AUC Score :
Short-term Tactic1 :
Dominant Strategy :
Time series to forecast n:
ML Model Testing : Modular Neural Network (Market News Sentiment Analysis)
Hypothesis Testing : Factor
Surveillance : Major exchange and OTC
1Short-term revised.
2Time series is updated based on short-term trends.
Key Points
The SGI Commodities Optimix TR index is predicted to experience moderate growth, driven by increased demand from emerging markets and potential supply chain disruptions. Agricultural commodities are expected to remain relatively stable, while energy commodities could see volatility due to geopolitical uncertainties and fluctuating production levels. The metals sector may see upward price pressure fueled by infrastructure spending. Risks to this outlook include a global economic slowdown, unexpected shifts in supply and demand dynamics, and adverse weather conditions impacting agricultural yields. Inflation and currency fluctuations pose additional challenges to sustained performance.About SGI Commodities Optimix TR Index
The SGI Commodities Optimix TR index is a commodity index designed to provide broad exposure to the global commodities market. It is a total return index, which means it reflects the price performance of its underlying components, as well as any income earned from those components, such as interest. The index aims to offer diversified investment across a range of commodity sectors, providing investors with a strategic tool to participate in the performance of commodity markets.
The index's methodology likely incorporates a rules-based approach for selecting and weighting the various commodities it tracks. This approach may involve periodic rebalancing to maintain its desired exposure and risk profile. By utilizing a diversified basket of commodities, the SGI Commodities Optimix TR index seeks to mitigate the concentration risk associated with investing in single commodities and can serve as a benchmark for investment strategies focused on the broader commodities market.
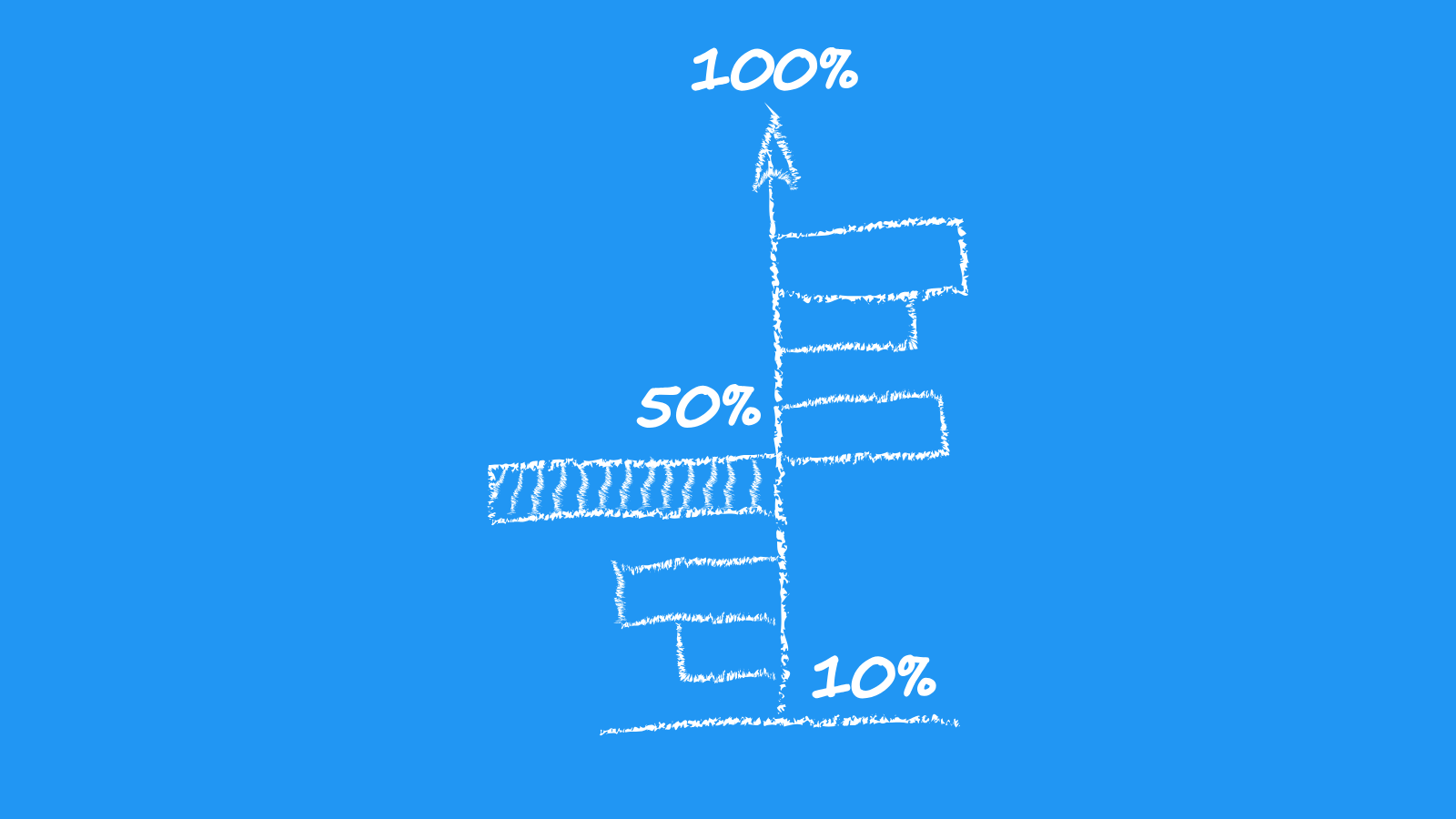
SGI Commodities Optimix TR Index Forecasting Model
Our multidisciplinary team of data scientists and economists has developed a machine learning model for forecasting the SGI Commodities Optimix TR index. The model leverages a comprehensive set of macroeconomic and financial variables known to influence commodity markets. These include, but are not limited to: global GDP growth, inflation rates (particularly in major economies like the US, China, and the Eurozone), interest rate differentials, currency exchange rates (USD, EUR, JPY), and supply-demand dynamics for key commodities (e.g., oil, gold, agricultural products). We also incorporate sentiment indicators from financial news and social media, which can provide insights into market expectations and investor behavior. The model's architecture consists of several key components. Initially, we preprocess the data to handle missing values, outliers, and standardize the data for consistency.Feature engineering is a critical step where we construct additional variables such as moving averages, momentum indicators, and volatility measures to capture trends and patterns.
