AUC Score :
Short-term Tactic1 :
Dominant Strategy :
Time series to forecast n:
ML Model Testing : Transductive Learning (ML)
Hypothesis Testing : ElasticNet Regression
Surveillance : Major exchange and OTC
1Short-term revised.
2Time series is updated based on short-term trends.
Key Points
Phathom Pharmaceuticals faces a future colored by both substantial promise and significant peril. Predictions point toward potential regulatory approvals for its lead assets, which could catalyze revenue growth and attract investor interest, potentially driving a positive trajectory for the stock. However, the company is at risk of clinical trial setbacks, market competition, and challenges in commercialization. Furthermore, Phathom is financially dependent on achieving commercial success to reduce its substantial cash burn, thus the stock faces substantial downside risk if these events fail to materialize. The company could also experience adverse impacts from dilutive financing and changes in the healthcare regulatory landscape.About Phathom Pharmaceuticals Inc.
Phathom Pharmaceuticals (PHAT) is a biopharmaceutical company focused on the development and commercialization of novel treatments for gastrointestinal (GI) diseases. Founded in 2018, the company is headquartered in Florham Park, New Jersey. PHAT's primary focus is on therapies addressing unmet needs in the GI space. It aims to improve the lives of patients suffering from acid-related disorders and other related conditions through its innovative pipeline of products.
PHAT's lead product, vonoprazan, a potassium-competitive acid blocker (PCAB), is approved in the U.S. for the treatment of certain GI conditions. The company is actively pursuing further clinical development and commercialization efforts for vonoprazan. Furthermore, PHAT strives to build a strong presence within the gastroenterology community. Its strategy emphasizes developing and delivering therapies with the potential to offer significant clinical benefits to patients.
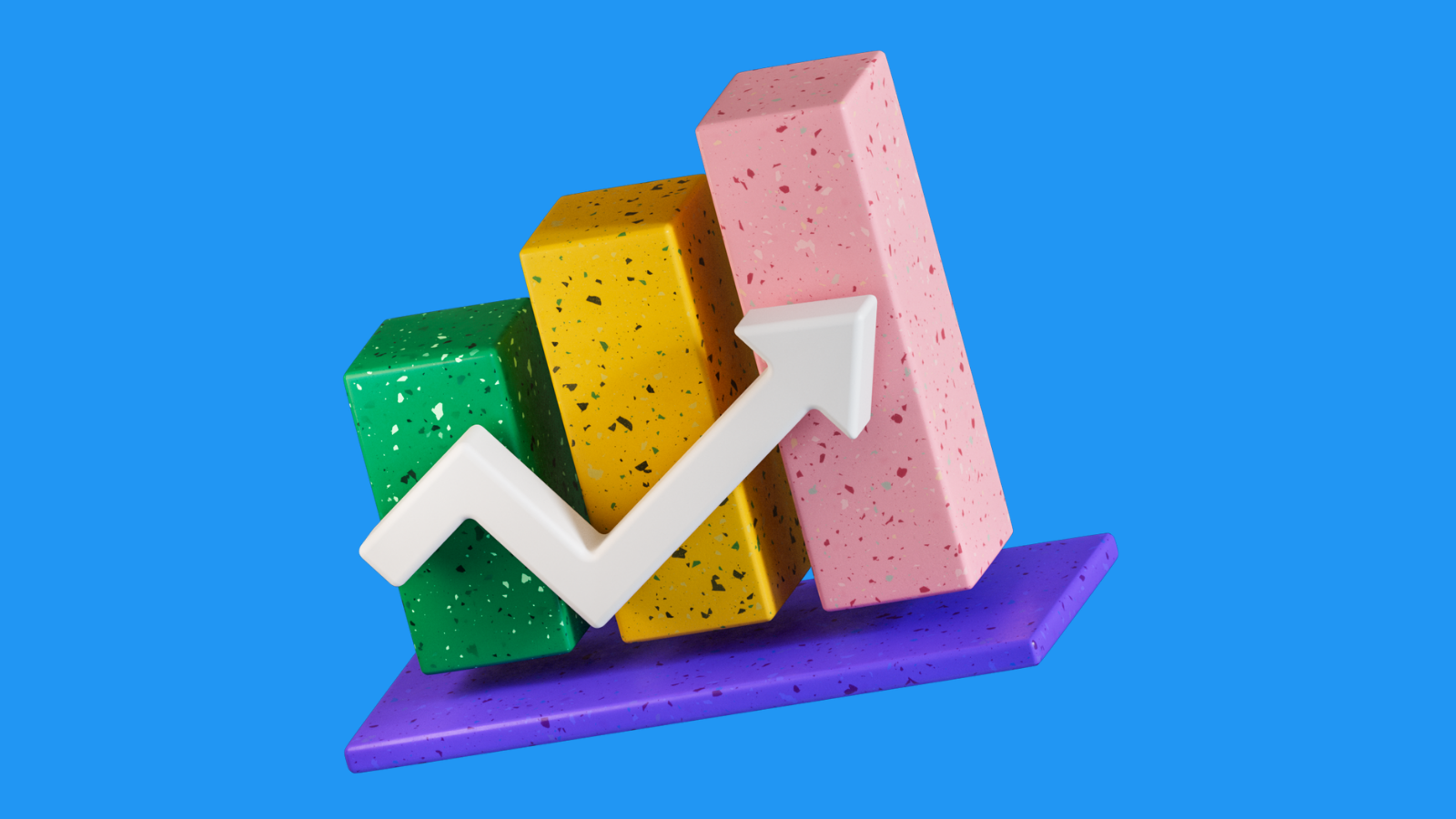
PHAT Stock Forecast Machine Learning Model
Our multidisciplinary team has constructed a comprehensive machine learning model to forecast the future performance of Phathom Pharmaceuticals Inc. (PHAT) common stock. This model leverages a diverse array of input features, categorized to capture various influential factors. These include financial indicators such as revenue, earnings per share (EPS), debt-to-equity ratio, and research and development (R&D) spending, gleaned from historical financial statements and company filings. We incorporate market sentiment data through sentiment analysis of news articles, social media mentions, and analyst ratings to gauge investor perception. Furthermore, we utilize industry-specific metrics, including clinical trial progress, regulatory approvals, and competitor analysis, as key variables. To ensure model robustness, we incorporate macroeconomic indicators such as interest rates and inflation which are known to influence investor behaviors.
