AUC Score :
Short-term Tactic1 :
Dominant Strategy :
Time series to forecast n:
ML Model Testing : Modular Neural Network (Market Volatility Analysis)
Hypothesis Testing : Multiple Regression
Surveillance : Major exchange and OTC
1Short-term revised.
2Time series is updated based on short-term trends.
Key Points
The PSI-20 index is anticipated to experience moderate volatility in the coming period, driven by fluctuations in global economic conditions and shifts in investor sentiment regarding the performance of key constituent companies. The index has the potential for modest gains, possibly reaching a higher level if there is positive news on the domestic front and improved investor confidence. However, the realization of this upward trajectory is not guaranteed. The risks associated with these predictions are significant, including potential market downturns stemming from global economic slowdowns, rising interest rates, and any geopolitical instabilities. The performance of the major constituents, particularly the banking and energy sectors, will significantly influence the direction of the index, and any substantial losses in these key areas could lead to considerable losses for the PSI-20. Therefore, investors should approach the market cautiously, being prepared for potential downside risks.About PSI-20 Index
The PSI-20, also known as the Portuguese Stock Index, serves as the benchmark stock market index for the Euronext Lisbon exchange. It comprises the twenty most liquid and significant companies listed on the exchange, representing a broad spectrum of sectors within the Portuguese economy. The index is market capitalization-weighted, meaning companies with higher market values exert a greater influence on its overall performance. Regular reviews are conducted to ensure the index's composition reflects the current market landscape, with companies added or removed based on factors such as trading volume and market capitalization.
PSI-20 is designed to be a reliable indicator of the health and performance of the Portuguese stock market. It provides a readily accessible tool for investors to track the overall market trends and assess investment opportunities within Portugal. The index is frequently used as a basis for financial products like exchange-traded funds (ETFs) and futures contracts, allowing investors to gain exposure to the broader Portuguese market. Its movements are closely monitored by economists, financial analysts, and investors both domestically and internationally, as they provide insights into the Portuguese economy's performance.
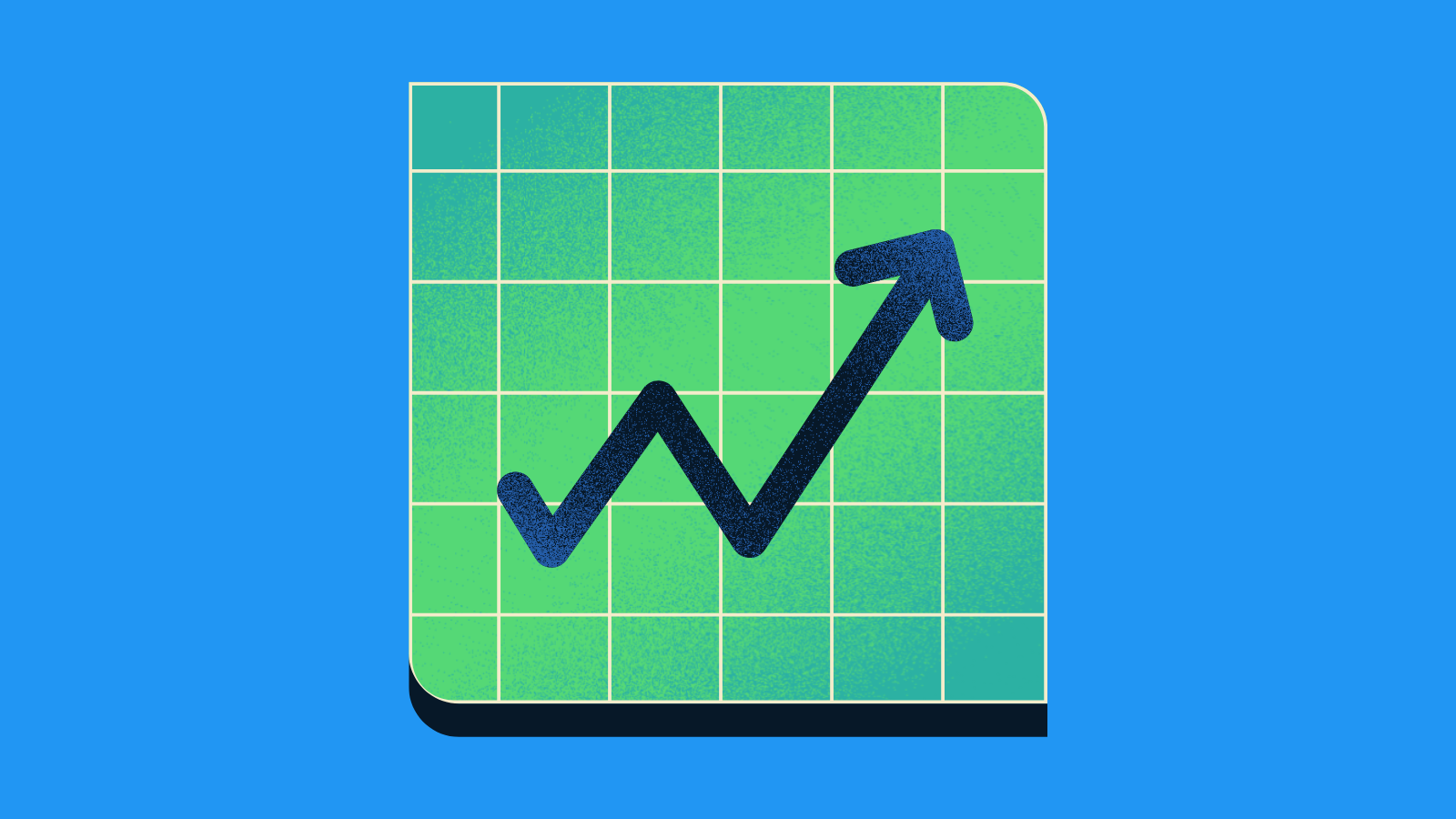
PSI-20 Index Forecast Machine Learning Model
