AUC Score :
Short-term Tactic1 :
Dominant Strategy :
Time series to forecast n:
ML Model Testing : Modular Neural Network (Market Volatility Analysis)
Hypothesis Testing : Multiple Regression
Surveillance : Major exchange and OTC
1Short-term revised.
2Time series is updated based on short-term trends.
Key Points
SCHR is projected to experience moderate growth driven by increasing demand for its computational drug discovery platform. This will likely be fueled by an uptick in research and development spending within the pharmaceutical industry. However, the company faces risks including intense competition from both established and emerging players in the field. Regulatory hurdles related to drug approvals could delay or negatively impact its revenue streams. The company is also susceptible to economic downturns that might impact its ability to attract new clients and retain existing ones. Furthermore, failure in technological advancements or challenges in securing new partnerships could hinder its expansion plans, leading to potential stagnation or decline.About Schrodinger
Schrodinger is a prominent scientific software company specializing in computational chemistry, physics, and biology. The company develops and sells software for drug discovery, materials science, and related research fields. Schrodinger's products are used by researchers in both academia and the pharmaceutical and biotechnology industries to model and simulate molecular interactions, predict the properties of molecules, and accelerate the discovery and development of new medicines and materials. The company's focus is on providing advanced computational tools that enhance scientific productivity and innovation.
Schrodinger's business model revolves around providing software licenses and associated services, including support, training, and consulting. Schrodinger employs a team of scientists and software engineers who are committed to pushing the boundaries of computational science. Schrodinger's long-term objectives revolve around continuous advancement of its software platforms, expansion of its customer base, and exploration of new scientific applications for its technologies. The company aims to remain at the forefront of the computational drug discovery and materials science arenas.
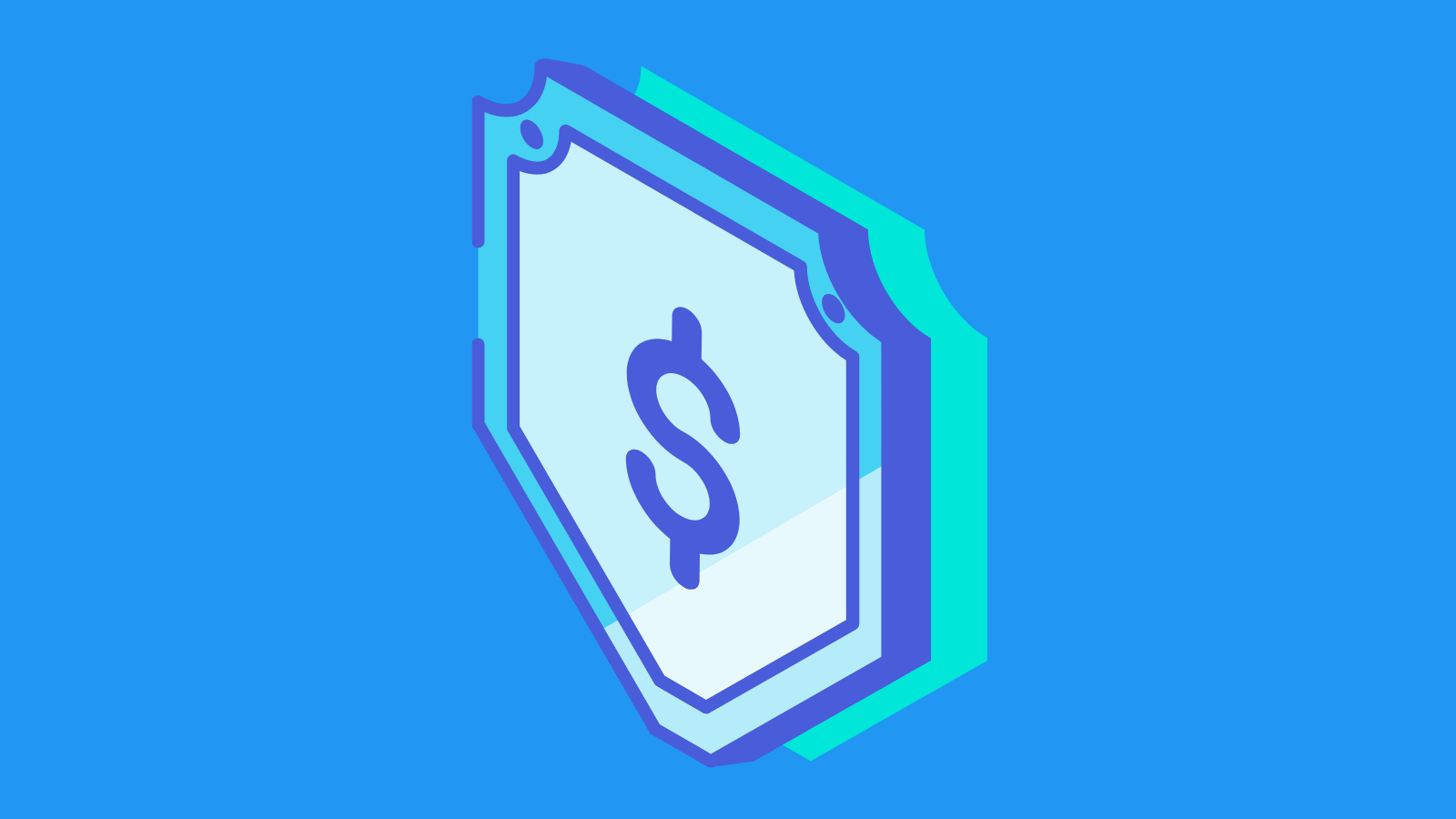
SDGR Stock Forecast Model
Our team of data scientists and economists has developed a machine learning model to forecast Schrodinger Inc. (SDGR) common stock performance. The model leverages a comprehensive set of features encompassing both fundamental and technical indicators. Fundamental factors include, but are not limited to, revenue growth, research and development expenditure, earnings per share, debt-to-equity ratio, and institutional ownership. These variables are critical in understanding the company's financial health, innovation pipeline, and overall market position. Technical indicators, such as moving averages, relative strength index (RSI), trading volume, and historical price volatility, are incorporated to capture market sentiment and short-term price movements. The data is sourced from reputable financial data providers and is cleaned, preprocessed, and engineered to optimize the model's predictive power.
