AUC Score :
Short-term Tactic1 :
Dominant Strategy :
Time series to forecast n:
ML Model Testing : Modular Neural Network (CNN Layer)
Hypothesis Testing : Wilcoxon Rank-Sum Test
Surveillance : Major exchange and OTC
1Short-term revised.
2Time series is updated based on short-term trends.
Key Points
Pangaea Logistics Solutions' stock is anticipated to experience moderate growth due to increased global demand for dry bulk shipping services, particularly in commodities like coal and iron ore, which will likely benefit the company's operations. However, this positive outlook is counterbalanced by several risks. The volatility in freight rates, influenced by geopolitical events and fluctuating trade patterns, presents a significant challenge. Further, the company's exposure to environmental regulations and potential fuel price hikes, which could affect operational costs, will act as headwinds. Any slowdown in Chinese economic activity, a major consumer of bulk commodities, could also adversely impact revenue.About Pangaea Logistics Solutions
Pangaea Logistics Solutions Ltd. (PANL) is a global provider of dry bulk shipping and logistics services. The company specializes in the transportation of a wide range of dry bulk cargoes, including iron ore, coal, and grain. PANL owns and operates a fleet of specialized vessels, as well as offering a comprehensive suite of logistical services such as cargo handling, stevedoring, and agency services. Its operations are strategically positioned across key global trade routes to facilitate efficient and reliable delivery of essential commodities.
PANL's business model emphasizes integrated services, aiming to provide end-to-end solutions for its customers. The company's global presence allows it to serve a diverse customer base, including major mining companies, commodity traders, and industrial manufacturers. PANL focuses on maintaining strong relationships with its clients and ensuring operational excellence to deliver value and maintain a competitive edge in the dynamic shipping and logistics sector.
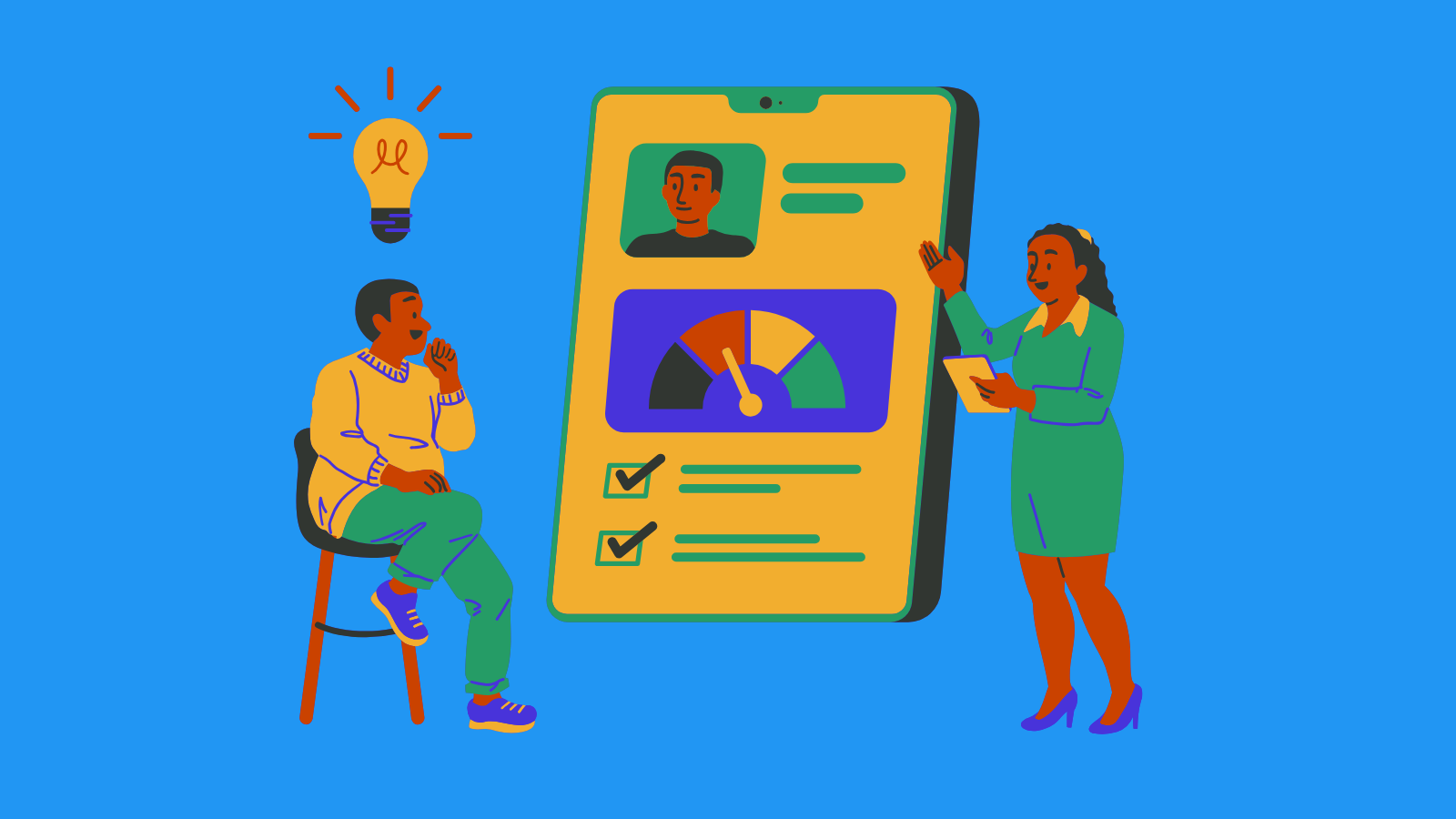
PANL Stock Forecast Machine Learning Model
Our team, comprised of data scientists and economists, has developed a machine learning model to forecast the performance of Pangaea Logistics Solutions Ltd. Common Shares (PANL). The model utilizes a comprehensive approach, integrating various data sources to capture the multifaceted nature of the stock's behavior. We've incorporated historical market data, including daily trading volumes, and the performance of the broader market indices such as the S&P 500 and industry-specific