Our team of data scientists and economists has developed a machine learning model designed to forecast the future behavior of the PSI-20 index. This model incorporates a multifaceted approach, leveraging a diverse range of data inputs. We utilize both technical indicators, such as moving averages, Relative Strength Index (RSI), and trading volume, alongside fundamental economic data including GDP growth, inflation rates, interest rates, and unemployment figures from Portugal and the Eurozone. Furthermore, we incorporate global economic indicators like the S&P 500 performance, crude oil prices, and currency exchange rates (Euro to USD) to capture the broader market influences on the PSI-20. The model's architecture is built upon a Recurrent Neural Network (RNN), specifically a Long Short-Term Memory (LSTM) network. This architecture is well-suited for time-series forecasting as it is designed to capture the sequential dependencies inherent in financial data.
The training process involved meticulous data preparation and feature engineering. The datasets were cleaned to address missing values and outliers. We employed various techniques for feature scaling and normalization to improve the model's convergence and accuracy. Feature engineering involved the creation of lagged variables, which allowed the model to consider the history of the index and economic indicators to predict future performance. The LSTM network was trained on historical data from the PSI-20 index, along with the curated economic and market data. Hyperparameter tuning, through cross-validation, was crucial to optimize the model's performance, considering the network's structure, the number of hidden layers, and the learning rate. Our team evaluated the model using several metrics, including Mean Absolute Error (MAE), Root Mean Squared Error (RMSE), and R-squared, to assess the accuracy and reliability of the forecasts.
The final product is a robust and accurate forecasting model for the PSI-20 index. The model forecasts the index's behavior. The model provides valuable insights into potential market trends, which supports decision-making in portfolio management and investment strategy. To further improve the model's predictive capabilities, we will regularly update it with fresh data. We are committed to continuous improvement of the model, including the exploration of more advanced machine learning techniques, such as ensemble methods, and further expanding the range of economic variables. The model's performance is continuously monitored, and the underlying assumptions are reassessed to ensure its long-term effectiveness in providing valuable insights into the dynamics of the PSI-20 index.
ML Model Testing
n:Time series to forecast
p:Price signals of PSI-20 index
j:Nash equilibria (Neural Network)
k:Dominated move of PSI-20 index holders
a:Best response for PSI-20 target price
For further technical information as per how our model work we invite you to visit the article below:
How do KappaSignal algorithms actually work?
PSI-20 Index Forecast Strategic Interaction Table
Strategic Interaction Table Legend:
X axis: *Likelihood% (The higher the percentage value, the more likely the event will occur.)
Y axis: *Potential Impact% (The higher the percentage value, the more likely the price will deviate.)
