AUC Score :
Short-term Tactic1 :
Dominant Strategy :
Time series to forecast n:
ML Model Testing : Multi-Task Learning (ML)
Hypothesis Testing : Stepwise Regression
Surveillance : Major exchange and OTC
1Short-term revised.
2Time series is updated based on short-term trends.
Key Points
The Dow Jones U.S. Technology Index is anticipated to experience moderate growth, driven by continued innovation in artificial intelligence, cloud computing, and cybersecurity. Demand for tech products and services is likely to remain robust, supporting the index's positive trajectory. However, there are inherent risks: Geopolitical tensions, particularly trade disputes, could disrupt supply chains and negatively impact tech companies with significant international exposure. Moreover, increased regulatory scrutiny of tech giants and potential antitrust actions pose a threat, and the market could correct if there is a broader economic downturn. Rapid technological advancements also bring the risk of obsolescence for some companies, and intense competition could squeeze profit margins for others.About Dow Jones U.S. Technology Index
The Dow Jones U.S. Technology Index is a stock market index that tracks the performance of companies considered to be technology-focused within the United States. This index includes a diverse range of businesses involved in software, hardware, semiconductors, internet services, and other related sectors. It serves as a benchmark for the technology sector, providing a snapshot of its overall health and growth. Companies are selected and weighted within the index based on factors such as market capitalization and other financial metrics.
The Dow Jones U.S. Technology Index is utilized by investors, analysts, and fund managers to gauge the performance of technology investments and compare them to broader market trends. Its fluctuations are influenced by technological advancements, economic conditions, and investor sentiment surrounding the sector. The index's composition is subject to periodic review, with additions and deletions of companies as market dynamics evolve. Consequently, this index offers insights into the leading technology companies in the United States.
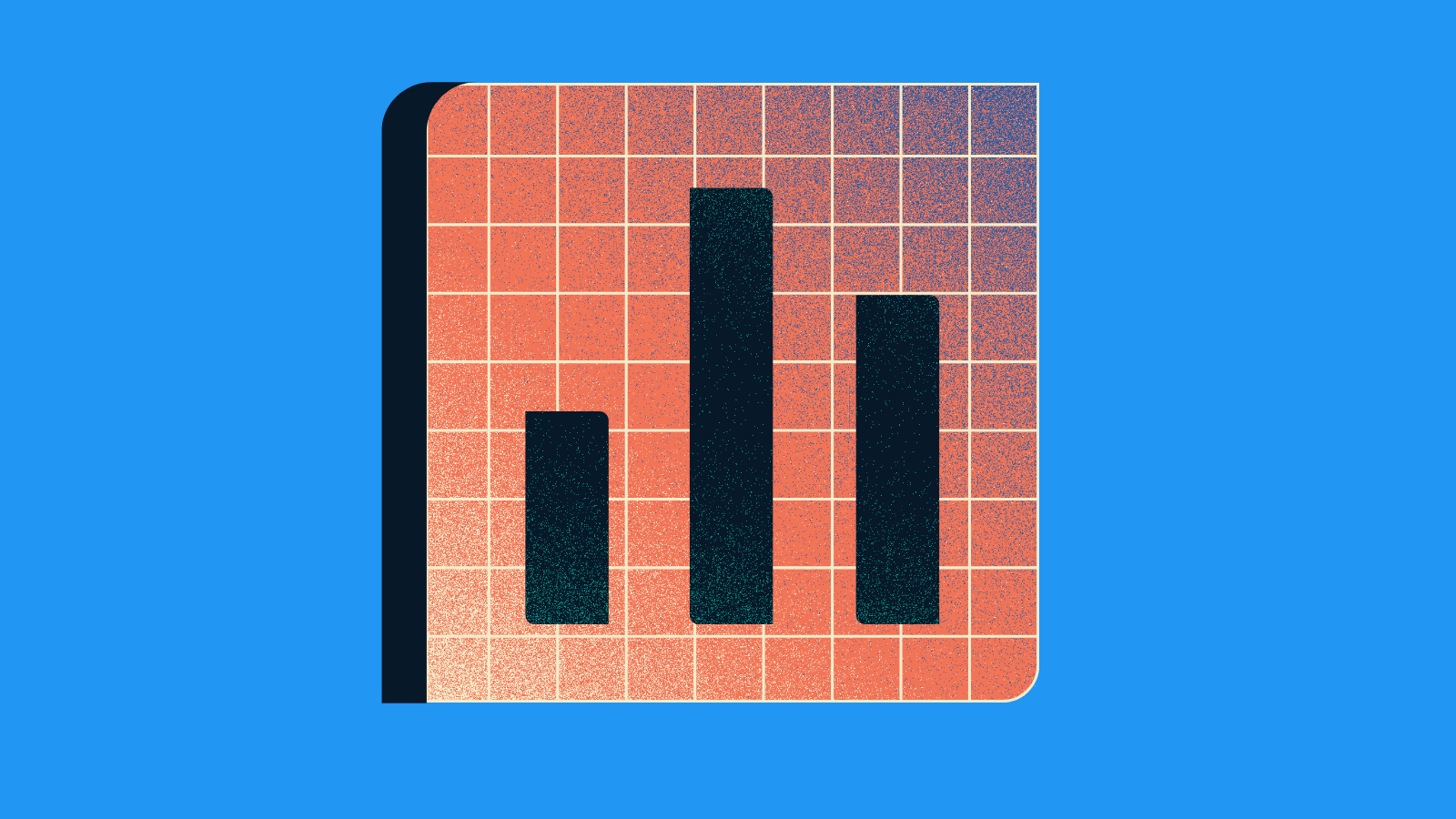
Machine Learning Model for Dow Jones U.S. Technology Index Forecast
Our team of data scientists and economists proposes a machine learning model to forecast the Dow Jones U.S. Technology Index. The core of our approach involves employing a **hybrid model** combining the strengths of both time series analysis and machine learning techniques. Specifically, we plan to utilize a **Recurrent Neural Network (RNN), particularly a Long Short-Term Memory (LSTM) network**, due to its ability to effectively capture long-term dependencies inherent in financial time series data. This will be complemented by incorporating exogenous variables derived from economic indicators, such as consumer confidence, inflation rates, interest rates, and sector-specific economic data related to the technology industry. These variables will serve as inputs to the model, enabling it to consider macroeconomic factors influencing the index's performance. Feature engineering will be crucial, involving techniques like rolling window statistics and transformations, to enrich the model's understanding of the data's temporal characteristics.