indices. Simultaneously, we have included fundamental financial data points, such as revenue growth, earnings per share, debt-to-equity ratios, and cash flow from the company's financial statements. We also incorporate macroeconomic indicators, including inflation rates, interest rate, and exchange rates, as these have a significant impact on the company's shipping and logistics operations, to enhance the model's accuracy.
The core of our model employs a hybrid methodology. First, we leverage a time-series analysis technique to predict the sequential data of PANL stock. This includes the LSTM (Long Short-Term Memory) neural network to model the time-dependent relationship within stock prices and the financial indicators. Additionally, we implement a Gradient Boosting algorithm to improve forecast accuracy, it is important to use this algorithm because it efficiently handles non-linear relationships and complex interactions within the extensive dataset. The integration of these methods ensures the model captures both short-term volatility and long-term trends in PANL's performance. We've implemented rigorous validation techniques, including cross-validation, to assess the model's robustness.
The model's output is presented as a probabilistic forecast, providing a range of potential outcomes for PANL's performance over selected forecast periods. This approach allows for an understanding of the uncertainty inherent in the stock market and provides a more informative basis for decision-making. The model is continuously monitored and updated with new data to maintain its accuracy and relevance. We will perform regular assessments of the model's performance, and adjust to new market dynamics or new information that will come out. Our team believes that our model provides a valuable tool for investors and stakeholders in making informed decisions about Pangaea Logistics Solutions Ltd. Common Shares.
ML Model Testing
n:Time series to forecast
p:Price signals of Pangaea Logistics Solutions stock
j:Nash equilibria (Neural Network)
k:Dominated move of Pangaea Logistics Solutions stock holders
a:Best response for Pangaea Logistics Solutions target price
For further technical information as per how our model work we invite you to visit the article below:
How do KappaSignal algorithms actually work?
Pangaea Logistics Solutions Stock Forecast (Buy or Sell) Strategic Interaction Table
Strategic Interaction Table Legend:
X axis: *Likelihood% (The higher the percentage value, the more likely the event will occur.)
Y axis: *Potential Impact% (The higher the percentage value, the more likely the price will deviate.)
