AUC Score :
Short-term Tactic1 :
Dominant Strategy :
Time series to forecast n:
ML Model Testing : Modular Neural Network (Market Volatility Analysis)
Hypothesis Testing : Lasso Regression
Surveillance : Major exchange and OTC
1Short-term revised.
2Time series is updated based on short-term trends.
Key Points
The TR/CC CRB Aluminum index is likely to experience moderate volatility. Expect a possible slight upward trend due to increasing demand from the construction and automotive sectors coupled with potential supply chain disruptions. However, downside risks exist, including slower-than-anticipated global economic growth that could curb demand and the impact of evolving trade policies which could affect supply dynamics and pricing negatively.About TR/CC CRB Aluminum Index
The TR/CC CRB Aluminum index is a commodity index that tracks the price performance of aluminum, a key industrial metal. It serves as a benchmark for the aluminum market, providing insights into price fluctuations and overall market trends. The index is part of the broader TR/CC CRB Commodity Index family, which is a globally recognized gauge of commodity price movements. It's designed to reflect the price dynamics of physical aluminum as traded on major exchanges and through other relevant market mechanisms.
As an index, the TR/CC CRB Aluminum is typically used by investors, analysts, and traders as a tool for monitoring and analyzing the aluminum market. The index's composition and methodology are carefully designed to accurately reflect the price behavior of aluminum. The data generated by the index offers insights into supply and demand dynamics, economic conditions, and geopolitical factors that affect aluminum pricing. Consequently, it aids in risk management, portfolio construction, and investment strategy development for those involved in the aluminum market or those with a vested interest in the broader commodity landscape.
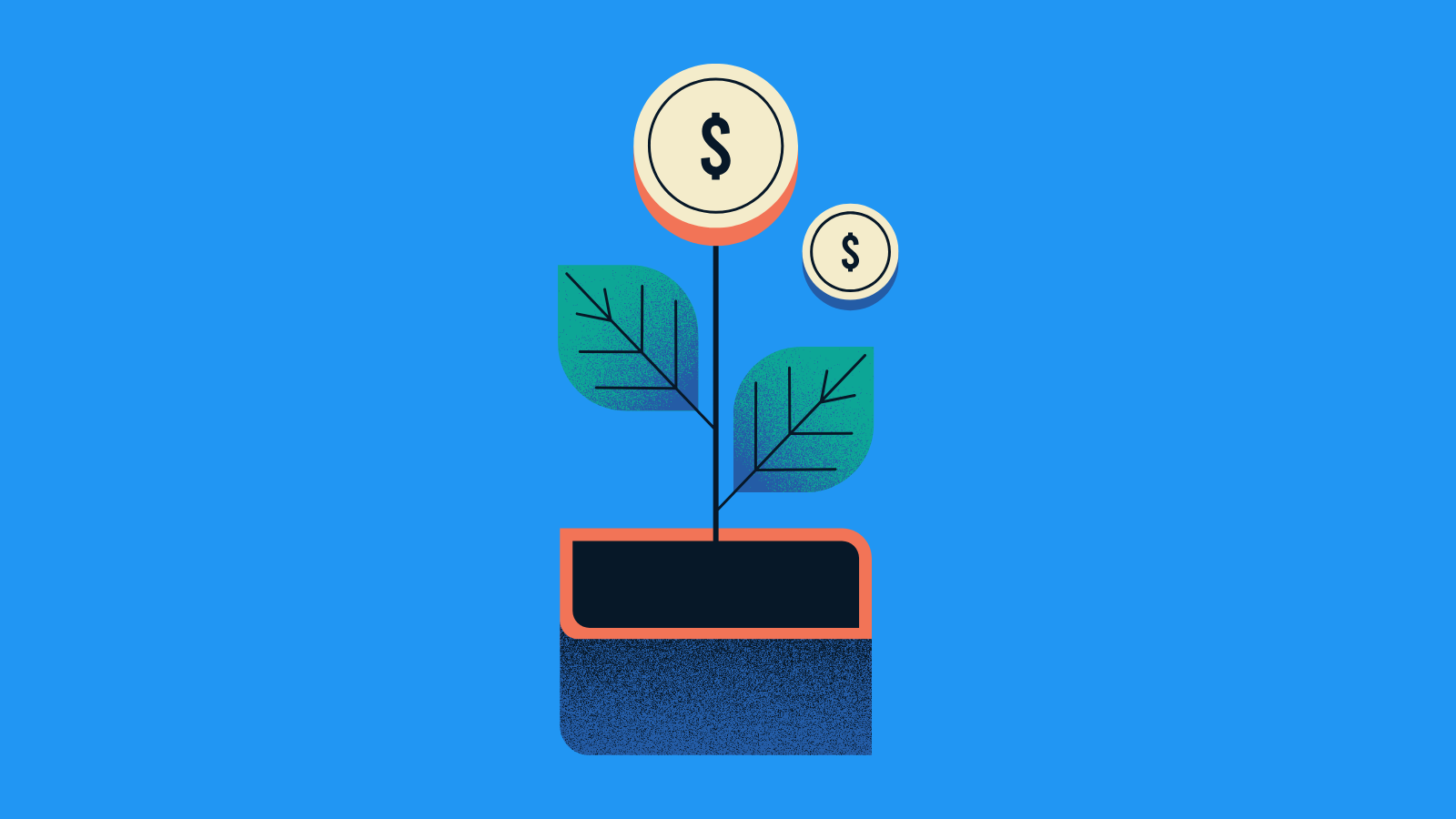
Machine Learning Model for TR/CC CRB Aluminum Index Forecast
Our team of data scientists and economists proposes a comprehensive machine learning model for forecasting the TR/CC CRB Aluminum index. The model's architecture begins with meticulous data preparation. We will gather historical data from various reliable sources, including the TR/CC CRB Aluminum index itself, macroeconomic indicators like global GDP growth, industrial production indices, and inflation rates. Furthermore, we will incorporate industry-specific data such as global aluminum demand and supply dynamics, inventory levels, and relevant exchange rates. The collected data will undergo rigorous cleaning and preprocessing, handling missing values, outliers, and ensuring data consistency. Feature engineering will be critical. We will create lagged features, moving averages, and other derived variables to capture temporal dependencies and reveal underlying trends within the dataset. The selection of features will be based on both statistical significance and domain expertise.
