AUC Score :
Short-term Tactic1 :
Dominant Strategy :
Time series to forecast n:
ML Model Testing : Transductive Learning (ML)
Hypothesis Testing : Paired T-Test
Surveillance : Major exchange and OTC
1Short-term revised.
2Time series is updated based on short-term trends.
Key Points
The S&P GSCI Silver index is projected to experience moderate volatility. It is anticipated that the index will see an overall increase driven by rising industrial demand and safe-haven appeal. However, downside risks exist including potential macroeconomic slowdowns and fluctuations in the strength of the U.S. dollar, which could negatively impact silver prices. Geopolitical instability and supply chain disruptions are other factors to consider that could significantly influence the index's performance. Furthermore, increased investment in competing assets may also pose risks to the index.About S&P GSCI Silver Index
The S&P GSCI Silver is a commodity index that tracks the performance of the silver market. It's a sub-index within the broader S&P GSCI, which represents a diversified basket of global commodities. The index is designed to provide investors with a benchmark for silver's price movements and is often used as a reference point for investment strategies. It operates on the principle of a production-weighted methodology, meaning the index's composition is determined by the relative global production volumes of silver.
This index allows investors to gain exposure to the silver market without directly owning physical silver. Its value fluctuates based on the spot price of silver, reflecting supply and demand dynamics, geopolitical events, and macroeconomic factors. Investors can use the S&P GSCI Silver index to assess the performance of silver as an asset class, analyze its correlation with other assets, and to develop investment and hedging strategies, though potential investors should conduct thorough research to understand the risks associated with commodity investments.
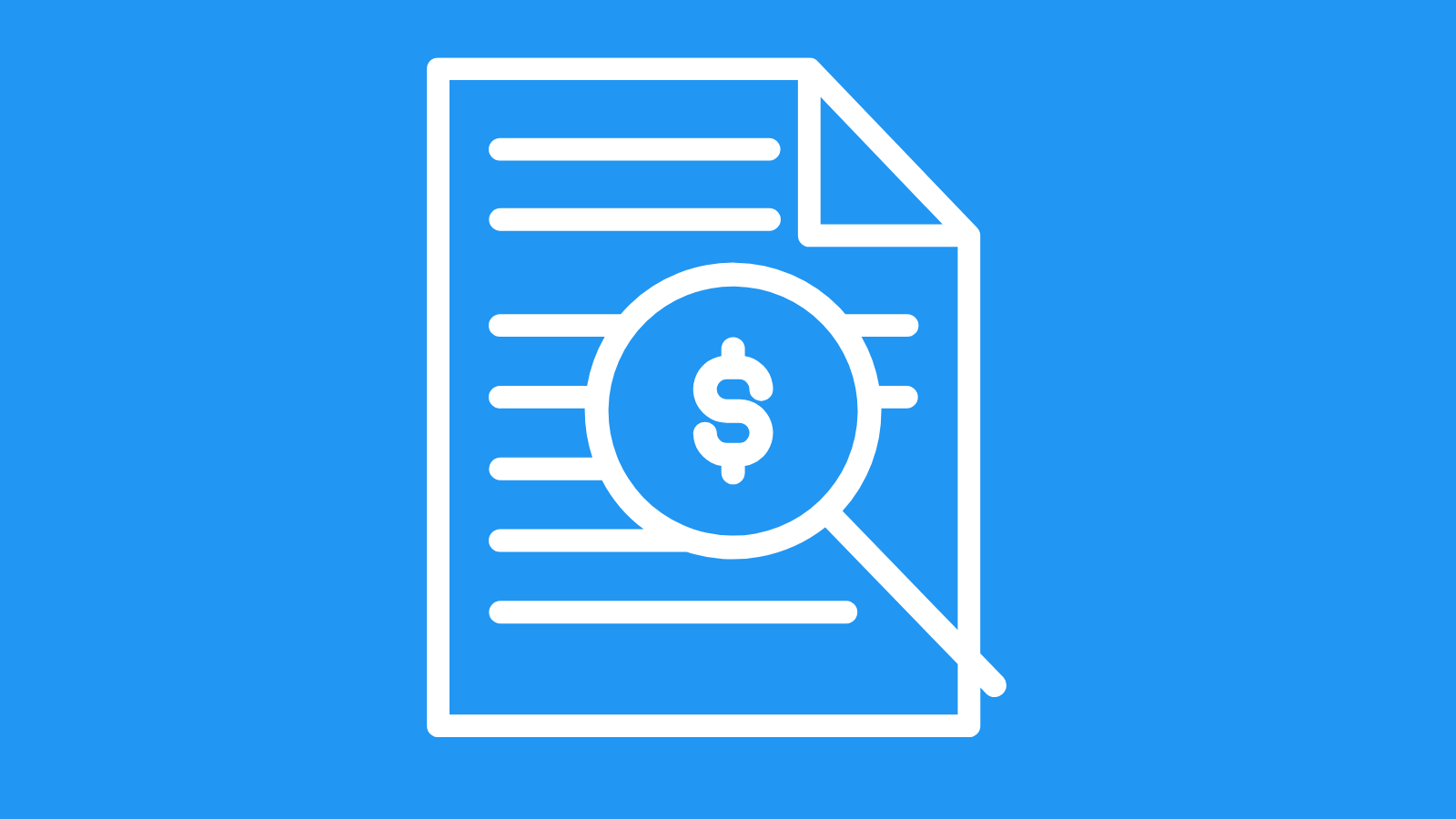
S&P GSCI Silver Index Forecasting Machine Learning Model
The development of a robust forecasting model for the S&P GSCI Silver index necessitates a multi-faceted approach, integrating both macroeconomic indicators and time-series data analysis. Our initial step involves the collection and preprocessing of a comprehensive dataset. This includes, but is not limited to, historical silver price data, alongside relevant economic variables such as the U.S. Dollar Index (DXY), inflation rates (CPI), industrial production indices, gold prices, and interest rate differentials. These economic factors are crucial as they directly influence silver's investment appeal and industrial demand. Data preprocessing will encompass handling missing values, outlier detection, and feature scaling to ensure data quality and consistency. Furthermore, feature engineering will be employed to create lagged variables, moving averages, and other derived features to capture temporal dependencies within the time series.