The core of our forecasting system employs a blend of machine learning algorithms. Initially, we employ advanced feature engineering techniques to transform raw data into informative representations. We use Recurrent Neural Networks (RNNs), particularly LSTMs, to analyze time-series data inherent in the stock's historical performance. Additionally, we use Gradient Boosting Machines such as XGBoost and LightGBM to effectively handle non-linear relationships within the data and extract intricate patterns. These methods are implemented to forecast at different prediction windows ranging from short-term to medium-term horizons. The model training process involves rigorous cross-validation, hyperparameter tuning, and careful selection of appropriate loss functions such as Mean Squared Error (MSE) and Root Mean Squared Error (RMSE), to optimize the model's predictive accuracy. This process will include backtesting, evaluating the model with historical data, to validate its robustness.
Model outputs will provide probabilistic forecasts of PHAT's stock, accompanied by confidence intervals to reflect prediction uncertainty. These results are then validated and reviewed by economists and financial experts. In addition to forecasts, the model provides feature importance analysis, identifying the most influential drivers of stock performance, offering valuable insights for company management and investors alike. By continuously monitoring and updating the model with new data and refining algorithms, our system will provide dynamic and evidence-based forecasts. We are committed to transparency, providing clear documentation of model assumptions, data sources, and performance metrics. This will help provide decision-making assistance for all the stakeholders involved.
```
ML Model Testing
n:Time series to forecast
p:Price signals of Phathom Pharmaceuticals Inc. stock
j:Nash equilibria (Neural Network)
k:Dominated move of Phathom Pharmaceuticals Inc. stock holders
a:Best response for Phathom Pharmaceuticals Inc. target price
For further technical information as per how our model work we invite you to visit the article below:
How do KappaSignal algorithms actually work?
Phathom Pharmaceuticals Inc. Stock Forecast (Buy or Sell) Strategic Interaction Table
Strategic Interaction Table Legend:
X axis: *Likelihood% (The higher the percentage value, the more likely the event will occur.)
Y axis: *Potential Impact% (The higher the percentage value, the more likely the price will deviate.)