The model will be trained on a comprehensive historical dataset of the Dow Jones U.S. Technology Index, spanning at least a decade, along with corresponding data for all selected macroeconomic indicators. We will employ a **train-validation-test split** approach to rigorously evaluate the model's performance. The training phase will involve optimizing the LSTM network's parameters using techniques like backpropagation through time and gradient descent. The validation set will be used for hyperparameter tuning to mitigate overfitting. The chosen evaluation metrics will include **Mean Absolute Error (MAE), Root Mean Squared Error (RMSE), and the directional accuracy**. These metrics will assess the model's ability to predict the index's movements accurately, both in terms of magnitude and direction. Furthermore, we will conduct backtesting with out-of-sample data to assess the model's performance in a realistic trading scenario and evaluate its robustness against various market conditions.
The deployment of this model necessitates careful consideration of real-time data ingestion and model retraining. **A real-time data pipeline** will be established to feed current index values and economic indicators into the model. To adapt to evolving market dynamics, we will implement a retraining mechanism to ensure the model's continued accuracy. This will involve periodic retraining using updated data. Furthermore, we will incorporate **model interpretability tools** to understand the influence of individual variables on the forecasts. This will enable informed decision-making by identifying key economic factors driving the index's performance. Regular monitoring and validation of the model's accuracy will be essential to maintain its reliability, and this model has the potential to contribute significantly to the investment strategy.
ML Model Testing
n:Time series to forecast
p:Price signals of Dow Jones U.S. Technology index
j:Nash equilibria (Neural Network)
k:Dominated move of Dow Jones U.S. Technology index holders
a:Best response for Dow Jones U.S. Technology target price
For further technical information as per how our model work we invite you to visit the article below:
How do KappaSignal algorithms actually work?
Dow Jones U.S. Technology Index Forecast Strategic Interaction Table
Strategic Interaction Table Legend:
X axis: *Likelihood% (The higher the percentage value, the more likely the event will occur.)
Y axis: *Potential Impact% (The higher the percentage value, the more likely the price will deviate.)
Z axis (Grey to Black): *Technical Analysis%
Dow Jones U.S. Technology Index: Financial Outlook and Forecast
The Dow Jones U.S. Technology Index, representing a broad spectrum of the technology sector, currently presents a mixed financial outlook. The sector has experienced significant growth over the past decade, driven by advancements in areas like cloud computing, artificial intelligence, e-commerce, and mobile technology. This growth has translated into substantial revenue and profit increases for many companies within the index, boosting overall market capitalization. Furthermore, ongoing trends such as the increased reliance on technology for business operations, the burgeoning Internet of Things (IoT), and the continued expansion of digital services are expected to serve as strong tailwinds for the sector. However, the sector is not without its challenges. Rising interest rates, potential for increased regulatory scrutiny, and geopolitical uncertainties could impact growth. Investors should therefore carefully consider the diverse aspects of the technology landscape when evaluating the index's potential for future performance.
