AUC Score :
Short-term Tactic1 :
Dominant Strategy :
Time series to forecast n:
ML Model Testing : Modular Neural Network (Market News Sentiment Analysis)
Hypothesis Testing : Pearson Correlation
Surveillance : Major exchange and OTC
1Short-term revised.
2Time series is updated based on short-term trends.
Key Points
The S-Net ITG Agriculture USD index is projected to experience a period of moderate growth driven by increasing global demand for agricultural products and ongoing technological advancements in farming practices. This positive trajectory could be tempered by several key risks. Adverse weather conditions, such as droughts or floods, pose a significant threat to crop yields and, consequently, the index's performance. Geopolitical instability could disrupt trade routes and supply chains, leading to price volatility. Furthermore, changes in government policies, particularly those related to subsidies, tariffs, or environmental regulations, could have a substantial impact. Finally, unexpected outbreaks of plant diseases or pest infestations represent a continuous, unpredictable danger.About S-Net ITG Agriculture USD Index
The S-Net ITG Agriculture USD Index serves as a financial benchmark designed to monitor the performance of a basket of agricultural commodities. This index, denominated in US dollars, provides investors with a mechanism to track the overall trends and movements within the global agricultural market. It typically encompasses a diverse selection of agricultural products, which may include but are not limited to grains, oilseeds, soft commodities (like sugar and coffee), and livestock-related products. The index is meticulously constructed using a rules-based methodology to ensure transparency and replicability.
The S-Net ITG Agriculture USD Index is frequently employed by financial institutions, fund managers, and individual investors seeking exposure to the agricultural sector. As a market indicator, it helps assess the investment potential of agricultural commodities, allowing investors to make informed decisions based on market dynamics. The index's value is susceptible to several macroeconomic factors, including weather patterns, supply and demand fluctuations, geopolitical events, and currency exchange rates, creating both opportunities and risks for investors. The index provides a crucial reference point to evaluate the efficacy of investment strategies within the agricultural sphere.
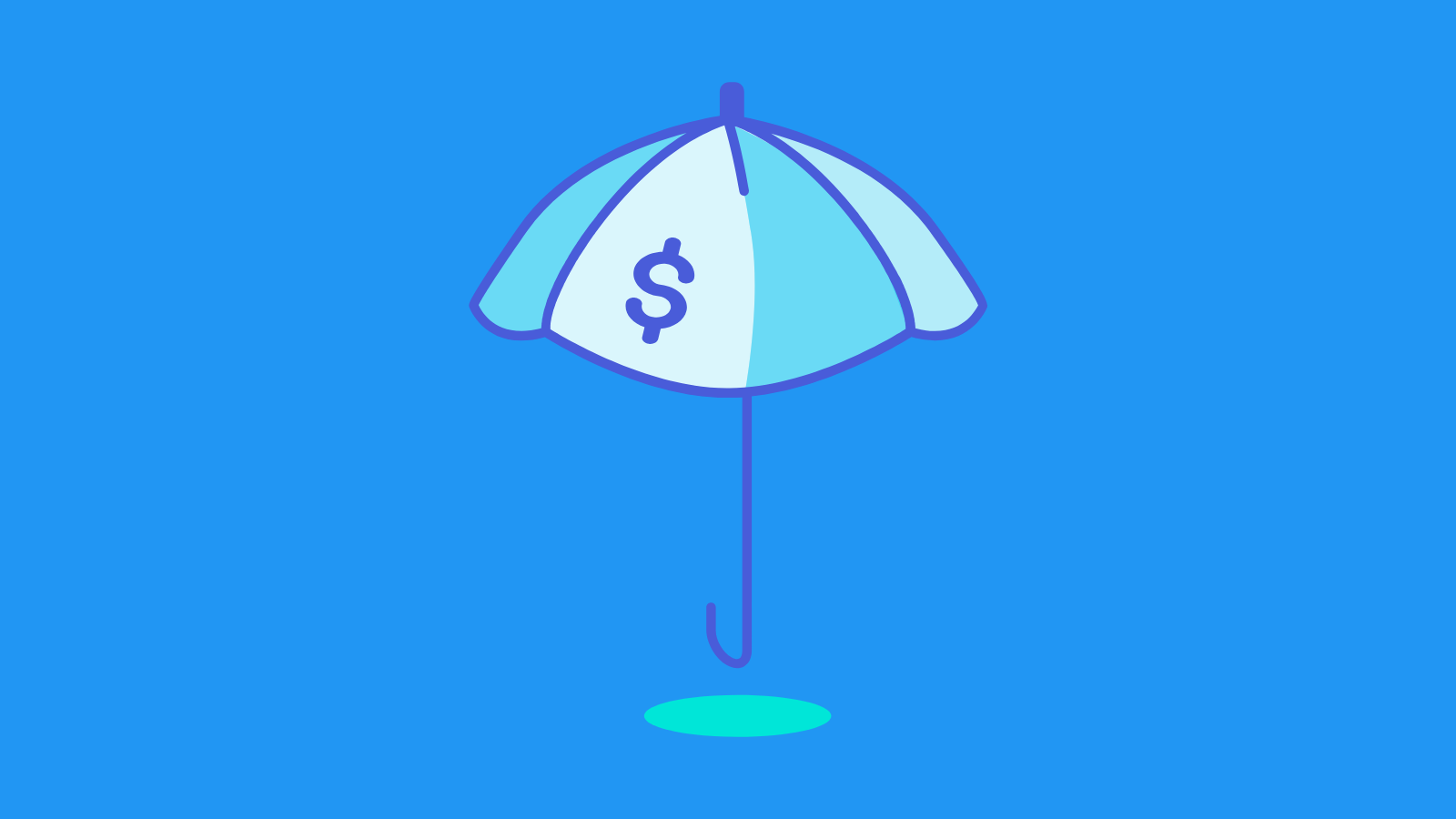
S-Net ITG Agriculture USD Index Forecast Model
Our team of data scientists and economists has developed a machine learning model designed to forecast the S-Net ITG Agriculture USD index. The core of our model employs a hybrid approach, leveraging the strengths of both time series analysis and macroeconomic indicators. We utilize a Recurrent Neural Network (RNN), specifically a Long Short-Term Memory (LSTM) network, to capture the temporal dependencies inherent in the index's historical data. This allows the model to learn from past price fluctuations, identify trends, and recognize recurring patterns. Crucially, we preprocess the data with careful consideration for seasonality and potential outliers, ensuring the model's robustness and accuracy. Feature engineering is a vital component, incorporating lagged values of the index itself to exploit autocorrelation.
