AUC Score :
Short-term Tactic1 :
Dominant Strategy :
Time series to forecast n:
ML Model Testing : Reinforcement Machine Learning (ML)
Hypothesis Testing : Independent T-Test
Surveillance : Major exchange and OTC
1Short-term revised.
2Time series is updated based on short-term trends.
Key Points
The TR/CC CRB Corn index is projected to experience volatile trading conditions. The index may encounter a period of price increases as a result of concerns regarding weather patterns and their impact on global yields. However, the index faces substantial downside risks; a slowdown in global economic growth and increased production from major corn producing regions could pressure prices downwards. Overall, the index's trajectory remains highly uncertain, with potential for significant price swings driven by supply-demand dynamics, geopolitical factors, and shifts in investor sentiment.About TR/CC CRB Corn Index
The Thomson Reuters/CoreCommodity CRB Index, often referred to as the CRB Index, is a benchmark reflecting the price movements of a basket of commodities. It is designed to provide a broad measure of commodity market performance and is a widely followed indicator of inflation expectations and overall economic health. The index includes a diverse selection of raw materials, encompassing energy products, precious metals, industrial metals, and agricultural commodities. Weights within the index are determined based on historical trading volume and liquidity, ensuring that the index reflects the relative importance of each commodity in the global market.
The CRB Index serves as a valuable tool for investors, providing a means to track the commodity market's performance and to diversify portfolios. It can also be utilized as a hedging instrument against inflation or economic uncertainty. Furthermore, the index is frequently employed by economists and financial analysts to assess trends in commodity prices and their potential impact on the broader economy. Its composition and methodology are designed to provide a representative and easily accessible measure of the overall commodity market's activity.
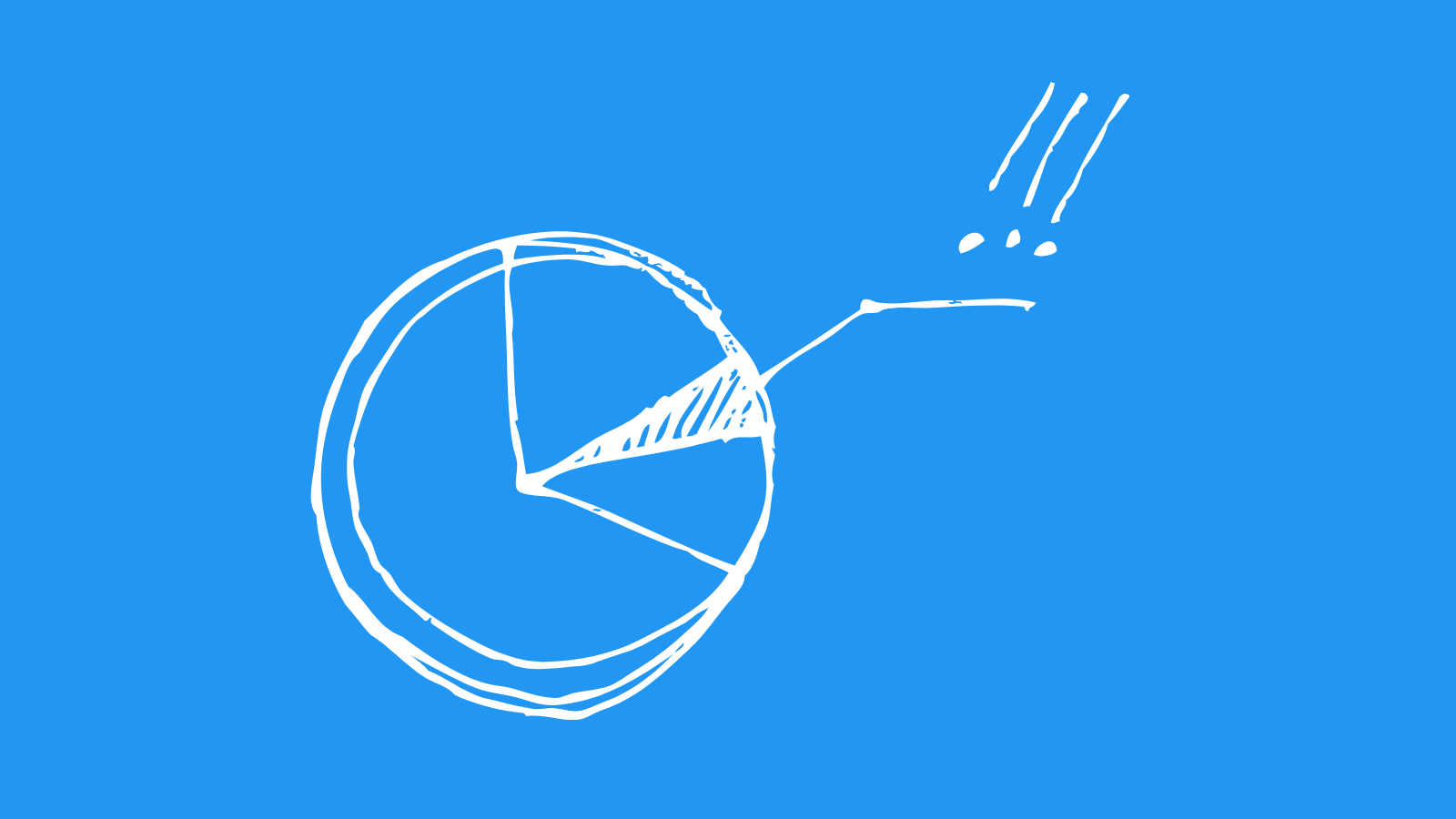
Machine Learning Model for TR/CC CRB Corn Index Forecasting
Our team, composed of data scientists and economists, has developed a machine learning model for forecasting the TR/CC CRB Corn Index. The model utilizes a comprehensive dataset incorporating various economic and agricultural indicators to provide accurate predictions. Key features incorporated include global corn supply and demand dynamics, such as production levels in major corn-producing countries like the United States, Brazil, and Ukraine; weather patterns and climate data, including temperature, rainfall, and soil moisture, which significantly