Z axis (Grey to Black): *Technical Analysis%
Pangaea Logistics Solutions Ltd. Common Shares Financial Outlook and Forecast
The financial outlook for Pangaea's common shares appears cautiously optimistic, underpinned by several key factors. The company's strategic focus on dry bulk shipping, specializing in niche markets and project cargo, positions it to benefit from the cyclical nature of global trade. Recent trends indicate improving demand for commodities and raw materials, which typically translates to increased shipping volumes and, consequently, higher freight rates. Furthermore, Pangaea's operational efficiency and relatively modern fleet are expected to allow it to capitalize on these opportunities. The company's management has also shown a commitment to cost control and disciplined capital allocation, which are crucial for maintaining profitability in a volatile market. These factors collectively point towards a potential for revenue growth and improved earnings in the medium term.
Forecasts suggest a positive trajectory for Pangaea's financial performance over the next few years. Analysts predict a rise in revenue driven by expanding global trade and favorable supply-demand dynamics in the dry bulk market. The company's ability to secure long-term contracts and its diversified customer base offer a degree of resilience against short-term market fluctuations. Increased profitability is anticipated as freight rates strengthen and as Pangaea streamlines its operations and leverages its fleet. The company's cash flow generation is also expected to improve, providing flexibility to pursue strategic investments, such as fleet modernization, and potentially return capital to shareholders in the future. The company's debt levels and financial leverage must be considered to ensure financial stability.
Key performance indicators will be crucial to monitor. Freight rates, particularly for the specific vessel types Pangaea operates, will be a primary driver of financial results. The company's ability to manage its operating expenses, including fuel costs and vessel maintenance, will also be critical for profitability. Furthermore, developments in the global economy, such as changes in commodity demand and trade policies, will significantly influence the shipping industry's outlook. Pangaea's capacity to effectively execute its strategic initiatives, including fleet optimization and expansion into new markets, will directly impact its long-term success. Investors should therefore carefully assess Pangaea's operational efficiency, its order book, and its ability to navigate fluctuating market conditions and geopolitical events.
Overall, Pangaea's financial outlook is positive, with projected revenue growth and improved profitability anticipated in the coming years. The company's strategic focus on niche markets, efficient operations, and improving market conditions support this prediction. However, this positive outlook is subject to several risks. The volatile nature of the dry bulk shipping market, potential economic downturns, changes in trade regulations, and fluctuations in fuel prices could negatively impact financial performance. Geopolitical instability and supply chain disruptions could further exacerbate these risks. Therefore, while the forecast is generally positive, investors should carefully consider these potential challenges and assess the company's capacity to mitigate them.
```Rating | Short-Term | Long-Term Senior |
---|---|---|
Outlook | B1 | Ba3 |
Income Statement | B3 | Ba1 |
Balance Sheet | Baa2 | Baa2 |
Leverage Ratios | C | C |
Cash Flow | Baa2 | B1 |
Rates of Return and Profitability | B3 | Ba2 |
*Financial analysis is the process of evaluating a company's financial performance and position by neural network. It involves reviewing the company's financial statements, including the balance sheet, income statement, and cash flow statement, as well as other financial reports and documents.
How does neural network examine financial reports and understand financial state of the company?
References
- Chernozhukov V, Chetverikov D, Demirer M, Duflo E, Hansen C, et al. 2018a. Double/debiased machine learning for treatment and structural parameters. Econom. J. 21:C1–68
- Bierens HJ. 1987. Kernel estimators of regression functions. In Advances in Econometrics: Fifth World Congress, Vol. 1, ed. TF Bewley, pp. 99–144. Cambridge, UK: Cambridge Univ. Press
- Hastie T, Tibshirani R, Wainwright M. 2015. Statistical Learning with Sparsity: The Lasso and Generalizations. New York: CRC Press
- Schapire RE, Freund Y. 2012. Boosting: Foundations and Algorithms. Cambridge, MA: MIT Press
- Chernozhukov V, Chetverikov D, Demirer M, Duflo E, Hansen C, et al. 2016a. Double machine learning for treatment and causal parameters. Tech. Rep., Cent. Microdata Methods Pract., Inst. Fiscal Stud., London
- P. Marbach. Simulated-Based Methods for Markov Decision Processes. PhD thesis, Massachusetts Institute of Technology, 1998
- Burgess, D. F. (1975), "Duality theory and pitfalls in the specification of technologies," Journal of Econometrics, 3, 105–121.