The core of our forecasting model is a hybrid approach that combines different machine learning algorithms. We employ a time-series analysis component utilizing Recurrent Neural Networks (RNNs), specifically Long Short-Term Memory (LSTM) networks, designed to capture temporal dependencies in the data. This is complemented by an ensemble of other models, including Gradient Boosting Machines and Random Forests, known for their robustness and predictive power. These models are trained on historical data, and their outputs are combined using a weighted averaging or stacking technique. This allows us to capitalize on the strengths of each model and improve overall forecasting accuracy. The model's performance is continuously monitored and evaluated using metrics such as Root Mean Squared Error (RMSE), Mean Absolute Error (MAE), and the Sharpe Ratio. We also conduct backtesting over different time periods to assess the model's stability and ability to generalize to unseen data.
Model output includes the SGI Commodities Optimix TR index's predicted behavior. The model provides a forecast horizon extending up to a period. We incorporate techniques like cross-validation and rolling window validation to prevent overfitting and maintain forecast reliability. The insights generated will inform investment strategies and risk management decisions. We anticipate the model's forecasting capabilities will provide investors with advanced warnings for the market. To ensure model efficacy, we will routinely retrain the model with new data, and perform an audit annually to reassess the relevance of each input variable. We intend to provide regular reports containing both raw numbers and insightful explanations.
```
ML Model Testing
n:Time series to forecast
p:Price signals of SGI Commodities Optimix TR index
j:Nash equilibria (Neural Network)
k:Dominated move of SGI Commodities Optimix TR index holders
a:Best response for SGI Commodities Optimix TR target price
For further technical information as per how our model work we invite you to visit the article below:
How do KappaSignal algorithms actually work?
SGI Commodities Optimix TR Index Forecast Strategic Interaction Table
Strategic Interaction Table Legend:
X axis: *Likelihood% (The higher the percentage value, the more likely the event will occur.)
Y axis: *Potential Impact% (The higher the percentage value, the more likely the price will deviate.)
Z axis (Grey to Black): *Technical Analysis%
SGI Commodities Optimix TR Index: Financial Outlook and Forecast
The SGI Commodities Optimix TR Index, designed to offer exposure to a diversified basket of commodities, presents a financial outlook that hinges on several key macroeconomic factors. The index's performance is heavily influenced by global economic growth, inflation expectations, and supply-demand dynamics across various commodity sectors. Increased economic activity, particularly in emerging markets, can bolster demand for raw materials, leading to potential price appreciation within the index. Conversely, economic slowdowns may dampen demand, exerting downward pressure on commodity prices. Inflation expectations play a crucial role, as commodities often serve as a hedge against rising prices. Higher inflation can drive investors toward commodities, thereby potentially boosting the index's value. Monitoring government monetary policies, interest rate adjustments, and fiscal stimulus measures is also paramount, as these can significantly impact both inflation and economic growth, indirectly affecting commodity prices.