For model selection and training, we intend to explore a variety of machine learning algorithms. Initially, time-series models such as ARIMA (Autoregressive Integrated Moving Average) and its variations will serve as baseline models to establish a benchmark. Subsequently, advanced machine learning techniques, including Recurrent Neural Networks (RNNs), specifically LSTMs (Long Short-Term Memory), will be explored. LSTMs are well-suited for time-series forecasting due to their ability to capture long-range dependencies within the data. The model will be trained on a significant portion of the historical data, with a held-out portion reserved for validation and testing. Hyperparameter tuning will be conducted using techniques like grid search or random search, optimizing model performance based on metrics such as Mean Squared Error (MSE) and Mean Absolute Error (MAE). Cross-validation will also be employed to ensure model robustness.
The final model will be evaluated on its predictive accuracy, computational efficiency, and ability to generalize to unseen data. Performance will be assessed using established metrics. We plan to conduct sensitivity analyses to identify key variables driving price movements. Furthermore, the forecast results will be integrated into a comprehensive risk management framework. These forecasts can be used to optimize investment decisions, hedging strategies, and overall portfolio management. The model will be continuously monitored and refined as new data becomes available, and updated with new market dynamics to maintain forecast accuracy. The goal is to provide reliable insights for stakeholders, informed by data-driven analysis.
ML Model Testing
n:Time series to forecast
p:Price signals of S&P GSCI Silver index
j:Nash equilibria (Neural Network)
k:Dominated move of S&P GSCI Silver index holders
a:Best response for S&P GSCI Silver target price
For further technical information as per how our model work we invite you to visit the article below:
How do KappaSignal algorithms actually work?
S&P GSCI Silver Index Forecast Strategic Interaction Table
Strategic Interaction Table Legend:
X axis: *Likelihood% (The higher the percentage value, the more likely the event will occur.)
Y axis: *Potential Impact% (The higher the percentage value, the more likely the price will deviate.)
Z axis (Grey to Black): *Technical Analysis%
S&P GSCI Silver Index: Financial Outlook and Forecast
The S&P GSCI Silver index, reflecting the performance of silver futures contracts, presents a multifaceted financial outlook, largely shaped by macroeconomic factors, industrial demand, and investor sentiment. Currently, the index is influenced by global inflation rates, interest rate policies, and currency fluctuations. Concerns surrounding inflationary pressures have historically driven demand for silver as a hedge against eroding purchasing power, however, the effectiveness of silver as an inflation hedge can be debated, as its price movements also depend on various other factors. The interest rate decisions of major central banks such as the U.S. Federal Reserve play a crucial role; increasing interest rates typically strengthen the U.S. dollar, potentially making silver more expensive for buyers using other currencies and thus dampening demand. The index's performance is also tied to the strength of the dollar against other currencies, and a weaker dollar often leads to increased silver prices due to its inverse relationship. Furthermore, the index's financial outlook is closely tied to its liquidity, which reflects the ease with which silver futures contracts can be bought and sold without significantly impacting the price.
