AUC Score :
Short-term Tactic1 :
Dominant Strategy :
Time series to forecast n:
ML Model Testing : Modular Neural Network (Market News Sentiment Analysis)
Hypothesis Testing : Linear Regression
Surveillance : Major exchange and OTC
1Short-term revised.
2Time series is updated based on short-term trends.
Key Points
VNT's future appears cautiously optimistic, with predictions leaning towards moderate growth driven by its focus on automotive and mobility technologies. Revenue streams from recurring software and services are anticipated to stabilize financial performance. Potential risks include economic downturns that could impact automotive sales and infrastructure spending, as well as supply chain disruptions affecting manufacturing and product delivery. Increased competition within the rapidly evolving mobility sector also presents a challenge. Further, changes in consumer preferences towards electric vehicles and alternative fuels may influence VNT's product demand, making diversification imperative for sustained success.About Vontier Corporation
Vontier, a global industrial technology company, operates across various segments, including mobility technologies, diagnostics and repair solutions, and environmental and fuel solutions. The firm designs, manufactures, and services a wide range of products and systems. These offerings are geared towards improving safety, efficiency, and sustainability for its customers in diverse industries.
The company serves a global customer base with a focus on providing essential equipment, software, and services. It is dedicated to long-term growth via innovation, strategic acquisitions, and operational excellence. Vontier aims to generate value for its shareholders by leveraging its technological expertise and market positions to capitalize on prevailing industry trends and opportunities.
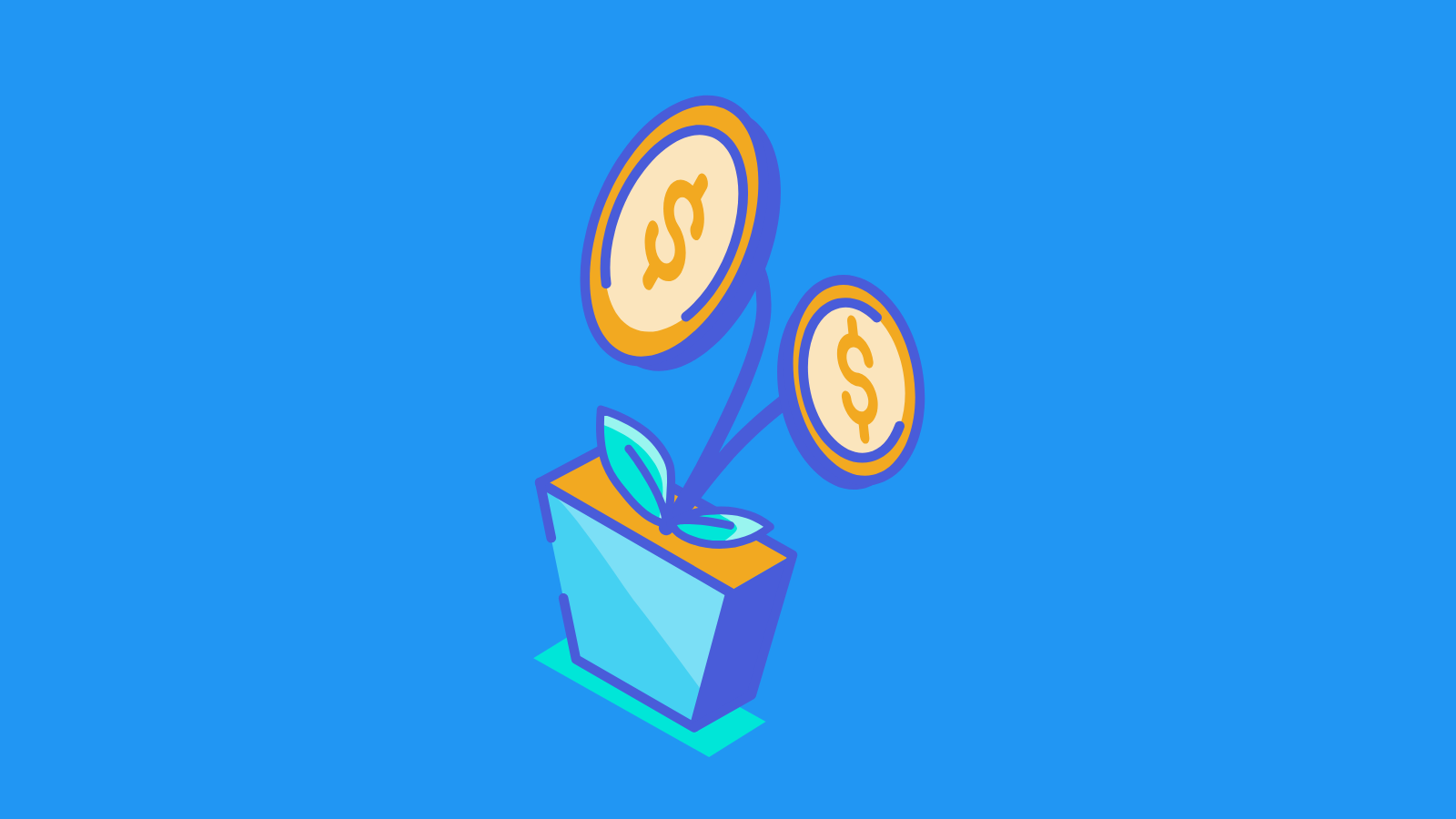
VNT Stock Forecast Machine Learning Model
Our team of data scientists and economists has developed a comprehensive machine learning model to forecast the performance of Vontier Corporation Common Stock (VNT). The model integrates a variety of data sources to capture the complex factors that influence stock movements. This encompasses both fundamental and technical indicators. Fundamental data includes key financial metrics such as revenue growth, profitability ratios (gross margin, operating margin, net margin), debt levels, and cash flow generation. These indicators provide insights into the company's underlying financial health and operational efficiency. Technical analysis incorporates historical price and volume data, along with derived technical indicators such as moving averages, Relative Strength Index (RSI), and Bollinger Bands. Economic indicators, including inflation rates, interest rates, and industry-specific performance metrics, are also integrated into the model to capture macroeconomic influences that impact Vontier's business.
