AUC Score :
Short-term Tactic1 :
Dominant Strategy :
Time series to forecast n:
ML Model Testing : Deductive Inference (ML)
Hypothesis Testing : Multiple Regression
Surveillance : Major exchange and OTC
1Short-term revised.
2Time series is updated based on short-term trends.
Key Points
A surge in the TR/CC CRB Heating Oil index is anticipated, fueled by strong seasonal demand coupled with potential supply disruptions stemming from geopolitical tensions and weather-related events. Conversely, the index faces a risk of decline if there's weakening economic activity that suppresses energy consumption, or if significant production increases emerge from major oil-producing regions, which could overwhelm demand. Unexpected mild weather is also a bearish factor.About TR/CC CRB Heating Oil Index
The TR/CC CRB Heating Oil index is a component of the broader Thomson Reuters/CoreCommodity CRB Index, a benchmark widely used to track the price movements of various commodity futures contracts. This sub-index specifically focuses on the heating oil market, reflecting the price fluctuations of heating oil futures traded on major commodity exchanges. As part of the larger CRB Index, it offers investors and analysts a means of gauging the performance of heating oil relative to other commodities and the overall commodities market. The heating oil market is vital as it is used for heating and power generation.
The TR/CC CRB Heating Oil index serves as a valuable tool for understanding trends and volatility within the heating oil sector. It provides information for those monitoring the energy market, analyzing potential investment opportunities, or managing risk in their portfolios. Its composition and methodology are subject to updates and revisions by Thomson Reuters to maintain accuracy and reflect evolving market dynamics. The index is tracked closely by traders, energy companies, and financial institutions seeking to understand the energy sector.
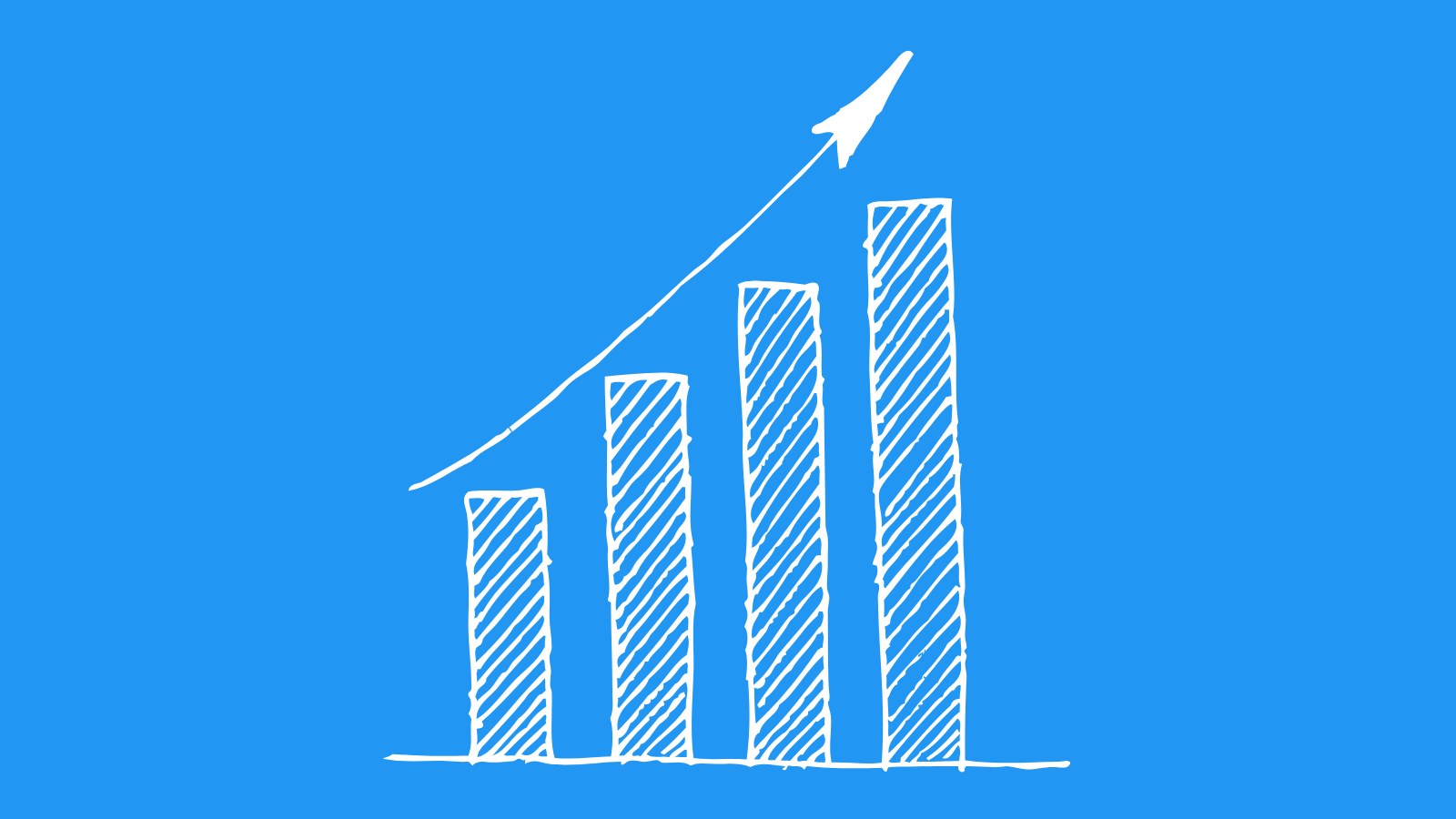
Machine Learning Model for TR/CC CRB Heating Oil Index Forecast
Our objective is to develop a robust machine learning model for forecasting the TR/CC CRB Heating Oil index. The forecasting process will leverage a diverse set of predictive variables encompassing macroeconomic indicators, supply-demand dynamics, and sentiment analysis. Key macroeconomic indicators include inflation rates, interest rates (such as the US Federal Funds Rate), and GDP growth forecasts from major economies like the United States, China, and the Eurozone. These indicators provide insights into overall economic activity, which in turn affects energy consumption. Furthermore, we will incorporate data on oil production from major producers (OPEC, Russia, and the US), refining capacity utilization, and inventory levels from organizations like the Energy Information Administration (EIA) to capture the supply-side factors influencing heating oil prices. Sentiment analysis will be implemented using natural language processing (NLP) techniques to analyze news articles and social media discussions related to the oil market.