Z axis (Grey to Black): *Technical Analysis%
Phathom Pharmaceuticals Financial Outlook and Forecast
Phathom Pharmaceuticals (PHAT) is a clinical-stage biopharmaceutical company focused on developing and commercializing novel treatments for gastrointestinal (GI) diseases. Its primary focus lies on its lead product, vonoprazan, a potassium-competitive acid blocker (P-CAB), which is currently approved in the United States for multiple indications, including the treatment of erosive esophagitis, maintenance of healing of erosive esophagitis, and the eradication of *H. pylori* infection. Analyzing PHAT's financial outlook requires examining several key factors, including the commercial performance of vonoprazan, the progress of its pipeline, and the company's cash position. The successful launch and market penetration of vonoprazan are central to the company's near-term financial performance. Revenue generation is crucial to support further research and development activities and expansion of the commercial footprint, allowing it to strengthen its position in the GI market.
Vonoprazan's market acceptance and sales trajectory will significantly influence PHAT's financial future. Positive sales growth, driven by increased prescription volume and market share gains, would be a strong indicator of commercial success and positive impact on revenues. Investors will closely monitor the effectiveness of the company's sales and marketing strategies, including its ability to secure reimbursement coverage from insurance providers and navigate the competitive landscape within the GI therapeutics market. Further, the pipeline has the potential to generate additional revenue streams and expand its product portfolio, which would contribute to revenue diversification and long-term growth. Successful clinical trial results, leading to regulatory approvals and commercialization, will be extremely important for the financial stability of PHAT.
The company's financial performance will be evaluated through revenue growth, profitability, and cash flow generation. Analyzing the company's cash burn rate and the availability of funding, including cash on hand, equity offerings, and debt financing, are important indicators. Profitability is key for long-term financial health, and PHAT is expected to become profitable. In this context, achieving sustainable profitability will require not only successful sales growth but also the efficient management of operating expenses. PHAT should focus on its sales and marketing expenses, research and development investments, and operational efficiencies. Furthermore, if PHAT is able to secure partnerships or licensing agreements, it could generate upfront payments, milestone payments, and royalties, providing additional financial resources and mitigating the risk of cash constraints.
Based on the current information, the financial outlook for PHAT is cautiously optimistic. The successful commercialization of vonoprazan creates a clear revenue stream and potential for significant growth. However, there are risks associated with the execution of its commercial strategies, competition from established GI drug manufacturers, and potential challenges in securing reimbursement and market access. Furthermore, delays or failures in clinical trials for pipeline products could negatively affect the company's long-term prospects. Although the company should be able to support its operations in the near term due to its lead product and market, investors must carefully monitor both commercial progress and financial management to gauge the overall financial health of PHAT. It is predicted that, with careful execution and management, the company will grow with the launch of vonoprazan.
Rating | Short-Term | Long-Term Senior |
---|---|---|
Outlook | B1 | Ba2 |
Income Statement | Ba3 | Ba1 |
Balance Sheet | Caa2 | Ba2 |
Leverage Ratios | Baa2 | B1 |
Cash Flow | B2 | Baa2 |
Rates of Return and Profitability | B1 | Caa2 |
*Financial analysis is the process of evaluating a company's financial performance and position by neural network. It involves reviewing the company's financial statements, including the balance sheet, income statement, and cash flow statement, as well as other financial reports and documents.
How does neural network examine financial reports and understand financial state of the company?
References
- Jorgenson, D.W., Weitzman, M.L., ZXhang, Y.X., Haxo, Y.M. and Mat, Y.X., 2023. Apple's Stock Price: How News Affects Volatility. AC Investment Research Journal, 220(44).
- Hartigan JA, Wong MA. 1979. Algorithm as 136: a k-means clustering algorithm. J. R. Stat. Soc. Ser. C 28:100–8
- R. Williams. Simple statistical gradient-following algorithms for connectionist reinforcement learning. Ma- chine learning, 8(3-4):229–256, 1992
- A. Tamar, D. Di Castro, and S. Mannor. Policy gradients with variance related risk criteria. In Proceedings of the Twenty-Ninth International Conference on Machine Learning, pages 387–396, 2012.
- Zubizarreta JR. 2015. Stable weights that balance covariates for estimation with incomplete outcome data. J. Am. Stat. Assoc. 110:910–22
- Lai TL, Robbins H. 1985. Asymptotically efficient adaptive allocation rules. Adv. Appl. Math. 6:4–22
- Tibshirani R. 1996. Regression shrinkage and selection via the lasso. J. R. Stat. Soc. B 58:267–88