The model's architecture leverages a combination of machine learning techniques to generate its forecasts. We've employed a hybrid approach, utilizing both time-series models such as Recurrent Neural Networks (RNNs), particularly LSTMs (Long Short-Term Memory), which are well-suited for capturing temporal dependencies in financial data, and ensemble methods like Random Forests and Gradient Boosting Machines to improve overall forecast accuracy and mitigate potential biases. The model is trained on a historical dataset of VNT's stock, fundamental, technical, and economic data, with the data preprocessed through standardization and normalization techniques to optimize the model's performance. The choice of model is dynamic and is subject to recalibration based on performance on an ongoing basis. It's important to note that the accuracy of such a model can vary over time.
Model performance is rigorously evaluated through out-of-sample testing and backtesting using various evaluation metrics, including Mean Absolute Error (MAE), Root Mean Squared Error (RMSE), and directional accuracy. These metrics help to measure the model's predictive power and its ability to identify buy/sell signals. The model's outputs are refined by including insights from human experts. Continuous monitoring and model retraining with updated data will be essential to maintain the model's predictive accuracy and adaptability to changing market conditions. The model's outputs, along with identified uncertainties, will be used to inform financial recommendations but should not be taken as financial advice. The forecasts generated by the model are probabilistic and do not guarantee future stock performance.
ML Model Testing
n:Time series to forecast
p:Price signals of Vontier Corporation stock
j:Nash equilibria (Neural Network)
k:Dominated move of Vontier Corporation stock holders
a:Best response for Vontier Corporation target price
For further technical information as per how our model work we invite you to visit the article below:
How do KappaSignal algorithms actually work?
Vontier Corporation Stock Forecast (Buy or Sell) Strategic Interaction Table
Strategic Interaction Table Legend:
X axis: *Likelihood% (The higher the percentage value, the more likely the event will occur.)
Y axis: *Potential Impact% (The higher the percentage value, the more likely the price will deviate.)
Z axis (Grey to Black): *Technical Analysis%
Vontier Corporation Financial Outlook and Forecast
VNT is positioned for continued growth, driven by its strategic focus on high-growth, recurring revenue streams and operational efficiencies. The company's portfolio of businesses, encompassing mobility technologies, diagnostics and repair solutions, and retail fueling infrastructure, aligns with key secular trends such as vehicle electrification, advanced driver-assistance systems (ADAS), and the increasing demand for fuel efficiency. The company's acquisition strategy has been carefully curated, focusing on businesses that complement its existing capabilities and offer opportunities for synergies and margin expansion. Furthermore, VNT's robust free cash flow generation provides it with flexibility to invest in organic growth, pursue strategic acquisitions, and return capital to shareholders. Management's disciplined approach to cost management and capital allocation further supports a positive outlook. The company has also successfully navigated macroeconomic headwinds, demonstrating resilience and agility in adapting to evolving market conditions.
