AUC Score :
Short-term Tactic1 :
Dominant Strategy :
Time series to forecast n:
ML Model Testing : Modular Neural Network (Market Volatility Analysis)
Hypothesis Testing : Statistical Hypothesis Testing
Surveillance : Major exchange and OTC
1Short-term revised.
2Time series is updated based on short-term trends.
Key Points
A potential rise in the DJ Commodity Nickel Index is anticipated, fueled by increasing demand from the electric vehicle industry and stainless steel production. This upward trajectory could face resistance from supply chain disruptions, geopolitical instability affecting major nickel producing nations, and potential shifts in global economic growth impacting overall industrial demand. The risk associated with this prediction includes volatility due to speculative trading, unforeseen technological advancements leading to alternative materials, and changes in government regulations impacting the mining and refining processes.About DJ Commodity Nickel Index
The Dow Jones Commodity Nickel Index is a financial benchmark designed to reflect the performance of investments in the nickel commodity market. It serves as a key indicator of price movements within the nickel sector, providing investors with a tool to assess market trends and gauge the overall health of the industry. The index tracks the spot price of nickel, typically derived from trading on major commodities exchanges. Its methodology is transparent and aims to offer an accurate representation of nickel's price fluctuations over time, which can be influenced by factors like global demand, production levels, and geopolitical events.
This index is utilized by various market participants, including institutional investors, commodity traders, and financial analysts. They use it for purposes such as performance benchmarking, creating investment products like exchange-traded funds (ETFs), and conducting in-depth market analysis. By following the Dow Jones Commodity Nickel Index, market participants can gain insights into the economic forces affecting nickel's valuation and potentially make informed decisions related to the commodity. This makes the index a valuable resource for anyone seeking to understand the intricacies of the nickel market.
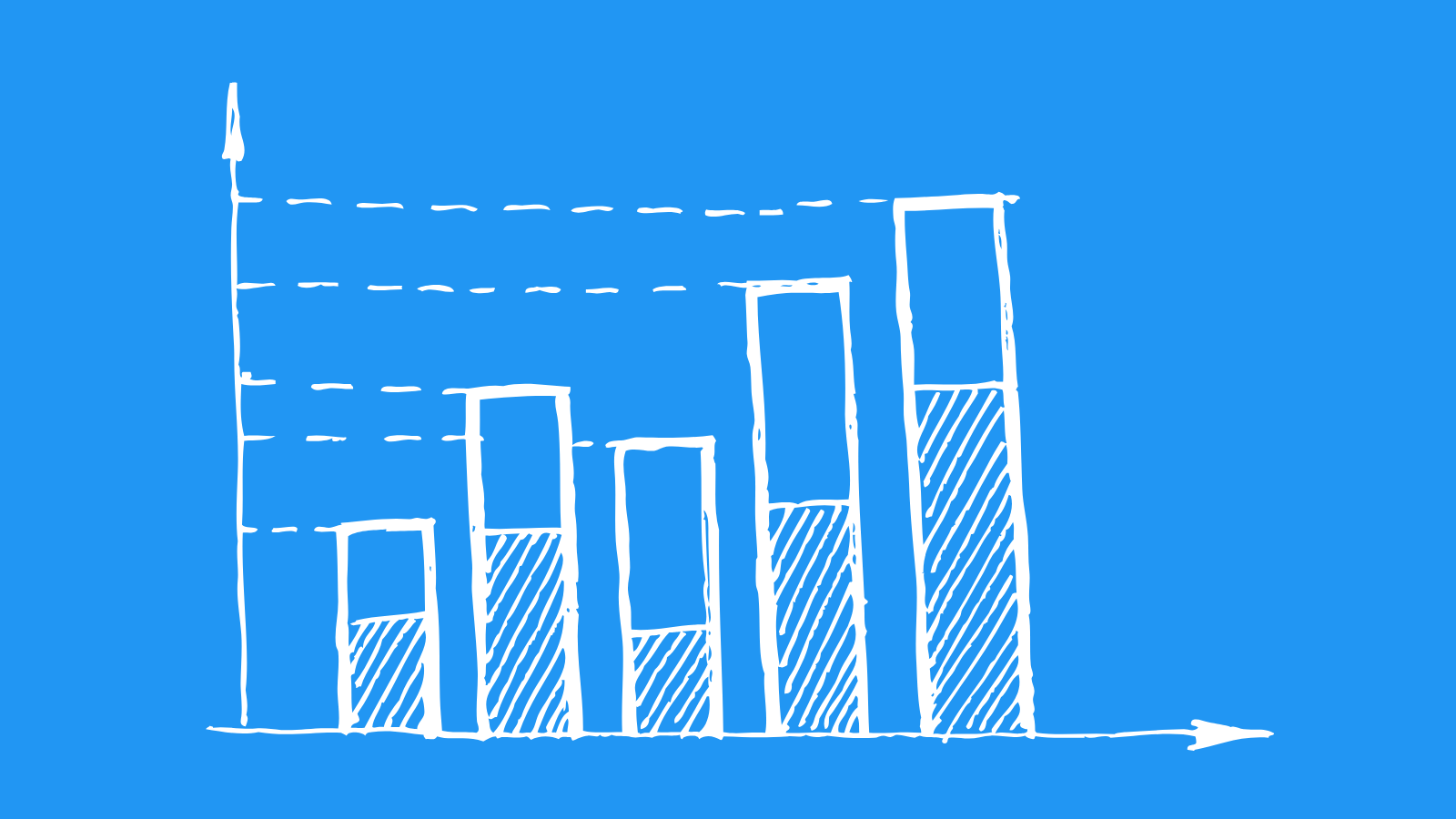
DJ Commodity Nickel Index Forecast Model
Our team of data scientists and economists proposes a comprehensive machine learning model to forecast the DJ Commodity Nickel Index. The core of our approach involves a time-series analysis framework, incorporating a variety of relevant economic and market indicators. We will utilize a blend of advanced techniques, including Recurrent Neural Networks (RNNs), specifically Long Short-Term Memory (LSTM) networks, renowned for their efficacy in capturing complex temporal dependencies in financial data. Furthermore, we will integrate Gradient Boosting Machines (GBMs) for their robustness and predictive power, especially in handling non-linear relationships within the dataset. Key input features will be meticulously selected and engineered. These features encompass past index values, trading volumes, volatility measures (e.g., implied volatility), global economic indicators such as Purchasing Managers' Indices (PMIs) for key manufacturing regions (China, Europe, US), and data related to supply and demand factors, including mine production, inventories, and consumption statistics.
