AUC Score :
Short-term Tactic1 :
Dominant Strategy :
Time series to forecast n:
ML Model Testing : Supervised Machine Learning (ML)
Hypothesis Testing : Spearman Correlation
Surveillance : Major exchange and OTC
1Short-term revised.
2Time series is updated based on short-term trends.
Key Points
CNR's future appears contingent on several factors. Based on current trends, the company may experience moderate revenue growth driven by fluctuations in commodity prices, particularly in the energy sector. However, profitability remains uncertain due to volatile operational costs and potential shifts in market demand. Risk factors include regulatory changes affecting resource extraction, unforeseen geopolitical instability impacting global supply chains, and intense competition from established players. Investors should be aware of the potential for increased volatility and the need for careful monitoring of market dynamics.About Core Natural Resources Inc.
Core Natural Resources Inc. is an energy company focused on the acquisition, development, and production of oil and natural gas properties. The company primarily operates in North America, with a strategic emphasis on exploring and extracting hydrocarbons from established resource basins. Its operational strategy includes leveraging advanced drilling and completion techniques to maximize production efficiency and enhance the economic viability of its projects. Additionally, the company actively manages its portfolio through strategic asset sales and acquisitions to optimize its reserves and production profile.
The company strives to create value for its shareholders through responsible resource management, operational excellence, and prudent financial strategies. Core Natural Resources is committed to adhering to stringent environmental regulations and safety standards. The firm's management team is focused on sustainable growth and aims to capitalize on opportunities within the dynamic energy market. Core Natural Resources Inc. focuses on maximizing returns while maintaining financial stability and reducing operational risks.
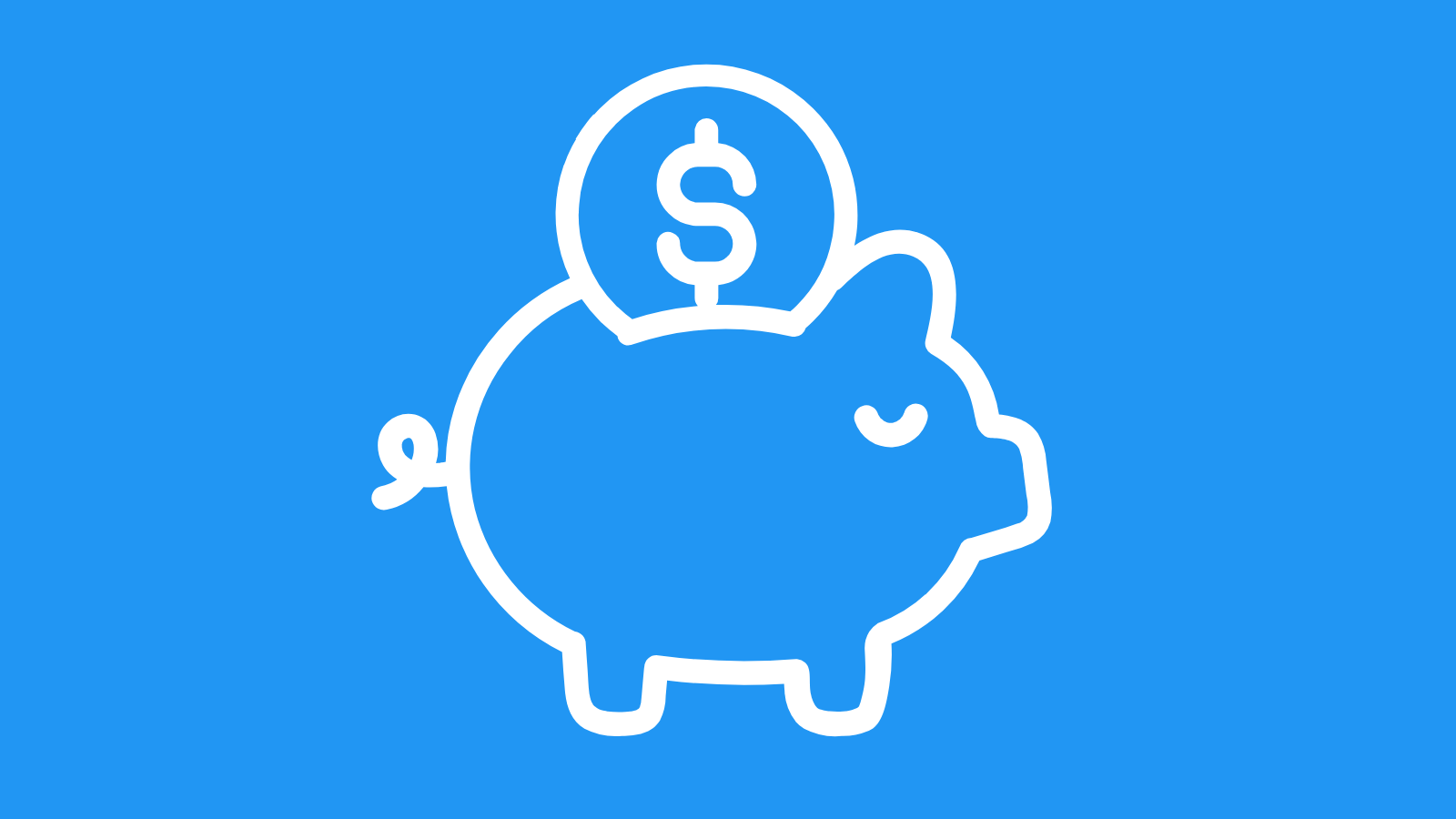
Machine Learning Model for CNR Stock Forecast
The objective is to construct a robust machine learning model for forecasting the performance of Core Natural Resources Inc. (CNR) stock. The model will integrate a diverse range of financial and economic indicators. Key data inputs will encompass historical stock price data, volume traded, and financial statements (including revenue, earnings, and debt levels). Macroeconomic variables, such as inflation rates, interest rates, commodity prices (specifically those relevant to natural resources), and relevant economic growth indicators will be incorporated. Feature engineering will play a crucial role in generating informative variables, incorporating technical indicators (e.g., moving averages, RSI, MACD) and transforming raw data to enhance model performance. The model will be optimized for predictive accuracy and also for stability over time, which is crucial for generating consistent forecasts.