The core of our forecasting model will employ a combination of machine learning algorithms. Initially, we will explore the application of time series models such as ARIMA and its variants, incorporating exogenous variables (ARIMAX) to account for the impact of external factors. We will also experiment with more sophisticated techniques, including Recurrent Neural Networks (RNNs), specifically Long Short-Term Memory (LSTM) networks, designed to handle sequential data and capture temporal dependencies in the price movements. These models can learn complex patterns and non-linear relationships. Additionally, we plan to investigate ensemble methods, such as Random Forests and Gradient Boosting, which combine the predictions of multiple models to improve overall accuracy and robustness. Feature engineering will be a crucial step, including the creation of lagged variables for both the heating oil price itself and the selected predictors to capture trends, seasonality, and cyclical behavior. We will also explore techniques like rolling averages and volatility calculations.
The model's performance will be assessed rigorously using a variety of metrics, including Mean Absolute Error (MAE), Mean Squared Error (MSE), and Root Mean Squared Error (RMSE). We will also utilize the R-squared statistic to evaluate the model's explanatory power. The dataset will be split into training, validation, and test sets to ensure proper model evaluation and prevent overfitting. The model parameters and hyperparameters will be carefully tuned using techniques like cross-validation. Regular model updates and retraining will be performed as new data becomes available to maintain forecasting accuracy over time. Model interpretability is important; therefore, we will apply techniques to understand the importance of each input feature, enabling us to offer insights into the factors that most significantly drive heating oil price fluctuations and support informed decision-making.
ML Model Testing
n:Time series to forecast
p:Price signals of TR/CC CRB Heating Oil index
j:Nash equilibria (Neural Network)
k:Dominated move of TR/CC CRB Heating Oil index holders
a:Best response for TR/CC CRB Heating Oil target price
For further technical information as per how our model work we invite you to visit the article below:
How do KappaSignal algorithms actually work?
TR/CC CRB Heating Oil Index Forecast Strategic Interaction Table
Strategic Interaction Table Legend:
X axis: *Likelihood% (The higher the percentage value, the more likely the event will occur.)
Y axis: *Potential Impact% (The higher the percentage value, the more likely the price will deviate.)
