AUC Score :
Short-term Tactic1 :
Dominant Strategy :
Time series to forecast n:
ML Model Testing : Inductive Learning (ML)
Hypothesis Testing : Logistic Regression
Surveillance : Major exchange and OTC
1Short-term revised.
2Time series is updated based on short-term trends.
Key Points
Red Violet's future prospects appear cautiously optimistic. Predictions suggest potential revenue growth fueled by increased demand for its data analytics and risk mitigation services, possibly leading to improved profitability. Expansion into new markets and strategic partnerships could further boost the company's financial performance. However, significant risks exist. The company faces intense competition within the data analytics sector, and market saturation could limit growth. Economic downturns could negatively impact demand for Red Violet's services, while data privacy regulations and changing customer preferences could lead to decreased revenue. Any cybersecurity breaches or data integrity concerns may damage the company's reputation and erode investor confidence. Furthermore, potential challenges with integrating acquisitions or failing to capitalize on technological advancements could also hinder long-term growth and market position.About Red Violet
RVVT is a technology company specializing in data analytics and information solutions. They provide a suite of services that help businesses and government agencies identify, understand, and connect with people and their associated information. Their core offerings include data-driven insights, risk assessment, and identity verification solutions, leveraging a vast database of public and proprietary records. The company focuses on helping clients make informed decisions through sophisticated data analysis and comprehensive reporting capabilities.
Their products serve various industries, including financial services, insurance, and law enforcement. RVVT emphasizes the accuracy and comprehensiveness of their data, aiming to deliver actionable intelligence to its customers. The company also prioritizes data privacy and compliance, ensuring that their services are used responsibly and ethically. RVVT strives to continuously innovate and enhance its offerings to meet the evolving needs of its clients and the data-driven landscape.
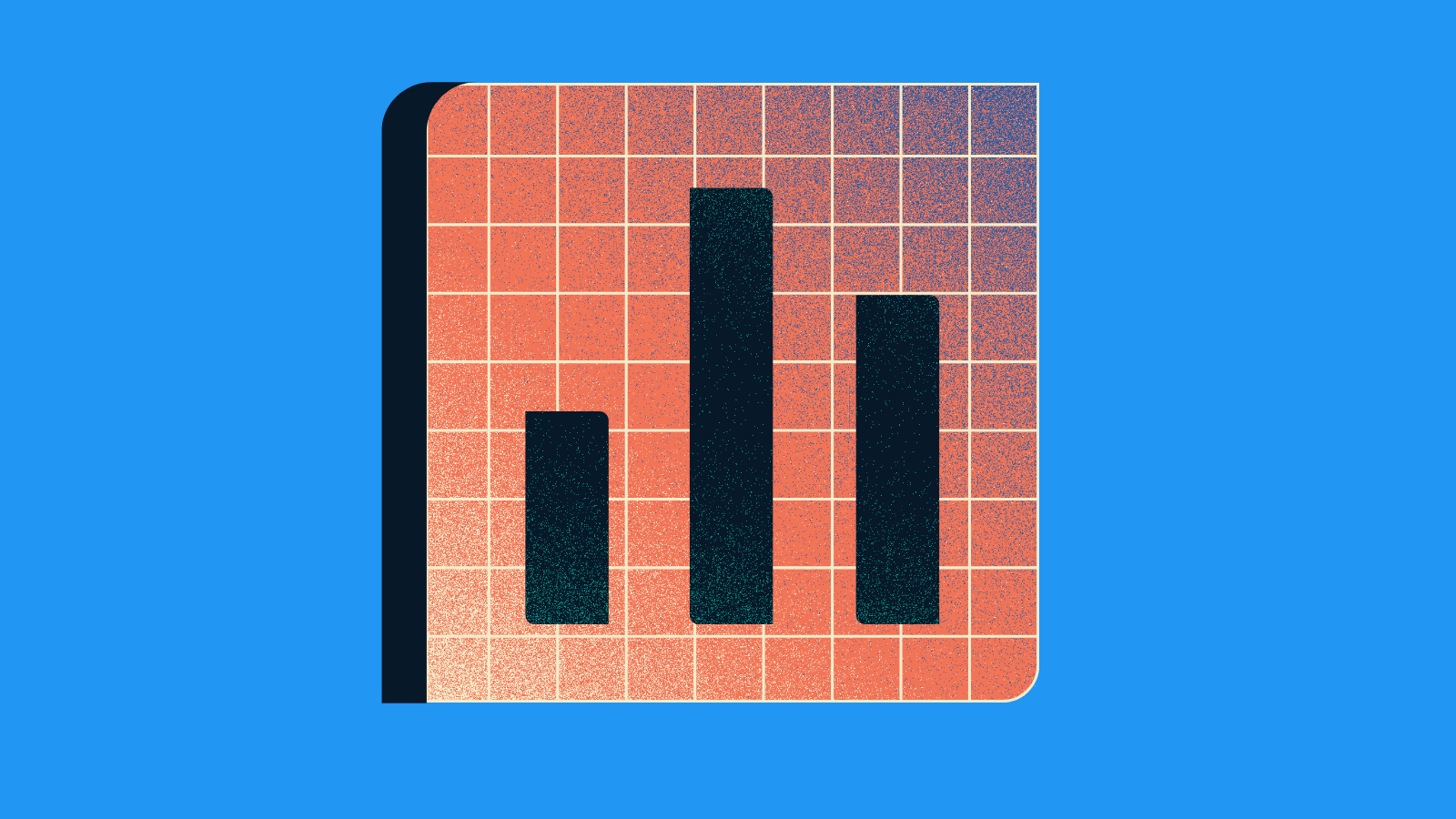
RDVT Stock Forecast Model: A Data Science and Economic Approach
