AUC Score :
Short-term Tactic1 :
Dominant Strategy :
Time series to forecast n:
ML Model Testing : Statistical Inference (ML)
Hypothesis Testing : Stepwise Regression
Surveillance : Major exchange and OTC
1Short-term revised.
2Time series is updated based on short-term trends.
Key Points
The TR/CC CRB ex Energy TR Index is anticipated to exhibit moderate volatility. We predict a modest upward trajectory, driven by ongoing global demand, supply chain dynamics and geopolitical influences, particularly impacting agricultural commodities and industrial metals. However, this outlook is tempered by risks. Economic slowdowns in major consuming nations, increased production from key suppliers, and unforeseen weather events could exert downward pressure on index values. The index is also susceptible to fluctuations in currency exchange rates, which can impact the competitiveness of commodities. Furthermore, supply disruptions and heightened inflation pose considerable challenges.About TR/CC CRB ex Energy TR Index
The TR/CC CRB ex Energy TR index, administered by Thomson Reuters and the Commodity Research Bureau (CRB), is a benchmark designed to track the price movements of a broad basket of commodities. It is a total return index, meaning it incorporates the effects of both price changes and any income generated from holding the underlying commodity futures contracts. The "ex Energy" designation signifies that the index excludes energy-related commodities, such as crude oil and natural gas, to offer investors a more diversified exposure to non-energy commodity markets.
This index provides a focused view on a selection of essential industrial commodities. It is widely used as a performance indicator for commodity investments, enabling investors to gauge the relative performance of non-energy commodity assets. Its construction aims to reflect the economic significance of these commodities, and the weighting methodology may be based on factors like trading volume and production levels. This makes it a useful tool for those seeking exposure to the global commodity market, excluding the often volatile energy sector.
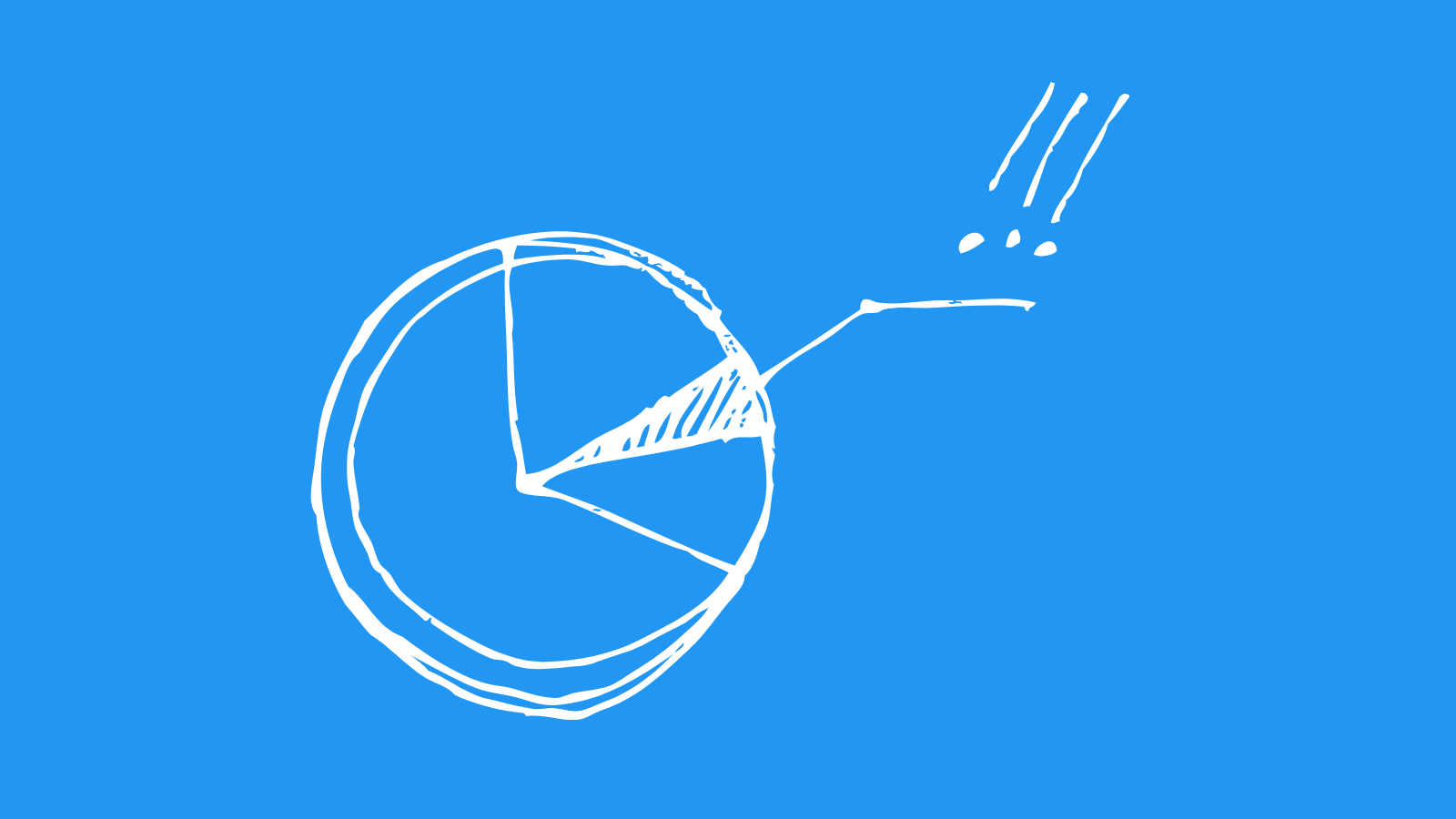
TR/CC CRB ex Energy TR Index Forecast Model
Our team of data scientists and economists has developed a machine learning model to forecast the TR/CC CRB ex Energy TR index. The core of our approach lies in utilizing a comprehensive dataset spanning various macroeconomic indicators, including inflation rates, interest rates (such as the federal funds rate and bond yields), industrial production figures, and global trade data, alongside commodity-specific information like supply chain disruptions and weather patterns. We will also integrate sentiment analysis from financial news articles and social media to capture market perceptions and expectations. The model will employ a combination of time series analysis (like ARIMA and its variations to capture autoregressive dependencies) and machine learning