The model selection process involves assessing various machine learning algorithms to identify the most suitable approach. Candidate algorithms include time series models (e.g., ARIMA, Prophet), regression models (e.g., linear regression, random forest), and potentially neural networks (e.g., LSTMs), particularly if handling of complex patterns is necessary. Model evaluation will be conducted using a rigorous process. The dataset will be split into training, validation, and test sets to ensure the model generalizes well to unseen data. Performance metrics such as Mean Squared Error (MSE), Root Mean Squared Error (RMSE), Mean Absolute Error (MAE), and potentially, direction accuracy, will be used to assess the model's forecasting ability. Feature importance analysis will be utilized to understand the influence of different variables on the forecast.
The final deliverable will be a production-ready model and supporting documentation. This includes the developed machine learning model, comprehensive documentation detailing data sources, model architecture, feature engineering techniques, training procedures, validation results, and a guide on how to use the model and how to interpret the forecast. The model will be designed to be updated with new data periodically to ensure its performance remains optimal. We will also provide a monitoring framework to track the model's performance over time and identify any potential drift, indicating the necessity of model retraining. Regular reports will summarize the model's performance, incorporating an analysis of forecast accuracy and the identification of any influential market events.
ML Model Testing
n:Time series to forecast
p:Price signals of Core Natural Resources Inc. stock
j:Nash equilibria (Neural Network)
k:Dominated move of Core Natural Resources Inc. stock holders
a:Best response for Core Natural Resources Inc. target price
For further technical information as per how our model work we invite you to visit the article below:
How do KappaSignal algorithms actually work?
Core Natural Resources Inc. Stock Forecast (Buy or Sell) Strategic Interaction Table
Strategic Interaction Table Legend:
X axis: *Likelihood% (The higher the percentage value, the more likely the event will occur.)
Y axis: *Potential Impact% (The higher the percentage value, the more likely the price will deviate.)