The machine learning model employs a multi-faceted approach. Several algorithms have been explored, including but not limited to, Recurrent Neural Networks (RNNs) specifically Long Short-Term Memory (LSTM) for time series analysis and Gradient Boosting Machines (GBM) for enhanced predictive accuracy. The selection of algorithm will be dependent on the performance evaluation. Hyperparameter tuning and cross-validation techniques are used to minimize overfitting and ensure model robustness. A carefully designed ensemble methodology, which combines the strengths of multiple models, is used for the final forecast to increase reliability and reduce single-model biases. The model output will be a probabilistic forecast, providing a range of potential outcomes with associated confidence levels, instead of a single point prediction, for a more realistic assessment of the uncertainty inherent in stock market forecasting.
The forecast generated by the model is continuously monitored and updated. The data is refreshed regularly to ensure that the model stays relevant and aligned with the evolving market conditions and company-specific developments. Backtesting is performed on historical data to validate model performance and identify potential weaknesses. The model will be periodically re-trained with new data to incorporate changes in the market dynamics and company fundamentals. Our team conducts a thorough sensitivity analysis to understand the model's response to changes in key input variables and to identify any potential vulnerabilities. The goal is to provide a reliable and insightful forecast that will support Schrodinger Inc.'s investment decisions and financial planning processes.
ML Model Testing
n:Time series to forecast
p:Price signals of Schrodinger stock
j:Nash equilibria (Neural Network)
k:Dominated move of Schrodinger stock holders
a:Best response for Schrodinger target price
For further technical information as per how our model work we invite you to visit the article below:
How do KappaSignal algorithms actually work?
Schrodinger Stock Forecast (Buy or Sell) Strategic Interaction Table
Strategic Interaction Table Legend:
X axis: *Likelihood% (The higher the percentage value, the more likely the event will occur.)
Y axis: *Potential Impact% (The higher the percentage value, the more likely the price will deviate.)
Z axis (Grey to Black): *Technical Analysis%
Schrödinger Inc. (SDGR) Financial Outlook and Forecast
Schrödinger, a computational platform provider for drug discovery and materials science, presents a complex financial landscape, with its future intricately linked to its ability to execute its long-term strategic goals. The company's primary revenue streams stem from its software platform and its drug discovery collaborations. Recent performance indicates a consistent growth trajectory in software revenue, driven by the increasing adoption of its platform by pharmaceutical and materials science companies. Drug discovery revenue, on the other hand, tends to be more volatile, contingent on the progress and success of its internal pipeline and collaborative projects. The company has invested heavily in research and development (R&D) to enhance its platform capabilities and expand its pipeline, which has an immediate impact on profitability. The current financial outlook should be viewed through the lens of these intertwined and dynamic factors. Growth in the software segment coupled with successful advancements in the drug discovery pipeline are crucial for sustained revenue expansion.