techniques (such as Random Forest and Gradient Boosting) to identify non-linear relationships within the data. Feature engineering will be critical; we will create lagged variables, moving averages, and other transformations to enhance predictive power. Model performance will be continuously evaluated through metrics such as Mean Absolute Error (MAE), Root Mean Squared Error (RMSE), and R-squared, using a holdout validation set to ensure robust out-of-sample accuracy. This rigorous approach ensures that the model accurately predicts the index's movement.
The model architecture will prioritize interpretability. While complex machine learning algorithms can be effective, understanding the drivers behind the forecasts is crucial for informed decision-making. Thus, we'll incorporate feature importance analysis from Random Forest and Gradient Boosting to identify the most influential predictors. Furthermore, we'll perform sensitivity analysis by varying key input variables to assess their impact on the forecast. To mitigate overfitting, we will employ cross-validation techniques and regularize the machine learning algorithms. The model will be regularly retrained and updated with fresh data to account for evolving market dynamics. Model output will include not only a point forecast but also a confidence interval, reflecting the uncertainty inherent in commodity markets. We will assess the model's robustness by checking extreme events during validation.
The model will be implemented to provide timely forecasts for the TR/CC CRB ex Energy TR index. The output will be tailored for both short-term (e.g., daily or weekly) and medium-term (e.g., monthly or quarterly) forecasts, based on the model's performance in different time horizons. The forecasts and confidence intervals, and also the explanations that drive the forecast will be communicated through interactive dashboards and reports, enabling stakeholders to track the index's anticipated behavior and plan investments. Our team will also conduct periodic model reviews to assess the model's efficacy and address potential biases or limitations. This ensures a reliable and actionable forecast for investors, traders, and policymakers. We ensure that the model stays updated with the most recent information on the index.
ML Model Testing
n:Time series to forecast
p:Price signals of TR/CC CRB ex Energy TR index
j:Nash equilibria (Neural Network)
k:Dominated move of TR/CC CRB ex Energy TR index holders
a:Best response for TR/CC CRB ex Energy TR target price
For further technical information as per how our model work we invite you to visit the article below:
How do KappaSignal algorithms actually work?
TR/CC CRB ex Energy TR Index Forecast Strategic Interaction Table
Strategic Interaction Table Legend:
X axis: *Likelihood% (The higher the percentage value, the more likely the event will occur.)
Y axis: *Potential Impact% (The higher the percentage value, the more likely the price will deviate.)
