media.
We will employ a blend of machine learning algorithms. These include Recurrent Neural Networks (RNNs), particularly Long Short-Term Memory (LSTM) networks, to capture the temporal dependencies inherent in stock price movements. Gradient Boosting Machines (GBMs), such as XGBoost or LightGBM, will be utilized to handle the diverse set of input variables and their potential interactions. For feature engineering, we will calculate various technical indicators (Moving Averages, RSI, MACD etc.) to augment the data set. The model training will be done by splitting the historical data into training, validation, and testing sets. Regularization techniques will be implemented to prevent overfitting. Model performance will be assessed using metrics like Mean Absolute Error (MAE), Root Mean Squared Error (RMSE), and the Mean Absolute Percentage Error (MAPE).
The final model will provide a probabilistic forecast of HLMN's future performance, including a predicted price range and confidence intervals for specified time horizons. The output will provide forecasts for short-term, mid-term and long-term predictions. Model validation will be done through backtesting on historical data not used during training and validation. Our data will be updated frequently to adjust any changes in the market. Moreover, we will monitor the model's performance and retrain it periodically to maintain its accuracy and adapt to evolving market dynamics. This cyclical process of monitoring, retraining, and validation ensures the model's continued relevance and its ability to provide actionable insights for HLMN's stock performance.
```
ML Model Testing
n:Time series to forecast
p:Price signals of Hillman Solutions Corp. stock
j:Nash equilibria (Neural Network)
k:Dominated move of Hillman Solutions Corp. stock holders
a:Best response for Hillman Solutions Corp. target price
For further technical information as per how our model work we invite you to visit the article below:
How do KappaSignal algorithms actually work?
Hillman Solutions Corp. Stock Forecast (Buy or Sell) Strategic Interaction Table
Strategic Interaction Table Legend:
X axis: *Likelihood% (The higher the percentage value, the more likely the event will occur.)
Y axis: *Potential Impact% (The higher the percentage value, the more likely the price will deviate.)
Z axis (Grey to Black): *Technical Analysis%
Hillman Solutions Corp. (HLMN): Financial Outlook and Forecast
The financial outlook for HLMN appears cautiously optimistic, with analysts generally projecting a period of moderate growth in the coming years. This positive sentiment stems from several key factors. Firstly, HLMN's strategic focus on the repair, maintenance, and improvement (RMI) market positions it well to benefit from ongoing trends in homeownership and renovation activity. The company's extensive product portfolio, encompassing fasteners, hardware, and related products, caters directly to this sector, which is characterized by resilience even during economic downturns. Secondly, HLMN's ability to maintain a strong presence in both professional and do-it-yourself (DIY) channels provides it with diversified revenue streams, mitigating risks associated with fluctuations in either market segment. The company's continued investments in expanding its e-commerce capabilities and distribution network further enhance its ability to capture market share and drive revenue growth.
In terms of specific financial metrics, analysts anticipate steady revenue growth for HLMN, supported by solid underlying demand and the company's strategic initiatives. Gross margins are expected to remain relatively stable, reflecting the company's ability to manage its cost of goods sold effectively and navigate supply chain challenges. Operating expenses will be closely monitored, and the company's focus on operational efficiency and cost control will be critical in managing profitability. The company has demonstrated its commitment to streamlining operations and integrating acquisitions, which will be crucial in realizing synergies and improving financial performance. Furthermore, HLMN's strong free cash flow generation supports its ability to invest in organic growth initiatives, pursue strategic acquisitions, and return capital to shareholders. The company has also demonstrated a commitment to reducing its debt levels, which will further enhance its financial flexibility.
A key element in HLMN's financial outlook is its ability to successfully integrate recent acquisitions and leverage them for synergistic growth. HLMN has made several strategic acquisitions in recent years, which have expanded its product offerings, geographic reach, and customer base. The integration of these acquired businesses will be crucial in realizing cost savings, increasing revenue through cross-selling, and improving overall operational efficiency. The company's track record of successful acquisitions and its focus on leveraging acquired assets for growth are positive signs. The ability to manage and improve operating margins in these acquisitions will be central to its future performance. Moreover, the ongoing expansion of its product offerings through innovation and the introduction of new solutions caters to evolving consumer needs. The ability to innovate and adapt to market changes is important for HLMN's competitive advantages in the long term.
Overall, the financial forecast for HLMN is positive, reflecting its strong market position, diversified revenue streams, and successful track record. The prediction is that HLMN will continue to grow at a moderate pace in the coming years, driven by sustained demand in the RMI market, successful integration of acquisitions, and its strategic initiatives. However, there are some risks that could impact the company's performance. These include fluctuations in raw material costs, potential disruptions to the supply chain, and intense competition within the hardware and fastener industry. The pace of economic activity, specifically in the housing market, can also impact the company's sales. Therefore, while the outlook is generally favorable, HLMN must maintain operational efficiency, effectively navigate industry challenges, and continuously adapt to changing market dynamics to achieve its growth targets.
Rating | Short-Term | Long-Term Senior |
---|---|---|
Outlook | Ba3 | Ba1 |
Income Statement | Baa2 | Caa2 |
Balance Sheet | Baa2 | Baa2 |
Leverage Ratios | Caa2 | Ba3 |
Cash Flow | Ba3 | Ba3 |
Rates of Return and Profitability | C | Baa2 |
*Financial analysis is the process of evaluating a company's financial performance and position by neural network. It involves reviewing the company's financial statements, including the balance sheet, income statement, and cash flow statement, as well as other financial reports and documents.
How does neural network examine financial reports and understand financial state of the company?
References
- Robins J, Rotnitzky A. 1995. Semiparametric efficiency in multivariate regression models with missing data. J. Am. Stat. Assoc. 90:122–29
- M. Petrik and D. Subramanian. An approximate solution method for large risk-averse Markov decision processes. In Proceedings of the 28th International Conference on Uncertainty in Artificial Intelligence, 2012.
- Chernozhukov V, Chetverikov D, Demirer M, Duflo E, Hansen C, Newey W. 2017. Double/debiased/ Neyman machine learning of treatment effects. Am. Econ. Rev. 107:261–65
- Farrell MH, Liang T, Misra S. 2018. Deep neural networks for estimation and inference: application to causal effects and other semiparametric estimands. arXiv:1809.09953 [econ.EM]
- Chipman HA, George EI, McCulloch RE. 2010. Bart: Bayesian additive regression trees. Ann. Appl. Stat. 4:266–98
- Mazumder R, Hastie T, Tibshirani R. 2010. Spectral regularization algorithms for learning large incomplete matrices. J. Mach. Learn. Res. 11:2287–322
- Rumelhart DE, Hinton GE, Williams RJ. 1986. Learning representations by back-propagating errors. Nature 323:533–36