Industrial demand represents a significant component that influences the silver index. Silver has a wide range of industrial applications, including its use in solar panels, electronics, and other technological devices. The growth of renewable energy, particularly solar energy, has bolstered the demand for silver. The increasing adoption of electric vehicles and the expansion of electronics manufacturing contribute to the demand for silver, impacting the index positively. Conversely, economic slowdowns that negatively affect industrial activities can pressure silver prices. The supply-demand dynamics in the silver market also influence the index's trajectory. Factors affecting silver supply, such as mining activities and recycling rates, and the responsiveness of supply to price changes, must be assessed in the context of increasing industrial and investment demand.
Investor sentiment is a crucial element in assessing the S&P GSCI Silver index's financial outlook. The investor interest in silver and precious metals, driven by geopolitical uncertainties, economic concerns, and market volatility, directly impacts price fluctuations. When market volatility increases, investors often seek safe-haven assets like silver, which drives its price upwards. The futures market's trading activity, including the volume of open interest and trading trends, helps measure market sentiment. The price is prone to significant price swings due to its speculative nature and is therefore sensitive to news, events, and market sentiment. Market sentiment is also often closely tied to other precious metals like gold, with the two typically exhibiting strong correlations. The index's financial performance also relies on the availability of silver ETFs (Exchange Traded Funds), whose popularity indicates the degree of investor engagement with the silver market.
In summary, the S&P GSCI Silver index presents a positive outlook, assuming continued industrial demand, particularly from the renewable energy sector, and a weakening U.S. dollar. Inflation concerns are likely to offer support to silver prices, but the degree of price impact will hinge on the trajectory of other global economic factors. Investors should be alert to risks such as a stronger U.S. dollar, economic recessions, and changes in industrial demand. The index's performance could be hindered by an unexpected decrease in industrial demand or increased supply from mining companies. It also involves other factors like changes in investor sentiment and geopolitical stability, which could introduce heightened volatility in its price. Investors should therefore remain vigilant and assess the risk-reward profile before investing.
Rating | Short-Term | Long-Term Senior |
---|---|---|
Outlook | B3 | Ba3 |
Income Statement | Ba1 | Baa2 |
Balance Sheet | B3 | Baa2 |
Leverage Ratios | Caa2 | Ba3 |
Cash Flow | B2 | Caa2 |
Rates of Return and Profitability | C | Caa2 |
*An aggregate rating for an index summarizes the overall sentiment towards the companies it includes. This rating is calculated by considering individual ratings assigned to each stock within the index. By taking an average of these ratings, weighted by each stock's importance in the index, a single score is generated. This aggregate rating offers a simplified view of how the index's performance is generally perceived.
How does neural network examine financial reports and understand financial state of the company?
References
- G. Theocharous and A. Hallak. Lifetime value marketing using reinforcement learning. RLDM 2013, page 19, 2013
- Andrews, D. W. K. W. Ploberger (1994), "Optimal tests when a nuisance parameter is present only under the alternative," Econometrica, 62, 1383–1414.
- R. Howard and J. Matheson. Risk sensitive Markov decision processes. Management Science, 18(7):356– 369, 1972
- J. Z. Leibo, V. Zambaldi, M. Lanctot, J. Marecki, and T. Graepel. Multi-agent Reinforcement Learning in Sequential Social Dilemmas. In Proceedings of the 16th International Conference on Autonomous Agents and Multiagent Systems (AAMAS 2017), Sao Paulo, Brazil, 2017
- Arora S, Li Y, Liang Y, Ma T. 2016. RAND-WALK: a latent variable model approach to word embeddings. Trans. Assoc. Comput. Linguist. 4:385–99
- Kitagawa T, Tetenov A. 2015. Who should be treated? Empirical welfare maximization methods for treatment choice. Tech. Rep., Cent. Microdata Methods Pract., Inst. Fiscal Stud., London
- A. Shapiro, W. Tekaya, J. da Costa, and M. Soares. Risk neutral and risk averse stochastic dual dynamic programming method. European journal of operational research, 224(2):375–391, 2013