Several factors will significantly influence the financial forecast for the Dow Jones U.S. Technology Index. Innovation remains a critical driver. Companies that can successfully develop and commercialize cutting-edge technologies will likely outperform. The index's constituents are continuously investing heavily in research and development to stay ahead of the competition. The ongoing digital transformation, with businesses worldwide embracing technology to improve efficiency, enhance customer experience, and gain a competitive edge, is another crucial factor. Moreover, the expansion of the cloud computing market, the increasing adoption of artificial intelligence across various industries, and the growing demand for cybersecurity solutions are poised to create ample opportunities for growth within the index. The index's weighting of specific sub-sectors will also play a role. For example, the performance of semiconductor companies, software providers, and internet companies will have a pronounced effect on the overall index performance. Mergers and acquisitions activity will also play a role in the technological sector, as companies seek to acquire innovative technologies or expand their market share.
In projecting the future, certain risks must be considered when evaluating the Dow Jones U.S. Technology Index. Geopolitical tensions and trade disputes could disrupt supply chains, particularly impacting semiconductor and hardware manufacturers. Increased regulation, particularly concerning data privacy, antitrust concerns, and cybersecurity, could add to operating costs and could limit growth opportunities for some companies. The potential for an economic slowdown, with rising interest rates and decreased consumer spending, would reduce demand for certain technological products and services. The rapid pace of technological change presents a risk as well. The companies within the index must continuously innovate and adapt to avoid disruption by new technologies. Additionally, the index's valuation, which is often at a premium compared to the broader market, makes it vulnerable to market corrections. Investors must be prepared for higher volatility. The sector's susceptibility to cyberattacks and data breaches remains an ongoing concern.
Overall, the Dow Jones U.S. Technology Index is predicted to experience continued, albeit moderated, growth in the near to mid-term. The robust demand for technological solutions, persistent innovation, and the ongoing digital transformation are expected to generate substantial returns. However, investors need to be aware of the risks. The increasing geopolitical tensions, the potential for regulatory changes, and the chance of an economic slowdown are notable threats that could impact the performance of the index. Investors should conduct thorough due diligence and consider a long-term horizon, while also being prepared for volatility. They should also understand how to effectively diversify their portfolios to mitigate the risk. The sector's reliance on innovation and its sensitivity to economic conditions necessitate a cautious approach when assessing its financial outlook and forecast.
Rating | Short-Term | Long-Term Senior |
---|---|---|
Outlook | B3 | Ba1 |
Income Statement | Caa2 | Baa2 |
Balance Sheet | Caa2 | Baa2 |
Leverage Ratios | B2 | B1 |
Cash Flow | C | B2 |
Rates of Return and Profitability | B3 | Baa2 |
*An aggregate rating for an index summarizes the overall sentiment towards the companies it includes. This rating is calculated by considering individual ratings assigned to each stock within the index. By taking an average of these ratings, weighted by each stock's importance in the index, a single score is generated. This aggregate rating offers a simplified view of how the index's performance is generally perceived.
How does neural network examine financial reports and understand financial state of the company?
References
- Athey S. 2019. The impact of machine learning on economics. In The Economics of Artificial Intelligence: An Agenda, ed. AK Agrawal, J Gans, A Goldfarb. Chicago: Univ. Chicago Press. In press
- Künzel S, Sekhon J, Bickel P, Yu B. 2017. Meta-learners for estimating heterogeneous treatment effects using machine learning. arXiv:1706.03461 [math.ST]
- Hastie T, Tibshirani R, Tibshirani RJ. 2017. Extended comparisons of best subset selection, forward stepwise selection, and the lasso. arXiv:1707.08692 [stat.ME]
- D. S. Bernstein, S. Zilberstein, and N. Immerman. The complexity of decentralized control of Markov Decision Processes. In UAI '00: Proceedings of the 16th Conference in Uncertainty in Artificial Intelligence, Stanford University, Stanford, California, USA, June 30 - July 3, 2000, pages 32–37, 2000.
- V. Konda and J. Tsitsiklis. Actor-Critic algorithms. In Proceedings of Advances in Neural Information Processing Systems 12, pages 1008–1014, 2000
- Vapnik V. 2013. The Nature of Statistical Learning Theory. Berlin: Springer
- H. Kushner and G. Yin. Stochastic approximation algorithms and applications. Springer, 1997.