AUC Score :
Short-term Tactic1 :
Dominant Strategy :
Time series to forecast n:
ML Model Testing : Multi-Instance Learning (ML)
Hypothesis Testing : Spearman Correlation
Surveillance : Major exchange and OTC
1Short-term revised.
2Time series is updated based on short-term trends.
Key Points
Fergusons' future performance appears moderately optimistic, contingent upon several factors. The company is expected to experience sustained revenue growth, driven by continued strength in the construction and renovation markets, coupled with strategic expansions in key product categories. Profit margins might face pressure due to increased competition and potential cost inflation, particularly in raw materials and logistics. Risks include economic downturns impacting construction activity, supply chain disruptions affecting product availability, and fluctuations in commodity prices impacting input costs. Success hinges on Fergusons' ability to manage costs effectively, innovate in its offerings, and maintain strong relationships with its suppliers and customers.About Ferguson Enterprises
Ferguson Enterprises Inc. (FERG) is a leading distributor of plumbing supplies, HVAC/R equipment, waterworks products, and industrial supplies. The company operates primarily in North America, serving both residential and commercial construction markets. FERG's extensive product portfolio and expansive distribution network allow it to meet the diverse needs of its customers, including contractors, municipalities, and industrial facilities. The company emphasizes its commitment to providing high-quality products and exceptional customer service.
FERG has a strong focus on operational efficiency and strategic acquisitions to expand its market reach and product offerings. Its business model is characterized by its ability to maintain strong relationships with both suppliers and customers. FERG is also known for its investments in technology to improve its distribution capabilities, enhance the customer experience, and streamline its internal processes. This strategic approach positions the company as a key player within the building supply industry.
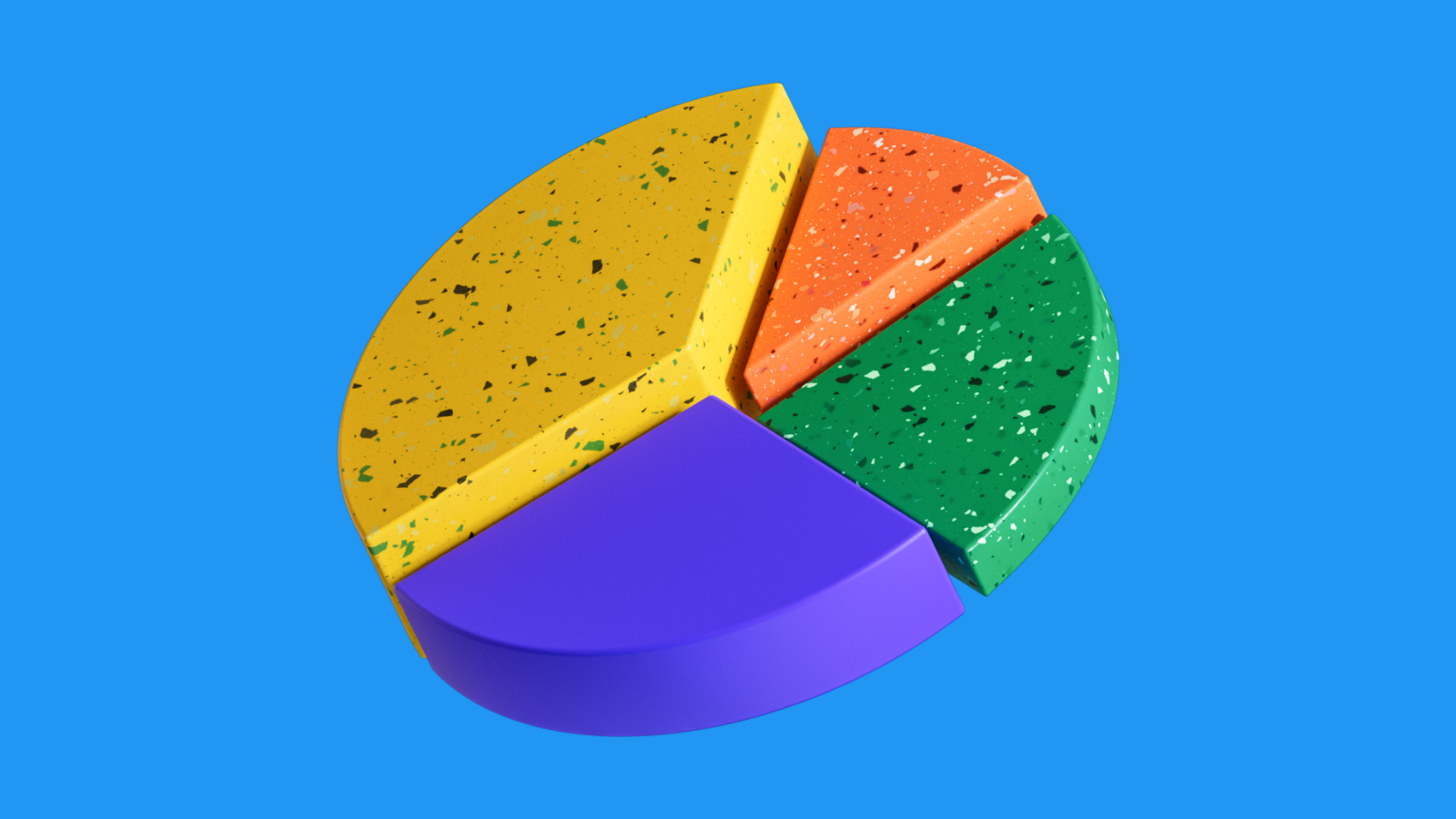
FERG Stock Prediction: A Machine Learning Model
Our team, comprising data scientists and economists, has developed a comprehensive machine learning model to forecast the future performance of Ferguson Enterprises Inc. (FERG) common stock. The model incorporates a multifaceted approach, integrating both fundamental and technical analysis. Fundamental data includes financial statements (revenue, earnings, debt levels, cash flow), industry-specific metrics (demand trends, competitive landscape), and macroeconomic indicators (GDP growth, interest rates, inflation) that could potentially affect FERG's business. Technical analysis focuses on historical price data and trading volume, utilizing