AUC Score :
Short-term Tactic1 :
Dominant Strategy :
Time series to forecast n:
ML Model Testing : Transductive Learning (ML)
Hypothesis Testing : Wilcoxon Sign-Rank Test
Surveillance : Major exchange and OTC
1Short-term revised.
2Time series is updated based on short-term trends.
Key Points
SQ's future appears uncertain. The company faces challenges in a competitive market, potentially impacting its growth trajectory. Strong headwinds in customer acquisition and retention could pressure revenue projections. Conversely, SQ may benefit from an aging population and the ongoing demand for insurance products, potentially driving modest gains. Regulatory changes and shifts in the healthcare landscape pose significant risks, requiring strategic adaptation. Failure to successfully navigate these complexities could lead to significant financial underperformance, including reduced profitability and share value erosion.About SelectQuote Inc.
SelectQuote is a direct-to-consumer retail company specializing in the distribution of insurance policies. Founded in 1985, SQ primarily operates in the United States, offering a variety of insurance products including health, auto, home, and life insurance. The company employs a sales-oriented model, utilizing a large team of licensed insurance agents who connect with potential customers through inbound and outbound phone calls. They focus on providing personalized insurance quotes and comparing policies from multiple insurance carriers to help consumers find the best coverage options. SQ aims to simplify the insurance shopping process.
SQ's business model depends on its ability to attract and retain customers, and to build strong relationships with insurance carriers. The company generates revenue through commissions paid by the insurance providers when policies are sold. They also operate online, allowing customers to receive quotes and information. SQ competes with other insurance distributors and online platforms. Their key to success depends on customer service and a convenient process to find the best insurance.
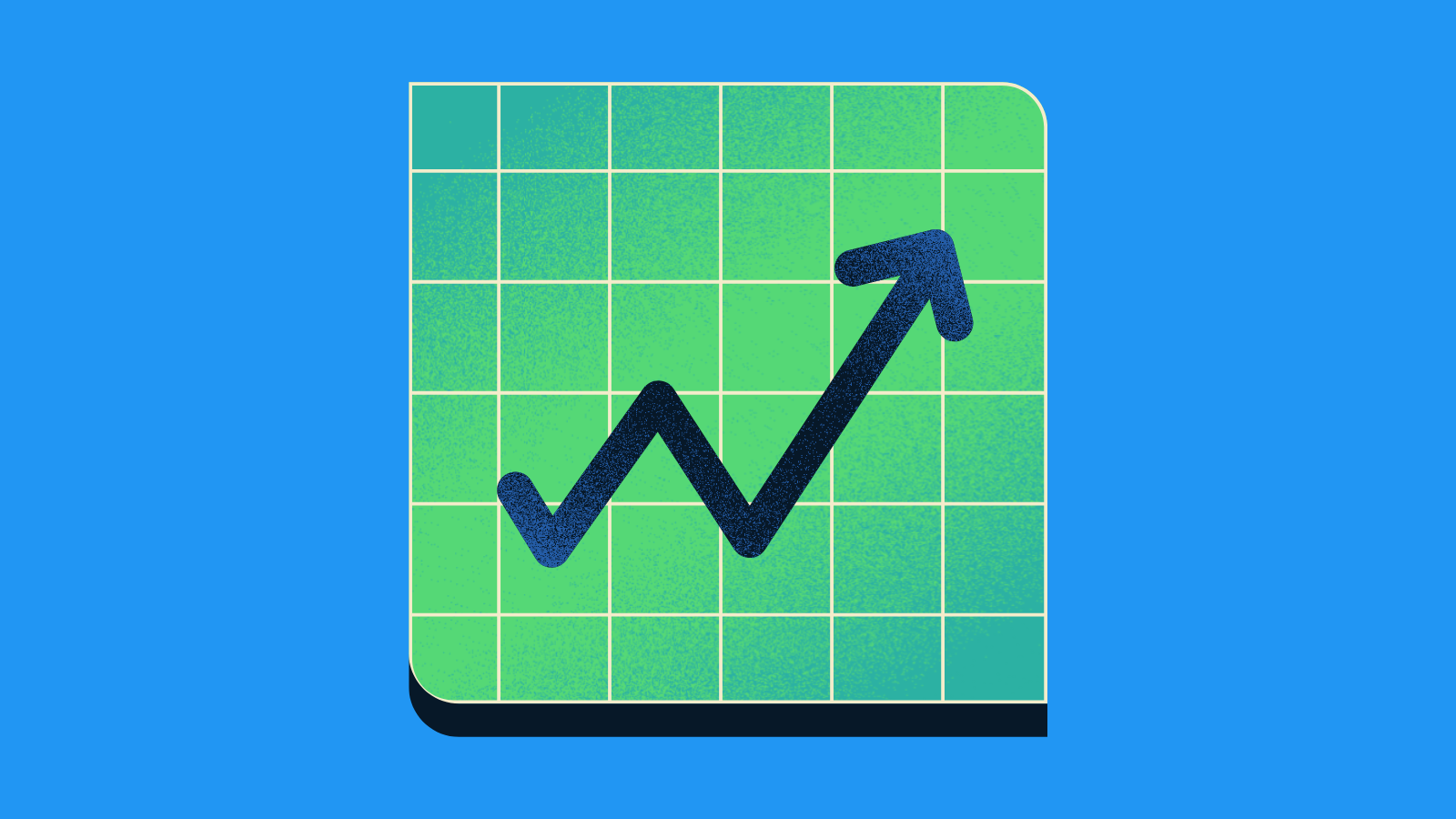
SLQT Stock Forecast Model
Our team of data scientists and economists proposes a machine learning model to forecast the performance of SelectQuote Inc. (SLQT) common stock. The model will employ a hybrid approach, combining time series analysis with machine learning techniques. The time series component will analyze historical stock data, including open, high, low, and close prices, alongside trading volume and moving averages. We will utilize techniques like ARIMA (Autoregressive Integrated Moving Average) and its variants to capture the inherent temporal dependencies in the stock's past behavior. This component will provide a baseline forecast based on the historical trends. We will also analyze the long and short term trends.