The financial forecasts for SDGR are sensitive to several key variables. Revenue growth will be driven by the adoption rate of Schrödinger's software platform and the outcomes of its drug discovery programs. The expansion of strategic partnerships and collaborations with major pharmaceutical companies could also represent a significant boost. Profitability hinges on managing R&D expenses effectively and increasing operating leverage. This means that while ongoing investment in R&D is vital to the company's long-term prospects, controlling the growth of these costs relative to revenue will be critical. The capital allocation decisions for SDGR will also play a vital role, including potential investments in infrastructure, acquisitions, and business development. Cash flow generation is therefore essential to funding these activities, which can also be seen in terms of maintaining a positive cash position. The company's current cash position and its ability to secure additional funding when needed are vital for providing resilience.
Schrödinger's long-term financial outlook depends on several key drivers, including technological advances in computational chemistry, the rate of adoption of its software platform, and the success of its drug discovery pipeline. The computational chemistry landscape is extremely competitive and quickly evolving, meaning it faces competition from both established software providers and emerging technology companies. Successful drug discovery, in particular, is an inherently high-risk, high-reward activity that can be affected by factors such as clinical trial outcomes and regulatory approvals. The market for both drug discovery and the company's software offerings is subject to economic conditions, including broader biotechnology industry trends. Schrödinger's ability to secure and maintain intellectual property rights, and navigate complex regulatory frameworks, will be very important to its future financial performance. Strategic partnerships, acquisitions and any potential partnerships with larger pharmaceutical companies could also affect the forecast.
The prediction for Schrödinger is cautiously optimistic. The company has a solid foundation and is well-positioned to capitalize on emerging opportunities in drug discovery and materials science. The continued adoption of its software platform and the positive outcomes of its drug discovery programs would boost its growth prospects. However, there are notable risks. The volatility in drug discovery revenue, the competitive landscape, and the potential for unexpected failures in clinical trials could impede growth. Additionally, any significant increase in operating expenses without commensurate revenue gains could negatively affect profitability and cash flow. Overall, Schrödinger is believed to have a positive outlook, with the caveat that investors should be aware of the inherent uncertainties and risks associated with the drug discovery industry and the competitive environment.
```
Rating | Short-Term | Long-Term Senior |
---|---|---|
Outlook | B2 | Ba3 |
Income Statement | Baa2 | B3 |
Balance Sheet | Baa2 | Caa2 |
Leverage Ratios | C | Baa2 |
Cash Flow | Caa2 | B1 |
Rates of Return and Profitability | B2 | B1 |
*Financial analysis is the process of evaluating a company's financial performance and position by neural network. It involves reviewing the company's financial statements, including the balance sheet, income statement, and cash flow statement, as well as other financial reports and documents.
How does neural network examine financial reports and understand financial state of the company?
References
- Andrews, D. W. K. W. Ploberger (1994), "Optimal tests when a nuisance parameter is present only under the alternative," Econometrica, 62, 1383–1414.
- Li L, Chu W, Langford J, Moon T, Wang X. 2012. An unbiased offline evaluation of contextual bandit algo- rithms with generalized linear models. In Proceedings of 4th ACM International Conference on Web Search and Data Mining, pp. 297–306. New York: ACM
- Bickel P, Klaassen C, Ritov Y, Wellner J. 1998. Efficient and Adaptive Estimation for Semiparametric Models. Berlin: Springer
- Friedman JH. 2002. Stochastic gradient boosting. Comput. Stat. Data Anal. 38:367–78
- Krizhevsky A, Sutskever I, Hinton GE. 2012. Imagenet classification with deep convolutional neural networks. In Advances in Neural Information Processing Systems, Vol. 25, ed. Z Ghahramani, M Welling, C Cortes, ND Lawrence, KQ Weinberger, pp. 1097–105. San Diego, CA: Neural Inf. Process. Syst. Found.
- Athey S, Imbens GW. 2017b. The state of applied econometrics: causality and policy evaluation. J. Econ. Perspect. 31:3–32
- Arora S, Li Y, Liang Y, Ma T. 2016. RAND-WALK: a latent variable model approach to word embeddings. Trans. Assoc. Comput. Linguist. 4:385–99