AUC Score :
Short-term Tactic1 :
Dominant Strategy :
Time series to forecast n:
ML Model Testing : Supervised Machine Learning (ML)
Hypothesis Testing : Statistical Hypothesis Testing
Surveillance : Major exchange and OTC
1Short-term revised.
2Time series is updated based on short-term trends.
Key Points
Acacia Tech's future hinges on successful monetization of its patent portfolio and the ability to secure favorable settlements or licensing agreements. It is predicted that revenue will fluctuate depending on the timing and size of these deals, with periods of high growth interspersed with slower times. Risk factors include the complexities of intellectual property litigation, the potential for patent invalidation, and the dependence on specific technologies and industries. There is also the risk that Acacia Tech might face stiff competition from other IP monetization firms. The company's ability to adapt to evolving technological landscapes and effectively manage its portfolio will be critical for its long-term success, coupled with the possibility of lower returns if litigation costs rise significantly or if the overall market for patent licensing cools down.About Acacia Research
Acacia Tech is a publicly traded company that focuses on acquiring, developing, and licensing patented technologies. It operates within the intellectual property (IP) sector, primarily concentrating on enforcing and monetizing its patent portfolio across various industries. The company's business model revolves around identifying and acquiring patents, often from universities, research institutions, and other entities. It then seeks to license these patents to companies that are using the protected technologies in their products and services. Acacia Tech's revenue generation relies heavily on securing licensing agreements and, when necessary, litigating to protect its IP rights.
The company's strategy often involves acquiring patents in high-growth technological areas. It then attempts to generate revenue by licensing these technologies to companies. Acacia Tech's business performance is directly tied to the success of its licensing efforts, the enforceability of its patents, and the overall market demand for the underlying technologies. It navigates the complexities of intellectual property law and the challenges of successfully enforcing its patent portfolio against potential infringers.
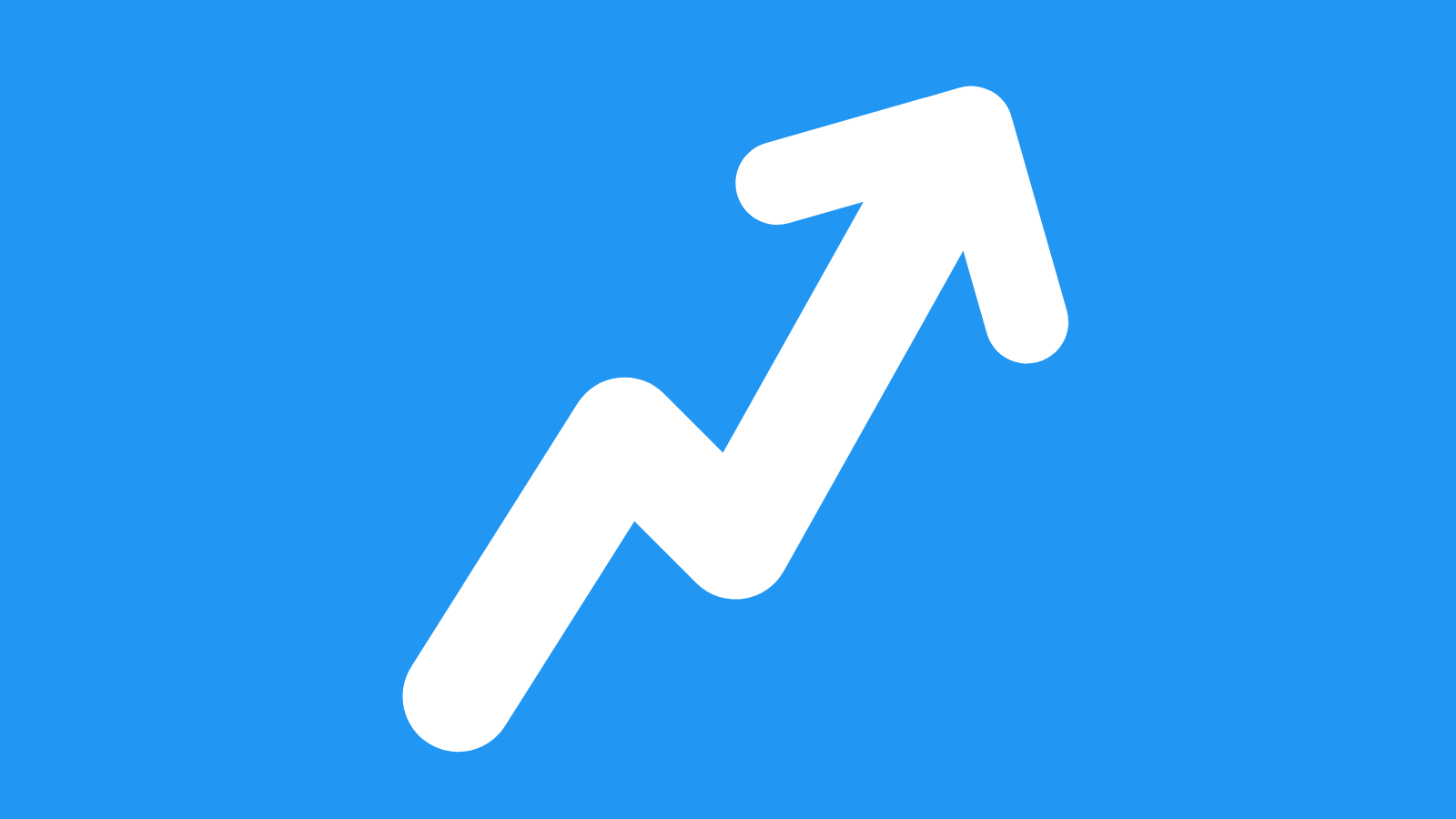
ACTG Stock Forecast Model: A Data Science and Economic Approach