The core of our model will employ an ensemble of machine learning algorithms, including time-series specific models and regression techniques. We plan to experiment with Recurrent Neural Networks (RNNs), particularly Long Short-Term Memory (LSTM) networks, to capture complex temporal patterns in the index data. Additionally, we will explore Gradient Boosting Machines (GBMs) and Random Forests, known for their ability to handle non-linear relationships and provide robust predictions. To enhance the model's performance, we will implement a hybrid approach that integrates predictions from different models and weights their contributions based on historical accuracy. Hyperparameter tuning will be performed using cross-validation techniques to optimize model parameters and prevent overfitting. Furthermore, we will conduct rigorous backtesting using historical data to assess the model's predictive performance and identify potential biases.
The model's output will provide predicted values for the TR/CC CRB Aluminum index, along with confidence intervals to reflect the uncertainty in the forecasts. We will develop a user-friendly interface for visualizing the predictions and analyzing the key drivers behind the index movements. The model will be regularly updated with new data to maintain its predictive accuracy. Furthermore, we will continuously monitor and refine the model by evaluating the performance and incorporating feedback from our team of economists and data scientists. This iterative process will enable us to adapt the model to changing market conditions and provide the most reliable forecasts possible, ultimately supporting informed decision-making for stakeholders involved in the aluminum market.
ML Model Testing
n:Time series to forecast
p:Price signals of TR/CC CRB Aluminum index
j:Nash equilibria (Neural Network)
k:Dominated move of TR/CC CRB Aluminum index holders
a:Best response for TR/CC CRB Aluminum target price
For further technical information as per how our model work we invite you to visit the article below:
How do KappaSignal algorithms actually work?
TR/CC CRB Aluminum Index Forecast Strategic Interaction Table
Strategic Interaction Table Legend:
X axis: *Likelihood% (The higher the percentage value, the more likely the event will occur.)
Y axis: *Potential Impact% (The higher the percentage value, the more likely the price will deviate.)