impact crop yields; trade data, comprising import and export volumes, and inventory levels. Furthermore, our model considers macroeconomic factors, such as the US dollar index, interest rates, and inflation, all of which influence commodity prices. Advanced feature engineering techniques are applied to transform raw data into formats suitable for the algorithms, including lagged variables, rolling averages, and seasonality adjustments.
We have implemented a multi-model approach, employing a combination of machine learning algorithms to enhance forecasting accuracy. Specifically, we are utilizing Random Forest, Gradient Boosting, and a Long Short-Term Memory (LSTM) recurrent neural network. The Random Forest and Gradient Boosting models are beneficial for capturing non-linear relationships between input variables and the corn index, while the LSTM network is designed to effectively handle the temporal dependencies and sequential nature of time series data. Each model is trained using historical TR/CC CRB Corn Index data alongside the economic and agricultural indicators. The models are then rigorously evaluated using various performance metrics, including Mean Absolute Error (MAE), Root Mean Squared Error (RMSE), and R-squared, based on the historical dataset split for training and testing purposes. In addition, the ensemble methods are implemented to merge the models.
The final forecasting output is generated by an ensemble method, combining the predictions from each individual model. We employ weighted averaging, where the weights for each model are determined based on its historical performance during the validation phase. Regular model retraining and updates are incorporated as new data become available, to maintain the accuracy of the forecasts. Our team also performs scenario analysis and risk assessment to explore potential fluctuations in the TR/CC CRB Corn Index, and provides comprehensive reports detailing the forecasting methodology, assumptions, and prediction with confidence intervals. These reports include recommendations to support stakeholders' decision-making in the commodity market.
ML Model Testing
n:Time series to forecast
p:Price signals of TR/CC CRB Corn index
j:Nash equilibria (Neural Network)
k:Dominated move of TR/CC CRB Corn index holders
a:Best response for TR/CC CRB Corn target price
For further technical information as per how our model work we invite you to visit the article below:
How do KappaSignal algorithms actually work?
TR/CC CRB Corn Index Forecast Strategic Interaction Table
Strategic Interaction Table Legend:
X axis: *Likelihood% (The higher the percentage value, the more likely the event will occur.)
Y axis: *Potential Impact% (The higher the percentage value, the more likely the price will deviate.)