Simultaneously, we will incorporate external economic and financial data into the model. This includes macroeconomic indicators such as GDP growth, inflation rates, unemployment figures, and interest rates. Sector-specific data, such as competitor performance, health insurance market trends, and changes in regulatory environments, will also be factored in. We will utilize machine learning algorithms, such as Random Forest and Gradient Boosting, to capture the complex, non-linear relationships between these external factors and SLQT's stock performance. This component will help the model understand the market dynamics and its impact on the stock. We will also collect the data from reliable source like: SEC filings, news articles, company reports, and analyst ratings.
The final model will be a composite of the time series and machine learning components, with the ability to learn from new data as it becomes available. We will use rigorous validation methods, including backtesting and out-of-sample testing, to assess the model's accuracy and robustness. The model's outputs will include a forecast for SLQT's performance. We will provide this forecast with confidence intervals and clear explanations. We will regularly monitor the model's performance and recalibrate as needed to adapt to evolving market conditions. The success of the model will largely depend on the quality and availability of data and the ability to capture the complex financial market drivers.
ML Model Testing
n:Time series to forecast
p:Price signals of SelectQuote Inc. stock
j:Nash equilibria (Neural Network)
k:Dominated move of SelectQuote Inc. stock holders
a:Best response for SelectQuote Inc. target price
For further technical information as per how our model work we invite you to visit the article below:
How do KappaSignal algorithms actually work?
SelectQuote Inc. Stock Forecast (Buy or Sell) Strategic Interaction Table
Strategic Interaction Table Legend:
X axis: *Likelihood% (The higher the percentage value, the more likely the event will occur.)
Y axis: *Potential Impact% (The higher the percentage value, the more likely the price will deviate.)
Z axis (Grey to Black): *Technical Analysis%
SelectQuote Inc. (SLQT) Financial Outlook and Forecast
The financial outlook for SLQT presents a mixed picture, influenced by a confluence of factors within the health insurance marketplace and the company's operational strategies. The company has been navigating a challenging environment characterized by increased competition, evolving consumer preferences, and shifts in the regulatory landscape. Recent financial performance has been marked by revenue fluctuations and profitability pressures. Management has undertaken initiatives to streamline operations, improve sales efficiency, and broaden product offerings, which are crucial to driving long-term sustainable growth. The company's strategy appears to be centered on improving its online sales capabilities, strengthening its relationships with insurance carriers, and expanding into new market segments. These efforts may take time to yield measurable results.