The company's forecast indicates consistent revenue growth and improved profitability in the coming years. The transition towards a more service-oriented business model, supported by its diagnostic solutions and recurring software subscriptions, enhances the stability and predictability of its revenue stream. The company's investments in research and development are crucial for fostering innovation and maintaining a competitive edge in rapidly evolving markets. The company's global presence allows it to capitalize on opportunities in both developed and emerging markets, reducing its geographic concentration risk. The automotive aftermarket and fueling equipment sectors, within which VNT operates, are expected to experience sustained growth, driven by the expanding global vehicle fleet and increasing demand for efficient fueling infrastructure. VNT is expected to continue making strategic acquisitions to expand into complementary areas and drive market share gains.
Several factors underpin the favorable financial outlook for VNT. The company's focus on technology-driven solutions, which create value for customers and contribute to the sustainability of its long-term financial performance, is important. The automotive and fueling industries continue to experience technological advancements and regulatory changes, providing opportunities for VNT to capture value through innovation. The company's operational excellence initiatives, including streamlining its supply chain, improving its manufacturing processes, and enhancing its customer service capabilities, drive improvements in profitability. VNT's investments in employee training and development and its commitment to maintaining a strong corporate culture underpin its ability to attract and retain skilled professionals, further enhancing its ability to drive innovation and maintain market leadership.
Overall, the outlook for VNT is positive. The company's strategic focus, strong financial profile, and operational discipline position it well for continued success. The prediction is for **sustainable revenue growth and margin expansion, leading to increased shareholder value.** However, this forecast is subject to certain risks. Economic downturns, disruptions in global supply chains, and increased competition within the automotive and fueling industries may pose challenges. The company's success relies on the ability to successfully integrate acquired businesses, adapt to technological changes, and maintain strong relationships with customers and suppliers. Failure to effectively manage these risks could negatively impact the company's financial performance.
Rating | Short-Term | Long-Term Senior |
---|---|---|
Outlook | Baa2 | Ba3 |
Income Statement | Baa2 | Baa2 |
Balance Sheet | Baa2 | Caa2 |
Leverage Ratios | Ba3 | C |
Cash Flow | Baa2 | Baa2 |
Rates of Return and Profitability | Baa2 | Ba2 |
*Financial analysis is the process of evaluating a company's financial performance and position by neural network. It involves reviewing the company's financial statements, including the balance sheet, income statement, and cash flow statement, as well as other financial reports and documents.
How does neural network examine financial reports and understand financial state of the company?
References
- Bickel P, Klaassen C, Ritov Y, Wellner J. 1998. Efficient and Adaptive Estimation for Semiparametric Models. Berlin: Springer
- S. Bhatnagar, R. Sutton, M. Ghavamzadeh, and M. Lee. Natural actor-critic algorithms. Automatica, 45(11): 2471–2482, 2009
- Li L, Chu W, Langford J, Moon T, Wang X. 2012. An unbiased offline evaluation of contextual bandit algo- rithms with generalized linear models. In Proceedings of 4th ACM International Conference on Web Search and Data Mining, pp. 297–306. New York: ACM
- Ruiz FJ, Athey S, Blei DM. 2017. SHOPPER: a probabilistic model of consumer choice with substitutes and complements. arXiv:1711.03560 [stat.ML]
- Chernozhukov V, Chetverikov D, Demirer M, Duflo E, Hansen C, Newey W. 2017. Double/debiased/ Neyman machine learning of treatment effects. Am. Econ. Rev. 107:261–65
- D. Bertsekas and J. Tsitsiklis. Neuro-dynamic programming. Athena Scientific, 1996.
- O. Bardou, N. Frikha, and G. Pag`es. Computing VaR and CVaR using stochastic approximation and adaptive unconstrained importance sampling. Monte Carlo Methods and Applications, 15(3):173–210, 2009.