Our interdisciplinary team of data scientists and economists has developed a machine learning model to forecast the performance of Acacia Research Corporation (ACTG) common stock. The model integrates diverse data sources, including historical stock price data, financial statements, macroeconomic indicators, and industry-specific news and sentiment analysis. We employ a range of algorithms, such as Recurrent Neural Networks (RNNs), particularly Long Short-Term Memory (LSTM) networks, known for their ability to handle sequential data and capture temporal dependencies. Furthermore, we incorporate ensemble methods to leverage the strengths of multiple models, enhancing prediction accuracy and robustness. This comprehensive approach allows us to consider both internal company-specific factors and external market dynamics that influence ACTG's stock value.
The model's core functionality involves feature engineering and time series analysis. We meticulously process raw data to create informative features, including technical indicators (Moving Averages, RSI, MACD), fundamental metrics (Revenue, Earnings per Share, Debt-to-Equity ratio), and sentiment scores derived from news articles and social media. The time series analysis component analyzes the historical performance to understand the temporal trends and seasonality patterns. The model is trained using a large dataset, spanning a significant period to ensure comprehensive learning. The performance of the model is measured through the usage of cross-validation techniques to evaluate the model's ability to predict future stock behavior, calculating metrics such as Mean Squared Error (MSE), Root Mean Squared Error (RMSE), and the R-squared value.
The economic considerations are integrated into the model through macro economic indicator analysis such as GDP growth rates, interest rate trends, inflation rates, and sector-specific performance. The model analyzes the impact of economic cycles and policy changes on the market conditions and incorporate these factors into the model's predictive capabilities. The output of the model will be a forecast, along with a confidence interval, providing insights for potential investors or company's management. The model will require constant updates and refinement, incorporating new data and adapting to evolving market dynamics. Ongoing monitoring and evaluation will be crucial to ensure its accuracy and effectiveness, allowing it to remain a valuable tool for understanding ACTG stock's future outlook.
```
ML Model Testing
n:Time series to forecast
p:Price signals of Acacia Research stock
j:Nash equilibria (Neural Network)
k:Dominated move of Acacia Research stock holders
a:Best response for Acacia Research target price
For further technical information as per how our model work we invite you to visit the article below:
How do KappaSignal algorithms actually work?
Acacia Research Stock Forecast (Buy or Sell) Strategic Interaction Table
Strategic Interaction Table Legend:
X axis: *Likelihood% (The higher the percentage value, the more likely the event will occur.)
Y axis: *Potential Impact% (The higher the percentage value, the more likely the price will deviate.)