The RDVT stock forecast model leverages a multifaceted approach, combining the power of machine learning with economic insights. We employ a time series analysis incorporating historical stock performance data, including trading volumes, volatility measures, and moving averages, as foundational features. Furthermore, we integrate macroeconomic indicators such as GDP growth, inflation rates, and interest rate levels, acknowledging their significant impact on market sentiment and investor behavior. Textual data from news articles and financial reports related to Red Violet Inc., as well as competitor analysis, are analyzed using Natural Language Processing (NLP) techniques to gauge sentiment and incorporate qualitative factors. Feature engineering is crucial, transforming raw data into informative variables; for example, creating lagged variables, calculating technical indicators, and incorporating sentiment scores to capture temporal dependencies and market dynamics.
We evaluate several machine learning algorithms, including Recurrent Neural Networks (RNNs), specifically LSTMs, and Gradient Boosting Machines (GBMs), due to their effectiveness in time series prediction and non-linear relationships. Hyperparameter tuning is performed using techniques like cross-validation to optimize model performance, and we assess model accuracy using metrics like Mean Squared Error (MSE), Root Mean Squared Error (RMSE), and Mean Absolute Error (MAE). Additionally, we implement techniques such as early stopping and regularization to prevent overfitting. Model interpretation techniques, such as feature importance analysis, provide insights into the key drivers behind the model's predictions, enabling us to understand which factors most influence the stock's future behavior.
The final model will produce a forecast horizon, such as a rolling one-year period, with periodic updates to incorporate new data. Our team will perform ongoing monitoring and evaluation, including backtesting to assess the model's historical accuracy and stability. We recognize the inherent uncertainty in stock forecasting, thus, the model's outputs will be presented with a confidence interval to reflect potential prediction errors. A dashboard provides an interface for stakeholders to access and understand the forecast, including sensitivity analysis to demonstrate the impact of different factors. This model is a dynamic instrument designed to deliver actionable intelligence, which is further enhanced through continual refinement and adaptation to ever-evolving market dynamics.
ML Model Testing
n:Time series to forecast
p:Price signals of Red Violet stock
j:Nash equilibria (Neural Network)
k:Dominated move of Red Violet stock holders
a:Best response for Red Violet target price
For further technical information as per how our model work we invite you to visit the article below:
How do KappaSignal algorithms actually work?
Red Violet Stock Forecast (Buy or Sell) Strategic Interaction Table
Strategic Interaction Table Legend:
X axis: *Likelihood% (The higher the percentage value, the more likely the event will occur.)
Y axis: *Potential Impact% (The higher the percentage value, the more likely the price will deviate.)
