AUC Score :
Short-term Tactic1 :
Dominant Strategy :
Time series to forecast n:
ML Model Testing : Modular Neural Network (CNN Layer)
Hypothesis Testing : Paired T-Test
Surveillance : Major exchange and OTC
1Short-term revised.
2Time series is updated based on short-term trends.
Key Points
Upwork faces a future where its growth is likely to be moderately positive, driven by the increasing demand for remote work and the global expansion of freelance services. The company should see steady revenue increases as it attracts more clients and freelancers to its platform, possibly diversifying its service offerings to capture more market share. However, Upwork carries risks including intense competition from established players and emerging platforms, the potential for economic downturns to reduce client spending, and the challenges associated with maintaining platform security and trust. Furthermore, changing regulations regarding gig economy workers and labor practices could affect its business model and operating costs. Success depends on adapting to these risks while innovating its platform and expanding its global reach.About Upwork
Upwork Inc. is a global freelancing platform that connects businesses with independent professionals. The company facilitates work across various fields, including software development, creative design, writing, customer service, and finance. Upwork provides tools for project management, communication, and payment processing, streamlining the hiring and collaboration process for both clients and freelancers. Its core business model revolves around charging fees on transactions made through its platform.
The company operates in a dynamic market, benefiting from the increasing adoption of remote work and the gig economy. Upwork faces competition from other online platforms and traditional staffing agencies. The company focuses on technology upgrades, marketing and expanding its service offerings to stay ahead. It seeks to strengthen its position as a leading provider of freelance talent, supporting businesses of all sizes and contributing to the growth of the flexible workforce.
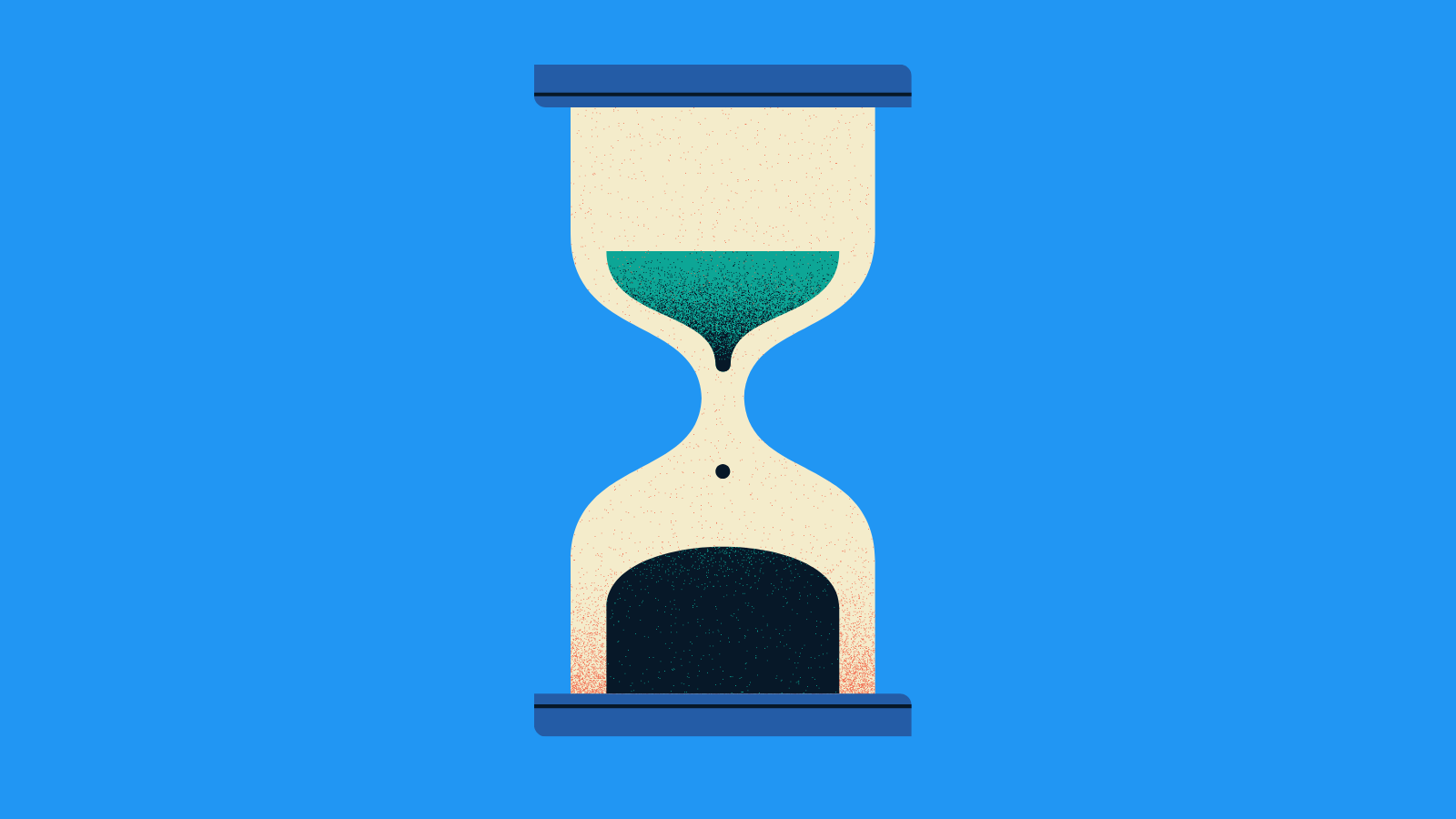
UPWK Stock Forecasting Model
Our team of data scientists and economists has developed a machine learning model to forecast the performance of Upwork Inc. (UPWK) stock. The model leverages a comprehensive set of features categorized into three main groups. First, we incorporate fundamental data, including quarterly and annual financial statements (revenue, earnings per share, profit margins, debt-to-equity ratio), key performance indicators (KPIs) such as the number of active clients and marketplace volume, and management guidance regarding future performance. Second, market data such as overall market sentiment (S&P 500 performance), sector-specific trends (technology sector movements), and trading volume of UPWK stock are included. Third, we analyze macroeconomic indicators like inflation rates, unemployment figures, and interest rate changes, given their impact on the overall economy and technology spending.
The model's architecture utilizes a combination of algorithms. A Random Forest model is employed for its ability to handle non-linear relationships and interactions between the diverse features. Time series analysis components are also incorporated, including ARIMA models, to capture the temporal dependencies inherent in stock prices. The model's parameters are tuned using a cross-validation approach to optimize predictive accuracy. We validate the model on unseen historical data to assess its performance and ensure robustness. Additionally, we incorporate regular model monitoring and re-training to ensure that it adapts to changes in market dynamics and new available data. The output of the model is a forecast of the expected trend for UPWK stock in the defined period, providing a directional signal to guide investment decisions.
Our model provides a valuable tool for analyzing UPWK stock. While the model offers predictive insights, it is crucial to understand that stock forecasting involves inherent uncertainty. The model's output must be considered alongside qualitative factors such as industry developments, competitive landscape, and company-specific news. We regularly review and refine the model, incorporating new data and feedback. The model's predictions are intended for informational purposes and should not be interpreted as financial advice. Investors must always conduct their own due diligence and consult with financial advisors before making investment decisions.
```
ML Model Testing
n:Time series to forecast
p:Price signals of Upwork stock
j:Nash equilibria (Neural Network)
k:Dominated move of Upwork stock holders
a:Best response for Upwork target price
For further technical information as per how our model work we invite you to visit the article below:
How do KappaSignal algorithms actually work?
Upwork Stock Forecast (Buy or Sell) Strategic Interaction Table
Strategic Interaction Table Legend:
X axis: *Likelihood% (The higher the percentage value, the more likely the event will occur.)
Y axis: *Potential Impact% (The higher the percentage value, the more likely the price will deviate.)