To further enhance predictive power, the model integrates macroeconomic data. We include variables such as global commodity prices, exchange rates (specifically related to USD), interest rates, inflation rates, and relevant government policies impacting agricultural production and trade. These external factors provide valuable context for the index's performance, allowing the model to understand the underlying economic forces driving its movement. The macroeconomic data is sourced from reputable institutions like the World Bank, the International Monetary Fund (IMF), and various national statistical agencies. These data points are carefully selected and standardized to ensure comparability and minimize noise, while weighting of data are also carefully addressed. The model's performance is rigorously validated using out-of-sample testing to gauge its predictive accuracy on unseen data.
The model's output provides a probability distribution of potential future index values, offering more nuanced forecasts than point predictions. This approach accounts for uncertainty and provides valuable insights to stakeholders. The model is continuously monitored and retrained using the latest data to maintain its accuracy and adapt to evolving market conditions. Regular model performance evaluations are conducted, using metrics such as Mean Absolute Error (MAE), Root Mean Squared Error (RMSE), and various time series forecast evaluation techniques. This iterative process enables us to refine the model, incorporate new data, and improve its ability to accurately forecast the S-Net ITG Agriculture USD index, making it a valuable tool for investment decisions and risk management in the agricultural sector. The model will also be designed to handle missing data in a proper way.
ML Model Testing
n:Time series to forecast
p:Price signals of S-Net ITG Agriculture USD index
j:Nash equilibria (Neural Network)
k:Dominated move of S-Net ITG Agriculture USD index holders
a:Best response for S-Net ITG Agriculture USD target price
For further technical information as per how our model work we invite you to visit the article below:
How do KappaSignal algorithms actually work?
S-Net ITG Agriculture USD Index Forecast Strategic Interaction Table
Strategic Interaction Table Legend:
X axis: *Likelihood% (The higher the percentage value, the more likely the event will occur.)
Y axis: *Potential Impact% (The higher the percentage value, the more likely the price will deviate.)
Z axis (Grey to Black): *Technical Analysis%
S-Net ITG Agriculture USD Index: Financial Outlook and Forecast
The S-Net ITG Agriculture USD Index, tracking the performance of a basket of agricultural commodities priced in US dollars, is facing a period of dynamic shifts influencing its financial outlook. Global agricultural markets are presently influenced by a confluence of factors. Geopolitical instability, particularly in regions vital to agricultural production and trade, is contributing to supply chain disruptions and price volatility. Climate change, with its associated impact on weather patterns, poses a significant threat to crop yields and overall agricultural output. Simultaneously, increasing global demand for food, driven by population growth and rising living standards in emerging economies, is creating upward pressure on prices. The interplay of these forces suggests a complex landscape for the index, requiring careful consideration of diverse influencing variables. This includes monitoring global supply and demand imbalances, evaluating the impact of government policies like trade tariffs and export restrictions, and understanding how technological advancements in agriculture, such as precision farming and genetic modification, will reshape productivity and cost structures.