The company's strategic focus on digital transformation and technological advancements is crucial for future performance. Success hinges on its ability to adapt to the increasing digitization of the insurance industry and provide consumers with a seamless and user-friendly experience. This includes investing in user interfaces, data analytics, and customer relationship management systems. SLQT's ability to attract and retain qualified sales agents will also be critical, particularly given the competitive market for talent. Additionally, the company's partnership with established insurance carriers is essential to ensure a consistent supply of products and services to offer its customer base. The overall health of the Medicare Advantage and health insurance markets is important for SLQT's success, as changes in these markets will directly affect the company's sales and revenue streams.
Analyzing the macro environment, several external factors will play a key role. Regulatory changes regarding healthcare, particularly regarding insurance sales practices and pricing models, will impact the company's operations and costs. Economic factors, such as inflation and interest rate changes, influence consumer spending and the overall affordability of health insurance plans. Furthermore, technological disruptions, such as the emergence of new platforms and data analytics tools, can create opportunities for market differentiation. Understanding these market trends is crucial for SLQT to adapt its strategies and remain competitive. SLQT should demonstrate its responsiveness to market changes, adapt its product offerings and its distribution channels.
Overall, the financial forecast for SLQT is cautiously optimistic. The ongoing implementation of its strategic initiatives, combined with its focus on digital transformation, positions the company for potential improvement over the long term. However, the company's future success is subject to several risks. These include, but are not limited to, competition from other insurance distributors, regulatory scrutiny, and economic downturns. The ability to execute its strategic plan effectively, manage operating costs, and navigate the changing healthcare landscape will be key to determining its financial performance and its sustainability in the industry. Therefore, while the long-term outlook appears favorable, significant risks remain, and investors should closely monitor the company's progress in mitigating them.
Rating | Short-Term | Long-Term Senior |
---|---|---|
Outlook | Ba3 | Ba1 |
Income Statement | Caa2 | Baa2 |
Balance Sheet | Ba1 | Baa2 |
Leverage Ratios | Baa2 | Caa2 |
Cash Flow | B2 | Ba1 |
Rates of Return and Profitability | B3 | Ba3 |
*Financial analysis is the process of evaluating a company's financial performance and position by neural network. It involves reviewing the company's financial statements, including the balance sheet, income statement, and cash flow statement, as well as other financial reports and documents.
How does neural network examine financial reports and understand financial state of the company?
References
- S. Devlin, L. Yliniemi, D. Kudenko, and K. Tumer. Potential-based difference rewards for multiagent reinforcement learning. In Proceedings of the Thirteenth International Joint Conference on Autonomous Agents and Multiagent Systems, May 2014
- P. Milgrom and I. Segal. Envelope theorems for arbitrary choice sets. Econometrica, 70(2):583–601, 2002
- J. N. Foerster, Y. M. Assael, N. de Freitas, and S. Whiteson. Learning to communicate with deep multi-agent reinforcement learning. In Advances in Neural Information Processing Systems 29: Annual Conference on Neural Information Processing Systems 2016, December 5-10, 2016, Barcelona, Spain, pages 2137–2145, 2016.
- P. Artzner, F. Delbaen, J. Eber, and D. Heath. Coherent measures of risk. Journal of Mathematical Finance, 9(3):203–228, 1999
- Jorgenson, D.W., Weitzman, M.L., ZXhang, Y.X., Haxo, Y.M. and Mat, Y.X., 2023. Can Neural Networks Predict Stock Market?. AC Investment Research Journal, 220(44).
- D. Bertsekas. Dynamic programming and optimal control. Athena Scientific, 1995.
- Chernozhukov V, Newey W, Robins J. 2018c. Double/de-biased machine learning using regularized Riesz representers. arXiv:1802.08667 [stat.ML]