Z axis (Grey to Black): *Technical Analysis%
Upwork Inc. Common Stock Financial Outlook and Forecast
The financial outlook for Upwork (UPWK) presents a complex picture shaped by its position in the evolving freelance and remote work landscape. The company's business model, centered on connecting businesses with freelance professionals, is well-positioned to benefit from the ongoing shift towards flexible work arrangements. UPWK generates revenue through service fees charged to both clients and freelancers, creating a sustainable revenue stream. Key financial indicators to watch include gross services volume (GSV), revenue growth, and profitability metrics such as adjusted EBITDA and free cash flow. The market's valuation of UPWK reflects expectations for continued strong growth, but also accounts for the inherent volatility of the gig economy and competition from alternative platforms and internal talent solutions.
UPWK's revenue forecast hinges on several critical factors. The continued expansion of the global freelance market is a primary driver, with increasing numbers of businesses opting for flexible staffing solutions. Furthermore, the company's ability to attract and retain high-quality freelancers and clients is crucial. Investments in technology and platform improvements to enhance user experience, facilitate project matching, and streamline payment processes will be essential for sustained growth. UPWK's success also depends on its ability to effectively compete with other freelancing platforms and navigate the challenges associated with global operations, including currency fluctuations and regulatory compliance. The expansion into higher-value services, like project management tools and specialized professional skill offerings, may represent important revenue opportunities for UPWK to increase revenue per client or project.
Profitability analysis for UPWK requires consideration of the company's cost structure. Key cost components include sales and marketing expenses, research and development expenditures, and general and administrative costs. UPWK is expected to manage these costs effectively to achieve positive adjusted EBITDA margins. The company's strategic investments in marketing and sales efforts will be critical to growing user base and attracting new clients and freelancers. Cost management will be crucial to achieving profitability targets and delivering strong returns for investors. The scalability of the platform, which enables UPWK to handle increasing transaction volume with relatively small marginal costs, is another positive sign for profitability. UPWK may consider partnerships or acquisitions to increase the quality of services in the long term.
In conclusion, the financial outlook for UPWK is generally positive, fueled by the expansion of the freelance market and the company's established position as a leading platform. Revenue growth is expected to continue, driven by strong user acquisition, platform improvements, and expansion of its service offerings. The company is expected to improve its profitability with careful cost management and platform scaling. A significant risk to this positive outlook is increased competition. Rapid technological changes, potential economic downturns impacting demand for freelance services, and regulatory changes could affect UPWK's forecast. The company is expected to improve its financial position, but this outlook comes with substantial risks.
```
Rating | Short-Term | Long-Term Senior |
---|---|---|
Outlook | B1 | Ba3 |
Income Statement | Ba3 | Caa2 |
Balance Sheet | Baa2 | Ba3 |
Leverage Ratios | B2 | Baa2 |
Cash Flow | C | Baa2 |
Rates of Return and Profitability | Ba3 | Caa2 |
*Financial analysis is the process of evaluating a company's financial performance and position by neural network. It involves reviewing the company's financial statements, including the balance sheet, income statement, and cash flow statement, as well as other financial reports and documents.
How does neural network examine financial reports and understand financial state of the company?
References
- E. van der Pol and F. A. Oliehoek. Coordinated deep reinforcement learners for traffic light control. NIPS Workshop on Learning, Inference and Control of Multi-Agent Systems, 2016.
- Thompson WR. 1933. On the likelihood that one unknown probability exceeds another in view of the evidence of two samples. Biometrika 25:285–94
- Athey S, Bayati M, Imbens G, Zhaonan Q. 2019. Ensemble methods for causal effects in panel data settings. NBER Work. Pap. 25675
- Ashley, R. (1988), "On the relative worth of recent macroeconomic forecasts," International Journal of Forecasting, 4, 363–376.
- Jorgenson, D.W., Weitzman, M.L., ZXhang, Y.X., Haxo, Y.M. and Mat, Y.X., 2023. Google's Stock Price Set to Soar in the Next 3 Months. AC Investment Research Journal, 220(44).
- K. Boda, J. Filar, Y. Lin, and L. Spanjers. Stochastic target hitting time and the problem of early retirement. Automatic Control, IEEE Transactions on, 49(3):409–419, 2004
- Z. Wang, T. Schaul, M. Hessel, H. van Hasselt, M. Lanctot, and N. de Freitas. Dueling network architectures for deep reinforcement learning. In Proceedings of the International Conference on Machine Learning (ICML), pages 1995–2003, 2016.