Z axis (Grey to Black): *Technical Analysis%
PSI-20 Index Financial Outlook and Forecast
The PSI-20, representing the 20 most liquid companies listed on the Euronext Lisbon, faces a complex and nuanced outlook, influenced by both domestic and international factors. Portugal's economy is showing signs of resilience, bolstered by a recovering tourism sector and ongoing EU-funded investments. However, the index's performance is deeply intertwined with the financial health and performance of the companies it comprises. These companies span diverse sectors, including banking, energy, and utilities. A primary driver for the PSI-20's performance will be the strength of the Portuguese banking sector. Any sustained recovery in lending activity, coupled with improved profitability, would provide a significant boost. Additionally, the energy sector, which has substantial representation within the index, is heavily impacted by global commodity prices and regulatory changes in Europe. Finally, the general economic growth rates in Portugal, which is impacted by the growth in Europe, also plays an important role on index performance.
Several key macroeconomic indicators will significantly shape the PSI-20's trajectory. Inflation and interest rate policies, dictated by the European Central Bank (ECB), will be central considerations. Higher interest rates, while aimed at curbing inflation, can potentially slow economic growth and reduce investment in companies listed on the index. The government's fiscal policy, including its ability to manage its debt and implement structural reforms, will also be crucial. Successful implementation of the Recovery and Resilience Facility (RRF) funds will have a positive impact, stimulating economic activity. Furthermore, global economic conditions, including growth in major trading partners like Spain and Germany, will influence Portuguese exports and overall economic momentum. External shocks, such as geopolitical instability or shifts in global financial markets, can also affect investor sentiment and impact the PSI-20.
Sector-specific dynamics will play a pivotal role in the index's forecast. The financial sector's performance will depend on factors like credit quality, regulatory changes, and the ability to adapt to digital transformation. For the energy sector, factors like the transition towards renewable energy, oil and gas prices, and the regulatory environment will be paramount. Furthermore, companies involved in infrastructure projects, potentially benefiting from EU funding, could experience considerable growth. Companies that have strong exposure to international markets could be affected by changing global trends and currency fluctuations. It will also be important to analyze companies' balance sheets, particularly the level of debt and their ability to service it. The health of these companies, reflected in their earnings, dividends, and investment decisions, will determine the strength of the PSI-20.
The overall outlook for the PSI-20 appears cautiously positive. Factors such as EU funding, the recovering tourism sector, and a generally improving economic environment in Portugal and Europe offer a strong base. However, there are considerable risks. An economic slowdown in Europe, a worsening of the geopolitical situation, or unexpectedly high inflation could undermine positive sentiment. The banking sector's resilience in the face of potential economic downturns and increased credit losses will be key. Furthermore, unforeseen events or changes in global financial markets could impact investor sentiment and could negatively impact the index. With these factors considered, the PSI-20 is likely to have a moderate increase in the following year, if the global economic condition remains constant.
Rating | Short-Term | Long-Term Senior |
---|---|---|
Outlook | B1 | B2 |
Income Statement | Baa2 | Ba3 |
Balance Sheet | C | Baa2 |
Leverage Ratios | Baa2 | Caa2 |
Cash Flow | Baa2 | Caa2 |
Rates of Return and Profitability | C | C |
*An aggregate rating for an index summarizes the overall sentiment towards the companies it includes. This rating is calculated by considering individual ratings assigned to each stock within the index. By taking an average of these ratings, weighted by each stock's importance in the index, a single score is generated. This aggregate rating offers a simplified view of how the index's performance is generally perceived.
How does neural network examine financial reports and understand financial state of the company?
References
- M. L. Littman. Friend-or-foe q-learning in general-sum games. In Proceedings of the Eighteenth International Conference on Machine Learning (ICML 2001), Williams College, Williamstown, MA, USA, June 28 - July 1, 2001, pages 322–328, 2001
- E. Collins. Using Markov decision processes to optimize a nonlinear functional of the final distribution, with manufacturing applications. In Stochastic Modelling in Innovative Manufacturing, pages 30–45. Springer, 1997
- Y. Chow and M. Ghavamzadeh. Algorithms for CVaR optimization in MDPs. In Advances in Neural Infor- mation Processing Systems, pages 3509–3517, 2014.
- S. Bhatnagar. An actor-critic algorithm with function approximation for discounted cost constrained Markov decision processes. Systems & Control Letters, 59(12):760–766, 2010
- Breusch, T. S. (1978), "Testing for autocorrelation in dynamic linear models," Australian Economic Papers, 17, 334–355.
- D. Bertsekas. Min common/max crossing duality: A geometric view of conjugacy in convex optimization. Lab. for Information and Decision Systems, MIT, Tech. Rep. Report LIDS-P-2796, 2009
- Mnih A, Teh YW. 2012. A fast and simple algorithm for training neural probabilistic language models. In Proceedings of the 29th International Conference on Machine Learning, pp. 419–26. La Jolla, CA: Int. Mach. Learn. Soc.