Z axis (Grey to Black): *Technical Analysis%
Financial Outlook and Forecast for Red Violet Inc.
The financial trajectory of Red Violet, specializing in data and analytics solutions, presents a complex picture. Revenue growth is expected to be driven by an increasing demand for data-driven insights across various industries, particularly within risk management and fraud prevention. RV's core products, which include identity verification and due diligence solutions, are likely to maintain strong demand. However, the competitive landscape is also fierce, with established players and emerging startups vying for market share. RV's success will depend on its ability to differentiate its offerings through innovation, strategic partnerships, and effective customer acquisition and retention strategies. The company's performance will be heavily influenced by the overall economic environment and the willingness of businesses to invest in data analytics tools.
Profitability prospects are subject to several influencing factors. RV's profitability is expected to benefit from operating leverage as its revenue expands. This means the company can increase profits without proportionally increasing costs. RV's ability to control its operational expenses, particularly in areas such as research and development, sales and marketing, and general administration, is important. The efficiency of RV's sales force and the effectiveness of its marketing initiatives are also critical factors in determining its path to profitability. The company may also consider strategic acquisitions or partnerships, which could further impact its cost structure and overall financial performance. Investors should also closely follow the company's debt levels and its ability to manage its balance sheet responsibly.
Analyzing Red Violet's future financial performance relies on the company's strategic positioning. Expansion into adjacent markets or developing new products could accelerate revenue growth. Furthermore, successful integration of acquired businesses and the establishment of strategic partnerships could create synergistic opportunities. However, the reliance on a limited number of key customers could expose RV to concentration risks. A loss of a major customer would likely have a significant negative impact on revenue. Investors should scrutinize the company's research and development investments, as these will influence its capacity to stay ahead of industry trends and launch new, valuable products. Changes in regulations regarding data privacy and security also present an opportunity or challenge.
In summary, the outlook for RV appears cautiously optimistic. While the company operates in a promising market, it is imperative to acknowledge several risks. A predicted sustained demand for data analytics tools and RV's strong offerings give a positive signal. However, this forecast is not without its risks. The intense competitive environment, the need for sustained product innovation, and potential economic downturns could negatively influence the company's performance. Investors should monitor RV's progress on operational efficiencies, customer retention, and the ability to successfully integrate new products and services, while also staying vigilant about evolving regulations.
```
Rating | Short-Term | Long-Term Senior |
---|---|---|
Outlook | Baa2 | Baa2 |
Income Statement | Baa2 | Ba3 |
Balance Sheet | Ba3 | Baa2 |
Leverage Ratios | B3 | Ba1 |
Cash Flow | Baa2 | Baa2 |
Rates of Return and Profitability | Baa2 | Ba2 |
*Financial analysis is the process of evaluating a company's financial performance and position by neural network. It involves reviewing the company's financial statements, including the balance sheet, income statement, and cash flow statement, as well as other financial reports and documents.
How does neural network examine financial reports and understand financial state of the company?
References
- Artis, M. J. W. Zhang (1990), "BVAR forecasts for the G-7," International Journal of Forecasting, 6, 349–362.
- V. Borkar. Q-learning for risk-sensitive control. Mathematics of Operations Research, 27:294–311, 2002.
- Blei DM, Lafferty JD. 2009. Topic models. In Text Mining: Classification, Clustering, and Applications, ed. A Srivastava, M Sahami, pp. 101–24. Boca Raton, FL: CRC Press
- Imbens G, Wooldridge J. 2009. Recent developments in the econometrics of program evaluation. J. Econ. Lit. 47:5–86
- Christou, C., P. A. V. B. Swamy G. S. Tavlas (1996), "Modelling optimal strategies for the allocation of wealth in multicurrency investments," International Journal of Forecasting, 12, 483–493.
- Bengio Y, Schwenk H, SenĂ©cal JS, Morin F, Gauvain JL. 2006. Neural probabilistic language models. In Innovations in Machine Learning: Theory and Applications, ed. DE Holmes, pp. 137–86. Berlin: Springer
- Kitagawa T, Tetenov A. 2015. Who should be treated? Empirical welfare maximization methods for treatment choice. Tech. Rep., Cent. Microdata Methods Pract., Inst. Fiscal Stud., London