Z axis (Grey to Black): *Technical Analysis%
TR/CC CRB Aluminum Index: Financial Outlook and Forecast
The TR/CC CRB Aluminum Index, a benchmark reflecting the price fluctuations of aluminum within the broader Thomson Reuters/CoreCommodity CRB Index, provides significant insights into the health of the global industrial sector. Analyzing the financial outlook for this index requires evaluating the interplay of supply and demand dynamics, global economic growth, and geopolitical factors. Demand is primarily driven by the automotive, aerospace, construction, and packaging industries. A robust global economy, particularly in emerging markets, typically translates into higher demand for aluminum, while any slowdown in these sectors could lead to reduced demand. On the supply side, factors such as production costs (including energy prices and bauxite availability), capacity utilization rates of aluminum smelters, and trade policies (such as tariffs and sanctions) play crucial roles. Investors and analysts closely monitor these elements to anticipate potential shifts in the index's value.
Understanding the forces impacting the aluminum market is crucial. China's role as the dominant producer and consumer significantly influences global aluminum prices. Any changes to its production policies, environmental regulations, or economic growth trajectory have a profound impact. Similarly, energy prices, a major component of aluminum smelting costs, have a direct effect on profitability. Higher energy costs can squeeze profit margins for producers, potentially leading to reduced production or higher aluminum prices to offset these costs. Furthermore, geopolitical events, such as trade disputes or supply disruptions from major aluminum-producing nations, can create volatility in the market. Economic indicators like manufacturing Purchasing Managers' Indexes (PMIs), construction starts, and automotive sales provide important signals about the demand outlook and the potential trajectory of the index. Furthermore, investment in aluminum infrastructure and the exploration of new aluminum-using technologies also influences the industry.
Key influencing factors are currently intertwined. A moderate slowdown in some major economies might curb the demand in the short term. However, the overall trajectory of the aluminum market depends heavily on factors beyond immediate demand, like the ongoing transition towards greener technologies, for which aluminum is essential. Supply chain disruptions, driven by international trade tensions and other global events, can also dramatically impact the index. The availability of bauxite, the ore from which aluminum is made, and the logistical aspects of its refining into the finished metal are critical factors. The cost and availability of electricity, particularly in the major smelting regions, are essential drivers of production costs.
The forecast for the TR/CC CRB Aluminum Index is currently cautiously optimistic. The underlying factors suggest a moderate increase over the next 12-18 months, supported by the long-term trend towards electric vehicles and increased use in construction. However, the risks to this prediction include a sharper-than-expected global economic downturn, which could depress demand. Moreover, any unforeseen supply chain disruptions or an escalation in trade tensions could significantly impact the index's value. The biggest risk is the possibility of increased competition from alternative materials like steel or carbon fiber for various industrial applications. The market's performance is dependent on the balance of supply and demand. Therefore, constant monitoring of demand dynamics, geopolitical developments, and technological advancements is critical to assess the true potential of aluminum.
```
Rating | Short-Term | Long-Term Senior |
---|---|---|
Outlook | B3 | Ba2 |
Income Statement | C | Baa2 |
Balance Sheet | C | Baa2 |
Leverage Ratios | B3 | Caa2 |
Cash Flow | B3 | B1 |
Rates of Return and Profitability | Ba3 | B3 |
*An aggregate rating for an index summarizes the overall sentiment towards the companies it includes. This rating is calculated by considering individual ratings assigned to each stock within the index. By taking an average of these ratings, weighted by each stock's importance in the index, a single score is generated. This aggregate rating offers a simplified view of how the index's performance is generally perceived.
How does neural network examine financial reports and understand financial state of the company?
References
- P. Artzner, F. Delbaen, J. Eber, and D. Heath. Coherent measures of risk. Journal of Mathematical Finance, 9(3):203–228, 1999
- Arora S, Li Y, Liang Y, Ma T. 2016. RAND-WALK: a latent variable model approach to word embeddings. Trans. Assoc. Comput. Linguist. 4:385–99
- Athey S, Wager S. 2017. Efficient policy learning. arXiv:1702.02896 [math.ST]
- Künzel S, Sekhon J, Bickel P, Yu B. 2017. Meta-learners for estimating heterogeneous treatment effects using machine learning. arXiv:1706.03461 [math.ST]
- D. Bertsekas. Dynamic programming and optimal control. Athena Scientific, 1995.
- Hirano K, Porter JR. 2009. Asymptotics for statistical treatment rules. Econometrica 77:1683–701
- Chernozhukov V, Demirer M, Duflo E, Fernandez-Val I. 2018b. Generic machine learning inference on heteroge- nous treatment effects in randomized experiments. NBER Work. Pap. 24678