various technical indicators like moving averages, Relative Strength Index (RSI), and Bollinger Bands to identify patterns and predict price movements. The model leverages a variety of machine learning algorithms, including Recurrent Neural Networks (RNNs), known for their ability to process sequential data and identify time-series patterns, and Gradient Boosting Machines, which offer strong predictive power through ensemble learning.
The model's architecture consists of several key stages. First, we collect and clean a vast dataset encompassing the aforementioned fundamental and technical data. This involves careful data cleaning, handling missing values, and transforming the data into a suitable format for the machine learning algorithms. Secondly, we conduct feature engineering, where we create new features from the raw data to improve model accuracy. Examples include calculating financial ratios, generating lagged values of technical indicators, and creating interaction terms. The dataset is then split into training, validation, and testing sets. The training set is used to train the model, the validation set is used to tune hyperparameters and prevent overfitting, and the test set is used to evaluate the model's performance on unseen data. Hyperparameter optimization is performed to tune the model and improve its ability to forecast the stock performance. Regular model updates will be performed to incorporate new information.
The final product of our analysis is a probabilistic forecast of FERG's stock movement. This includes not only a point estimate (predicted stock performance) but also a confidence interval, providing a measure of the model's uncertainty. The model's output is regularly reviewed and validated against actual market data. This allows us to monitor model performance, identify potential weaknesses, and continuously refine and improve its predictive capabilities. Furthermore, we will conduct sensitivity analyses to determine the impact of various economic scenarios on the forecast, offering insights to guide investment decisions and risk management strategies. Regular model retraining will be essential to account for new data.
```
ML Model Testing
n:Time series to forecast
p:Price signals of Ferguson Enterprises stock
j:Nash equilibria (Neural Network)
k:Dominated move of Ferguson Enterprises stock holders
a:Best response for Ferguson Enterprises target price
For further technical information as per how our model work we invite you to visit the article below:
How do KappaSignal algorithms actually work?
Ferguson Enterprises Stock Forecast (Buy or Sell) Strategic Interaction Table
Strategic Interaction Table Legend:
X axis: *Likelihood% (The higher the percentage value, the more likely the event will occur.)
Y axis: *Potential Impact% (The higher the percentage value, the more likely the price will deviate.)