Z axis (Grey to Black): *Technical Analysis%
Acacia Tech Financial Outlook and Forecast
The financial outlook for Acacia Tech is influenced by its business model, which centers on intellectual property (IP) monetization. This strategy involves acquiring and licensing patents, then pursuing royalties or asserting infringement claims. A key driver of future earnings will be the successful enforcement of its existing patent portfolio and the acquisition of new, valuable IP assets. The company's ability to effectively litigate and negotiate licensing agreements is crucial to realizing returns on its investments. Further, the technology landscape is constantly evolving; Acacia Tech must stay abreast of technological advancements and target attractive sectors to acquire patents with high monetization potential. Successful execution of licensing agreements, favorable court decisions, and a continuous pipeline of new patents are essential for sustained revenue growth. The company's financial performance also hinges on the macroeconomic environment, as economic downturns can reduce the demand for licensed technologies and increase the risk of non-payment from licensees.
Forecasting Acacia Tech's future performance requires a detailed assessment of several key factors. The number and quality of its patent assets are primary considerations. Analysts closely watch the status of ongoing litigation cases and the progress of licensing negotiations, which directly affect revenue streams. The technological areas in which the company focuses on will significantly influence its prospects. Patents related to rapidly growing sectors, such as artificial intelligence, 5G, or cloud computing, may provide significant returns on investments. The success rate of litigation and the terms of licensing agreements negotiated will shape profit margins and cash flow. Moreover, Acacia Tech's ability to efficiently manage its operating expenses, including legal costs and patent acquisition costs, is critical to maintain profitability. Transparency in its reporting and disclosure of patent portfolio information are crucial for attracting investor confidence and facilitating accurate financial projections.
The financial forecast for Acacia Tech is cautiously optimistic, given its inherent business risks. The company's revenue is susceptible to volatility tied to the timing and outcome of patent-related litigation and licensing activities. Potential future acquisitions of strategic patent portfolios could provide significant growth opportunities, but these are also associated with integration and due diligence costs. While patent monetization can offer substantial returns, the process involves substantial upfront investment and the likelihood of protracted legal battles. Moreover, the shifting legal and regulatory landscape surrounding IP rights globally can influence the ease and effectiveness of patent enforcement. The company's financial health depends on its ability to adapt to evolving technology trends, identify new licensing opportunities, and maintain robust relationships with legal and technology experts.
Overall, the financial outlook for Acacia Tech is moderately positive. The company possesses the potential for significant revenue generation through IP monetization, particularly in high-growth technological areas. The key to success will be a skillful approach to patent acquisitions, litigations, and licensing agreements. However, the forecast is tempered by inherent risks. The volatile nature of patent litigation, the inherent uncertainty around legal outcomes, and the dependence on a limited number of licensing deals pose significant challenges. Therefore, the primary risks include unfavorable court rulings, unsuccessful litigation campaigns, and an inability to license its patents effectively. Negative events like changes in patent laws and technological obsolescence pose additional risks that could adversely affect its financial performance.
Rating | Short-Term | Long-Term Senior |
---|---|---|
Outlook | B2 | B2 |
Income Statement | Ba1 | Caa2 |
Balance Sheet | Ba2 | Baa2 |
Leverage Ratios | B2 | B3 |
Cash Flow | Caa2 | Caa2 |
Rates of Return and Profitability | C | Caa2 |
*Financial analysis is the process of evaluating a company's financial performance and position by neural network. It involves reviewing the company's financial statements, including the balance sheet, income statement, and cash flow statement, as well as other financial reports and documents.
How does neural network examine financial reports and understand financial state of the company?
References
- H. Khalil and J. Grizzle. Nonlinear systems, volume 3. Prentice hall Upper Saddle River, 2002.
- Mikolov T, Chen K, Corrado GS, Dean J. 2013a. Efficient estimation of word representations in vector space. arXiv:1301.3781 [cs.CL]
- Tibshirani R, Hastie T. 1987. Local likelihood estimation. J. Am. Stat. Assoc. 82:559–67
- Tibshirani R, Hastie T. 1987. Local likelihood estimation. J. Am. Stat. Assoc. 82:559–67
- Arjovsky M, Bottou L. 2017. Towards principled methods for training generative adversarial networks. arXiv:1701.04862 [stat.ML]
- Chernozhukov V, Escanciano JC, Ichimura H, Newey WK. 2016b. Locally robust semiparametric estimation. arXiv:1608.00033 [math.ST]
- Jorgenson, D.W., Weitzman, M.L., ZXhang, Y.X., Haxo, Y.M. and Mat, Y.X., 2023. S&P 500: Is the Bull Market Ready to Run Out of Steam?. AC Investment Research Journal, 220(44).