Z axis (Grey to Black): *Technical Analysis%
TR/CC CRB Corn Index: Financial Outlook and Forecast
The TR/CC CRB Corn Index, a commodity index tracking the price movements of corn futures, presents a multifaceted financial outlook influenced by a complex interplay of global supply and demand dynamics, geopolitical events, and evolving agricultural practices. Currently, the index's trajectory is subject to considerable volatility, reflecting the sensitivity of corn prices to fluctuating production levels, trade policies, and the ever-present uncertainties of weather patterns. Understanding these variables is crucial for assessing the index's future performance. Demand, driven by both human consumption and livestock feed, remains a key driver. However, increasing usage for ethanol production and other industrial applications further complicate the equation. Simultaneously, supply is influenced by planting decisions across the globe, yields affected by weather, and the accumulation of global stockpiles. The index's performance is therefore a barometer of these diverse forces, requiring investors and market participants to closely monitor these factors for a comprehensive understanding of market trends.
The outlook for the TR/CC CRB Corn Index also depends on several important external forces. Geopolitical developments and international trade regulations play a crucial role. Trade disputes, tariff implementations, and shifts in import/export policies across major corn-producing and consuming nations can significantly affect price levels. Moreover, currency fluctuations, specifically the value of the US dollar (USD) in which corn futures are typically denominated, can add another layer of complexity to the index's valuation. Economic growth, particularly in emerging markets with high corn consumption, can stimulate demand. The index's long-term potential hinges on technological innovation within agriculture, including genetic modifications, precision farming, and improved irrigation techniques. These advancements can lead to increased yields and productivity, potentially influencing price stability, while also introducing new challenges related to sustainable agricultural practices and environmental concerns.
Furthermore, analysis of the TR/CC CRB Corn Index necessitates a careful assessment of regional variations. Climate change, erratic weather patterns, and the frequency of extreme weather events, such as droughts, floods, and heat waves, pose significant challenges to corn production globally. North America, South America, and Europe are the main production regions for corn. The specific climate conditions in each of these regions are critical, and therefore monitoring the weather and potential harvest outcomes in these geographies is essential to any accurate forecast. Government policies related to biofuels, agricultural subsidies, and environmental regulations can shape the supply side of the corn market and further influence pricing dynamics. This highlights that the dynamics of the index are both globally interconnected and locally sensitive.
Considering the factors outlined above, a cautiously optimistic prediction can be posited for the TR/CC CRB Corn Index. The increasing global population and continued growth in biofuel demand should provide support for corn prices. However, this positive outlook is accompanied by risks. Adverse weather events, trade uncertainties, and geopolitical tensions could disrupt supply chains and cause price volatility, potentially leading to periods of bearish movement. Moreover, technological advancements in agriculture, along with increased global corn stockpiles, could potentially depress prices. Therefore, investors and market participants should adopt a balanced approach, remaining vigilant to market signals and adjusting their strategies accordingly.
Rating | Short-Term | Long-Term Senior |
---|---|---|
Outlook | Caa2 | Ba2 |
Income Statement | Caa2 | Baa2 |
Balance Sheet | C | B1 |
Leverage Ratios | C | Ba1 |
Cash Flow | C | B3 |
Rates of Return and Profitability | Baa2 | B1 |
*An aggregate rating for an index summarizes the overall sentiment towards the companies it includes. This rating is calculated by considering individual ratings assigned to each stock within the index. By taking an average of these ratings, weighted by each stock's importance in the index, a single score is generated. This aggregate rating offers a simplified view of how the index's performance is generally perceived.
How does neural network examine financial reports and understand financial state of the company?
References
- Bastani H, Bayati M. 2015. Online decision-making with high-dimensional covariates. Work. Pap., Univ. Penn./ Stanford Grad. School Bus., Philadelphia/Stanford, CA
- Keane MP. 2013. Panel data discrete choice models of consumer demand. In The Oxford Handbook of Panel Data, ed. BH Baltagi, pp. 54–102. Oxford, UK: Oxford Univ. Press
- R. Howard and J. Matheson. Risk sensitive Markov decision processes. Management Science, 18(7):356– 369, 1972
- A. Shapiro, W. Tekaya, J. da Costa, and M. Soares. Risk neutral and risk averse stochastic dual dynamic programming method. European journal of operational research, 224(2):375–391, 2013
- M. J. Hausknecht and P. Stone. Deep recurrent Q-learning for partially observable MDPs. CoRR, abs/1507.06527, 2015
- F. A. Oliehoek and C. Amato. A Concise Introduction to Decentralized POMDPs. SpringerBriefs in Intelligent Systems. Springer, 2016
- Babula, R. A. (1988), "Contemporaneous correlation and modeling Canada's imports of U.S. crops," Journal of Agricultural Economics Research, 41, 33–38.