Z axis (Grey to Black): *Technical Analysis%
Ferguson Enterprises Inc. Common Stock Financial Outlook and Forecast
The financial outlook for Ferguson, a leading distributor of plumbing supplies, HVAC equipment, and industrial products, appears cautiously optimistic. The company has demonstrated robust performance in recent years, driven by its strong market position, extensive distribution network, and focus on serving diverse customer segments. This success is underpinned by a proven ability to navigate economic cycles and maintain profitability even during periods of market volatility. Key factors contributing to this positive outlook include the ongoing strength in the residential construction market, increased infrastructure spending, and the company's strategic initiatives focused on e-commerce and operational efficiency. Furthermore, the company's diverse product portfolio mitigates risk by allowing it to capitalize on various sectors' growth. The company is also well-positioned to benefit from the growing trend of sustainable building practices and eco-friendly products, which are gaining traction in the construction and industrial sectors, opening new avenues for revenue and market share expansion.
Several factors support a favorable financial forecast for the coming years. Firstly, the company's ability to leverage its vast distribution network, encompassing a large number of locations across North America, provides a significant competitive advantage. This infrastructure enables efficient product delivery and responsive customer service, bolstering customer loyalty and repeat business. Secondly, the company's strategic focus on e-commerce and digital initiatives is proving successful. The investment in online platforms and digital tools has improved the company's ability to interact with customers, gain insights into market trends, and improve its supply chain efficiency. This digital transformation has enhanced its revenue streams and reduced operational costs. Thirdly, the company's strategic acquisitions and expansions into new markets have facilitated diversification and growth. Furthermore, strong financial management, including effective inventory control and cost management, bolsters its financial stability.
Analysts project continued revenue and earnings growth for the company in the coming period. These forecasts anticipate moderate growth, reflecting the cyclical nature of the construction industry and broader economic conditions. The positive factors mentioned, alongside favorable macroeconomic conditions, such as increased infrastructure spending and a stable housing market, are expected to fuel expansion. The focus on higher-margin products and services, alongside continuous operational improvements, will also contribute to increased profitability. The company's proven track record of managing its debt and generating strong cash flow further reinforces the positive financial outlook. Strategic initiatives, such as expanding its presence in the waterworks and industrial markets, should contribute to revenue streams and further increase its market share.
Based on current market conditions and the company's strategic positioning, a positive growth trajectory is predicted. The company is well-placed to capitalize on the current market trends and the construction sector's projected expansion. However, there are also risks associated with this positive prediction. Economic downturns, which would lead to decreased spending in the construction market, could significantly affect revenue and earnings. Additionally, escalating raw material costs and supply chain disruptions could negatively impact its profitability. Intense competition within the distribution market and potential regulatory changes add to the complexities. Management's effective navigation of these risks and successful implementation of its strategic initiatives will be crucial in achieving the expected financial performance.
Rating | Short-Term | Long-Term Senior |
---|---|---|
Outlook | Baa2 | Ba1 |
Income Statement | Baa2 | Baa2 |
Balance Sheet | Baa2 | Baa2 |
Leverage Ratios | Ba1 | Caa2 |
Cash Flow | Baa2 | Caa2 |
Rates of Return and Profitability | Baa2 | Baa2 |
*Financial analysis is the process of evaluating a company's financial performance and position by neural network. It involves reviewing the company's financial statements, including the balance sheet, income statement, and cash flow statement, as well as other financial reports and documents.
How does neural network examine financial reports and understand financial state of the company?
References
- Morris CN. 1983. Parametric empirical Bayes inference: theory and applications. J. Am. Stat. Assoc. 78:47–55
- V. Borkar. Q-learning for risk-sensitive control. Mathematics of Operations Research, 27:294–311, 2002.
- N. B ̈auerle and J. Ott. Markov decision processes with average-value-at-risk criteria. Mathematical Methods of Operations Research, 74(3):361–379, 2011
- C. Szepesvári. Algorithms for Reinforcement Learning. Synthesis Lectures on Artificial Intelligence and Machine Learning. Morgan & Claypool Publishers, 2010
- V. Konda and J. Tsitsiklis. Actor-Critic algorithms. In Proceedings of Advances in Neural Information Processing Systems 12, pages 1008–1014, 2000
- Athey S, Imbens G, Wager S. 2016a. Efficient inference of average treatment effects in high dimensions via approximate residual balancing. arXiv:1604.07125 [math.ST]
- Bewley, R. M. Yang (1998), "On the size and power of system tests for cointegration," Review of Economics and Statistics, 80, 675–679.