Z axis (Grey to Black): *Technical Analysis%
TR/CC CRB ex Energy TR Index: Financial Outlook and Forecast
The TR/CC CRB ex Energy TR Index, a commodity benchmark that excludes the energy sector, presents a nuanced financial outlook. The index's performance is intricately linked to global economic growth, supply chain dynamics, and geopolitical events. Demand from emerging markets, particularly China and India, remains a significant driver, influencing the prices of industrial metals, agricultural commodities, and precious metals included within the index. Changes in monetary policy, such as interest rate adjustments by major central banks, play a crucial role in shaping investor sentiment and influencing capital flows towards or away from commodity markets. Moreover, seasonal factors impacting agricultural production and unforeseen disruptions like geopolitical instability or natural disasters can inject volatility into the index.
Analyzing the key components reveals additional insights. Industrial metals, such as copper and aluminum, often reflect the health of global manufacturing, while agricultural commodities like wheat and corn are strongly influenced by weather patterns and harvests. The inclusion of precious metals, particularly gold and silver, introduces a safe-haven element, reacting to economic uncertainty and inflation expectations. Furthermore, the relative weightings of each commodity within the index impact its overall performance. The fluctuations in demand and supply dynamics for each commodity have a significant impact on the overall price level of TR/CC CRB ex Energy TR. The ability to effectively hedge and forecast these fluctuations and dynamics becomes critical for informed investment and risk management strategies related to the index.
Recent trends suggest a mixed outlook for the TR/CC CRB ex Energy TR Index. Robust demand from emerging markets, combined with potential supply disruptions in some key commodities, could support price increases. However, concerns about economic slowdown in developed economies and the potential for increased interest rates may act as a headwind. Supply-side factors like production capacity and infrastructure developments will continue to affect prices. Global efforts toward decarbonization and investments in renewable energy projects could boost the demand for specific commodities, especially industrial metals used in infrastructure and electric vehicles. These considerations will need close monitoring to understand the price evolution and the effects on the index in the future.
Overall, a moderately positive outlook is anticipated for the TR/CC CRB ex Energy TR Index over the medium term, provided that global economic growth remains resilient. The sustained demand from emerging economies and the increasing investment in green energy solutions are expected to provide support for the index's constituents. However, this prediction is subject to several key risks. A sharper-than-expected economic slowdown in developed markets could dampen demand and pressure prices. Geopolitical tensions, natural disasters, or supply chain disruptions could also lead to volatility and unpredictable price movements. Further, changes in the strength of the U.S. dollar may impact the index, as commodities are typically priced in US dollars. Thus, careful monitoring of macroeconomic indicators, geopolitical events, and supply-demand dynamics is essential for investors and stakeholders with a vested interest in the index.
Rating | Short-Term | Long-Term Senior |
---|---|---|
Outlook | B1 | B2 |
Income Statement | C | Caa2 |
Balance Sheet | Baa2 | B2 |
Leverage Ratios | Ba1 | C |
Cash Flow | Baa2 | Ba1 |
Rates of Return and Profitability | Caa2 | B2 |
*An aggregate rating for an index summarizes the overall sentiment towards the companies it includes. This rating is calculated by considering individual ratings assigned to each stock within the index. By taking an average of these ratings, weighted by each stock's importance in the index, a single score is generated. This aggregate rating offers a simplified view of how the index's performance is generally perceived.
How does neural network examine financial reports and understand financial state of the company?
References
- A. Y. Ng, D. Harada, and S. J. Russell. Policy invariance under reward transformations: Theory and application to reward shaping. In Proceedings of the Sixteenth International Conference on Machine Learning (ICML 1999), Bled, Slovenia, June 27 - 30, 1999, pages 278–287, 1999.
- LeCun Y, Bengio Y, Hinton G. 2015. Deep learning. Nature 521:436–44
- L. Prashanth and M. Ghavamzadeh. Actor-critic algorithms for risk-sensitive MDPs. In Proceedings of Advances in Neural Information Processing Systems 26, pages 252–260, 2013.
- J. N. Foerster, Y. M. Assael, N. de Freitas, and S. Whiteson. Learning to communicate with deep multi-agent reinforcement learning. In Advances in Neural Information Processing Systems 29: Annual Conference on Neural Information Processing Systems 2016, December 5-10, 2016, Barcelona, Spain, pages 2137–2145, 2016.
- Burgess, D. F. (1975), "Duality theory and pitfalls in the specification of technologies," Journal of Econometrics, 3, 105–121.
- V. Borkar. Q-learning for risk-sensitive control. Mathematics of Operations Research, 27:294–311, 2002.
- Belloni A, Chernozhukov V, Hansen C. 2014. High-dimensional methods and inference on structural and treatment effects. J. Econ. Perspect. 28:29–50