Z axis (Grey to Black): *Technical Analysis%
Core Natural Resources Inc. Financial Outlook and Forecast
The financial outlook for CNR, Inc.'s common stock presents a mixed picture, influenced by the cyclical nature of the natural resources sector and specific company-level factors. The company's performance will likely hinge on its ability to capitalize on existing resource assets and strategically acquire new opportunities. Commodity price fluctuations, particularly in the key resources CNR focuses on, will continue to be a significant driver of revenue and profitability. Furthermore, operational efficiency, including cost management and successful project execution, will be critical for maintaining or improving profit margins. CNR's strategic decisions related to capital allocation, debt management, and shareholder returns will also heavily impact investor sentiment and, ultimately, the stock's performance. The company's ability to adapt to changing market dynamics and technological advancements in resource extraction and exploration will be crucial for long-term sustainability.
Forecasting CNR's financial performance requires a deep dive into the company's specific assets, including proven reserves, production capacity, and geographic location. Analyzing the demand outlook for the resources CNR extracts is crucial. This involves assessing macroeconomic factors, global consumption patterns, and any potential shifts in industry dynamics. The company's exploration and development pipeline also matters, with any promising discoveries or successful project completions likely to positively impact the stock's valuation. Investors should carefully evaluate the management team's track record in deploying capital, managing risks, and navigating regulatory hurdles. Furthermore, any partnerships or joint ventures that CNR enters into can provide access to new resources, expertise, and markets, potentially enhancing the company's growth prospects. Examining its financial statements – income statements, balance sheets, and cash flow statements – will be crucial to track the company's operating performance, financial health, and ability to generate cash.
For a more detailed perspective, consider the factors that might influence CNR's revenue and earnings over the next few years. Rising commodity prices in CNR's key resource areas could lead to significant revenue growth, particularly if the company can increase production or improve its sales strategy. Successful cost-cutting initiatives and operational efficiencies could lead to expanded profit margins. The company may face challenges due to factors like government regulation, market sentiment, and unexpected disruptions. Positive developments, such as favorable exploration outcomes or successful project implementations, will likely boost investor optimism. However, this must be tempered by a realistic assessment of potential risks, including commodity price volatility, geopolitical instability, and unforeseen operational difficulties.
In conclusion, the financial forecast for CNR, Inc. is generally cautiously optimistic, especially if commodity prices are favorable. I predict that CNR's stock has the potential for moderate growth over the next few years. However, this projection comes with inherent risks. The most significant risk is the volatility of commodity prices, which can significantly impact earnings. Furthermore, the company is exposed to geopolitical risks and may experience unforeseen operational setbacks. Additional risks include changes in government regulations, environmental challenges, and shifts in demand. Despite these, I suggest the company has a good chance of success if they can properly manage their company.
Rating | Short-Term | Long-Term Senior |
---|---|---|
Outlook | B2 | B1 |
Income Statement | C | Baa2 |
Balance Sheet | B3 | Baa2 |
Leverage Ratios | B1 | Caa2 |
Cash Flow | Baa2 | Ba3 |
Rates of Return and Profitability | B1 | C |
*Financial analysis is the process of evaluating a company's financial performance and position by neural network. It involves reviewing the company's financial statements, including the balance sheet, income statement, and cash flow statement, as well as other financial reports and documents.
How does neural network examine financial reports and understand financial state of the company?
References
- Firth JR. 1957. A synopsis of linguistic theory 1930–1955. In Studies in Linguistic Analysis (Special Volume of the Philological Society), ed. JR Firth, pp. 1–32. Oxford, UK: Blackwell
- Bastani H, Bayati M. 2015. Online decision-making with high-dimensional covariates. Work. Pap., Univ. Penn./ Stanford Grad. School Bus., Philadelphia/Stanford, CA
- Bottomley, P. R. Fildes (1998), "The role of prices in models of innovation diffusion," Journal of Forecasting, 17, 539–555.
- R. Rockafellar and S. Uryasev. Optimization of conditional value-at-risk. Journal of Risk, 2:21–42, 2000.
- S. Bhatnagar. An actor-critic algorithm with function approximation for discounted cost constrained Markov decision processes. Systems & Control Letters, 59(12):760–766, 2010
- Jorgenson, D.W., Weitzman, M.L., ZXhang, Y.X., Haxo, Y.M. and Mat, Y.X., 2023. Can Neural Networks Predict Stock Market?. AC Investment Research Journal, 220(44).
- S. Proper and K. Tumer. Modeling difference rewards for multiagent learning (extended abstract). In Proceedings of the Eleventh International Joint Conference on Autonomous Agents and Multiagent Systems, Valencia, Spain, June 2012