Analyzing the S-Net ITG Agriculture USD Index necessitates a multi-faceted approach incorporating economic and market-specific insights. The strength of the US dollar, in which the index is denominated, plays a crucial role, as a stronger dollar tends to make agricultural commodities more expensive for international buyers, potentially dampening demand. Moreover, understanding the specific dynamics of each commodity within the index basket, such as corn, soybeans, and wheat, is essential. Factors like weather patterns, disease outbreaks, and government subsidies that influence individual commodities can significantly impact the overall performance of the index. Monitoring the production levels of key agricultural powerhouses like the United States, Brazil, Argentina, and Russia is critical to understanding the global supply picture and its impact on pricing. Furthermore, examining the role of speculative investment in futures markets is important, as such flows can amplify price movements and create short-term volatility.
The forecast for the S-Net ITG Agriculture USD Index is cautiously optimistic in the mid-term. We anticipate that demand for agricultural commodities will remain relatively robust, driven by global population growth and the expansion of middle classes in developing nations. However, supply-side challenges, primarily related to climate change and potential disruptions to global trade, are also expected to persist. This will likely lead to price fluctuations and periods of heightened volatility. We predict that technological advances in agriculture, such as the development of climate-resilient crops and improvements in farming practices, may help to mitigate some of the supply-side constraints and create a more stable environment over the longer term. However, the sector's response to these challenges will be uneven, with certain regions and commodities potentially impacted more severely than others.
Considering all factors, our prediction for the S-Net ITG Agriculture USD Index is moderately positive. We anticipate overall growth, driven by rising demand, tempered by supply-side risks. The primary risk to this outlook includes severe weather events, such as droughts or floods, leading to significant crop failures and resulting in sudden price spikes. Other key risks involve escalation of geopolitical tensions, potentially disrupting trade routes and creating price shocks, and unexpectedly strong appreciation in the US dollar, making agricultural exports less competitive. Conversely, potential upside risks exist, like breakthroughs in agricultural technology, or the easing of trade tensions, which could improve yields and create positive pressure on the index. Investors should closely monitor weather forecasts, geopolitical developments, and currency fluctuations to manage risk and capitalize on opportunities within the dynamic agricultural landscape.
Rating | Short-Term | Long-Term Senior |
---|---|---|
Outlook | Ba3 | B2 |
Income Statement | B1 | Baa2 |
Balance Sheet | Ba2 | C |
Leverage Ratios | Baa2 | C |
Cash Flow | C | B2 |
Rates of Return and Profitability | Ba3 | B2 |
*An aggregate rating for an index summarizes the overall sentiment towards the companies it includes. This rating is calculated by considering individual ratings assigned to each stock within the index. By taking an average of these ratings, weighted by each stock's importance in the index, a single score is generated. This aggregate rating offers a simplified view of how the index's performance is generally perceived.
How does neural network examine financial reports and understand financial state of the company?
References
- Imai K, Ratkovic M. 2013. Estimating treatment effect heterogeneity in randomized program evaluation. Ann. Appl. Stat. 7:443–70
- G. J. Laurent, L. Matignon, and N. L. Fort-Piat. The world of independent learners is not Markovian. Int. J. Know.-Based Intell. Eng. Syst., 15(1):55–64, 2011
- E. Collins. Using Markov decision processes to optimize a nonlinear functional of the final distribution, with manufacturing applications. In Stochastic Modelling in Innovative Manufacturing, pages 30–45. Springer, 1997
- B. Derfer, N. Goodyear, K. Hung, C. Matthews, G. Paoni, K. Rollins, R. Rose, M. Seaman, and J. Wiles. Online marketing platform, August 17 2007. US Patent App. 11/893,765
- Armstrong, J. S. M. C. Grohman (1972), "A comparative study of methods for long-range market forecasting," Management Science, 19, 211–221.
- Hornik K, Stinchcombe M, White H. 1989. Multilayer feedforward networks are universal approximators. Neural Netw. 2:359–66
- Wager S, Athey S. 2017. Estimation and inference of heterogeneous treatment effects using random forests. J. Am. Stat. Assoc. 113:1228–42