Z axis (Grey to Black): *Technical Analysis%
Financial Outlook and Forecast for TR/CC CRB Heating Oil
The TR/CC CRB Heating Oil index, representing the price of heating oil, is intrinsically linked to a confluence of factors impacting global energy markets. Demand, supply dynamics, geopolitical events, and weather patterns all play critical roles in shaping its trajectory. Increased demand during colder months, driven by heating needs in residential, commercial, and industrial sectors, often puts upward pressure on prices. Conversely, ample supply, particularly from major oil-producing nations or increased refinery output, can contribute to price stabilization or downward trends. Furthermore, the index's volatility is often amplified by unexpected disruptions such as severe weather events impacting infrastructure, geopolitical tensions influencing production and distribution, and shifts in global economic activity which affects overall energy consumption. Understanding these multifaceted variables is crucial for assessing the index's financial outlook.
Supply-side considerations carry significant weight in forecasting. OPEC and its allies (OPEC+) actions are key. Production decisions, adherence to output quotas, and the potential for future production cuts or increases significantly impact the heating oil supply. Geopolitical instability in oil-producing regions is another area of concern. Conflicts, sanctions, or political unrest can disrupt production and transportation, leading to price spikes. Investment in refining capacity also plays a crucial role. Limited refining capacity can strain supply during peak demand, resulting in higher heating oil prices. Conversely, increased refining capacity and efficiency improvements can alleviate supply constraints and moderate price increases. Furthermore, the emergence of alternative energy sources and government policies aimed at transitioning away from fossil fuels introduce long-term uncertainties to the heating oil market.
Demand-side influences also have a considerable effect on the index's performance. Economic activity, particularly in the industrial sector, is a primary driver of heating oil consumption. A robust global economy generally leads to increased demand, while economic downturns can depress consumption. Weather patterns significantly affect seasonal demand, with colder winters typically leading to higher prices and warmer winters contributing to price decreases. Inventory levels are a crucial market indicator. High inventory levels can signal a potential surplus and downward pressure on prices, while low inventories can suggest a potential supply shortage and upward pressure. Furthermore, government regulations and incentives that promote energy efficiency or the adoption of alternative heating fuels can impact long-term heating oil demand, creating structural changes in the market.
Overall, the outlook for the TR/CC CRB Heating Oil index is cautiously positive. While near-term volatility is expected due to unforeseen circumstances such as geopolitical shocks or unpredictable weather, the underlying trend suggests a gradual increase in prices over the coming years, especially during peak demand seasons. This forecast is contingent on continued moderate global economic growth and limited expansions in refining capacity. The primary risks to this prediction include unexpectedly severe economic downturns, geopolitical instability leading to supply disruptions, significant advancements in alternative heating technologies that erode demand, and unexpected increases in production by OPEC+. Investors and market participants must closely monitor these variables and adapt their strategies accordingly, recognizing the inherent uncertainties and potential for significant price fluctuations within the heating oil market.
Rating | Short-Term | Long-Term Senior |
---|---|---|
Outlook | Ba3 | B3 |
Income Statement | Baa2 | C |
Balance Sheet | C | Caa2 |
Leverage Ratios | B2 | B3 |
Cash Flow | Baa2 | Baa2 |
Rates of Return and Profitability | Baa2 | C |
*An aggregate rating for an index summarizes the overall sentiment towards the companies it includes. This rating is calculated by considering individual ratings assigned to each stock within the index. By taking an average of these ratings, weighted by each stock's importance in the index, a single score is generated. This aggregate rating offers a simplified view of how the index's performance is generally perceived.
How does neural network examine financial reports and understand financial state of the company?
References
- Breiman L. 2001a. Random forests. Mach. Learn. 45:5–32
- Hill JL. 2011. Bayesian nonparametric modeling for causal inference. J. Comput. Graph. Stat. 20:217–40
- Clements, M. P. D. F. Hendry (1995), "Forecasting in cointegrated systems," Journal of Applied Econometrics, 10, 127–146.
- Hastie T, Tibshirani R, Friedman J. 2009. The Elements of Statistical Learning. Berlin: Springer
- M. Sobel. The variance of discounted Markov decision processes. Applied Probability, pages 794–802, 1982
- Pennington J, Socher R, Manning CD. 2014. GloVe: global vectors for word representation. In Proceedings of the 2014 Conference on Empirical Methods on Natural Language Processing, pp. 1532–43. New York: Assoc. Comput. Linguist.
- Arora S, Li Y, Liang Y, Ma T. 2016. RAND-WALK: a latent variable model approach to word embeddings. Trans. Assoc. Comput. Linguist. 4:385–99