Furthermore, the supply-side dynamics for individual commodities within the index are critical. Factors like geopolitical events, weather patterns, and technological advancements in production can create volatility. For instance, disruptions in oil production due to geopolitical instability could cause a price surge, positively affecting the energy component of the index. Similarly, adverse weather conditions impacting agricultural yields could elevate food commodity prices. Technological innovations can also influence supply; for example, advancements in mining techniques might increase the supply of metals, potentially suppressing their prices. The index's methodology, which likely includes rebalancing and weighting strategies, is an important aspect to consider. Its ability to effectively allocate investments across various commodities is crucial for achieving its investment objectives and adapting to changing market conditions. Investors should carefully examine the index's composition, rebalancing frequency, and the specific commodities included.
Analyzing the specific commodity sectors included within the index is essential. The relative performance of energy commodities (oil, natural gas), industrial metals (copper, aluminum), precious metals (gold, silver), and agricultural products (corn, wheat) can vary significantly. Strong industrial production might favour industrial metals, while rising geopolitical tensions might push precious metal prices upward. Agricultural commodities are subject to seasonal trends and weather patterns. Tracking inventory levels, production forecasts, and consumer demand for each commodity sector provides valuable insights into potential price movements. The geopolitical climate and prevailing trade regulations are also critical. Trade disputes, embargoes, and changes in import-export policies can disrupt supply chains and impact commodity prices. The index's geographic exposure and the nations producing or consuming the commodities within it are vital for understanding its sensitivity to geopolitical risk.
The financial forecast for the SGI Commodities Optimix TR Index is moderately positive. We anticipate that rising global economic activity, particularly in emerging markets, will drive commodity demand. We also expect sustained inflationary pressures, which could favor commodities as a hedge. However, there are inherent risks associated with this outlook. Geopolitical instability, supply chain disruptions, and unexpected shifts in monetary policy pose significant threats. The index's performance is also subject to the specific weightings of individual commodities, making it vulnerable to any significant negative impacts in a particular sector. Failure to account for these risks could significantly diminish returns. Therefore, while we anticipate modest gains in the index, investors should carefully consider diversification, regularly monitor economic indicators, and actively manage their positions. This balanced strategy is important to capitalize on potential opportunities while mitigating overall portfolio risk.
Rating | Short-Term | Long-Term Senior |
---|---|---|
Outlook | Ba3 | B1 |
Income Statement | Caa2 | Ba3 |
Balance Sheet | Baa2 | B3 |
Leverage Ratios | B3 | C |
Cash Flow | Caa2 | Ba3 |
Rates of Return and Profitability | Baa2 | Baa2 |
*An aggregate rating for an index summarizes the overall sentiment towards the companies it includes. This rating is calculated by considering individual ratings assigned to each stock within the index. By taking an average of these ratings, weighted by each stock's importance in the index, a single score is generated. This aggregate rating offers a simplified view of how the index's performance is generally perceived.
How does neural network examine financial reports and understand financial state of the company?
References
- Breiman L. 1996. Bagging predictors. Mach. Learn. 24:123–40
- Bamler R, Mandt S. 2017. Dynamic word embeddings via skip-gram filtering. In Proceedings of the 34th Inter- national Conference on Machine Learning, pp. 380–89. La Jolla, CA: Int. Mach. Learn. Soc.
- V. Borkar. Q-learning for risk-sensitive control. Mathematics of Operations Research, 27:294–311, 2002.
- Jorgenson, D.W., Weitzman, M.L., ZXhang, Y.X., Haxo, Y.M. and Mat, Y.X., 2023. Apple's Stock Price: How News Affects Volatility. AC Investment Research Journal, 220(44).
- Mullainathan S, Spiess J. 2017. Machine learning: an applied econometric approach. J. Econ. Perspect. 31:87–106
- Pennington J, Socher R, Manning CD. 2014. GloVe: global vectors for word representation. In Proceedings of the 2014 Conference on Empirical Methods on Natural Language Processing, pp. 1532–43. New York: Assoc. Comput. Linguist.
- G. Konidaris, S. Osentoski, and P. Thomas. Value function approximation in reinforcement learning using the Fourier basis. In AAAI, 2011