The model's construction emphasizes rigorous feature engineering and selection. We will employ dimensionality reduction techniques, like Principal Component Analysis (PCA), to address multicollinearity and reduce computational burden, while preserving crucial information. The model training process will involve dividing the historical data into training, validation, and testing sets, with careful attention to preserving the temporal ordering of the data to prevent data leakage. Hyperparameter optimization will be conducted using techniques like Grid Search or Bayesian Optimization to fine-tune the models for optimal performance. The model will be evaluated based on standard metrics such as Mean Squared Error (MSE), Root Mean Squared Error (RMSE), and Mean Absolute Error (MAE) to assess forecasting accuracy. Furthermore, the model's robustness will be tested through backtesting, stress testing, and scenario analysis.
The ultimate goal is to provide accurate and reliable forecasts to aid stakeholders in their decision-making processes. The output of our model will be a set of forecasted values for the DJ Commodity Nickel Index, along with confidence intervals to reflect the uncertainty inherent in the predictions. These forecasts can be used to inform investment strategies, risk management decisions, and market analysis. To ensure the ongoing utility of the model, a continuous monitoring and recalibration process is planned. The model will be regularly updated with new data, and its performance will be consistently evaluated. Any degradation in performance will trigger a review of the feature set, hyperparameter tuning, or even an architectural change to the model, ensuring that it remains a valuable and accurate tool for forecasting the DJ Commodity Nickel Index.
ML Model Testing
n:Time series to forecast
p:Price signals of DJ Commodity Nickel index
j:Nash equilibria (Neural Network)
k:Dominated move of DJ Commodity Nickel index holders
a:Best response for DJ Commodity Nickel target price
For further technical information as per how our model work we invite you to visit the article below:
How do KappaSignal algorithms actually work?
DJ Commodity Nickel Index Forecast Strategic Interaction Table
Strategic Interaction Table Legend:
X axis: *Likelihood% (The higher the percentage value, the more likely the event will occur.)
Y axis: *Potential Impact% (The higher the percentage value, the more likely the price will deviate.)
Z axis (Grey to Black): *Technical Analysis%
DJ Commodity Nickel Index: Financial Outlook and Forecast
The DJ Commodity Nickel Index tracks the performance of nickel futures contracts, providing a benchmark for investors seeking exposure to the nickel market. The current outlook for this index is heavily influenced by a confluence of factors, including global demand for nickel, particularly from the electric vehicle (EV) battery sector, supply chain dynamics, and geopolitical events. The rapid expansion of the EV market is the most significant driver, as nickel is a crucial component in lithium-ion batteries. This has spurred significant investment in nickel mining and refining capacities worldwide. Simultaneously, existing supply constraints, exacerbated by disruptions in major producing countries, continue to influence market dynamics. The index's trajectory is thus closely intertwined with the evolving landscape of the EV industry and the logistical realities of nickel extraction and processing. Investors must therefore carefully analyze these factors to determine the outlook for the DJ Commodity Nickel Index.
Analyzing the supply side reveals several crucial aspects. The primary sources of nickel are concentrated in a few countries, exposing the market to geopolitical risks. Any significant disruptions in these areas, such as production shutdowns, export restrictions, or political instability, could substantially impact nickel supply and, consequently, the index's performance. Furthermore, the environmental and social governance (ESG) considerations are gaining prominence. Nickel mining and processing can have significant environmental consequences, and these factors will increasingly influence investment decisions. Mining companies' ability to meet ESG standards will be a critical determinant of their access to financing and their long-term viability, ultimately affecting the index. Lastly, technological innovations in nickel extraction, like the development of new refining technologies and processes, can significantly impact production costs and efficiencies, with the index performance heavily linked to these advancements.
On the demand side, the EV sector will dictate the course for the DJ Commodity Nickel Index. While the demand from the EV industry is very large, it is equally important to look at the consumer demand for EVs. The index performance is tightly linked to consumer sentiments as any changes in consumer behavior could affect nickel demand. The demand from other sectors, like the stainless steel industry, must also be considered. While nickel is essential to producing stainless steel, its demand growth is slower when compared to the EV sector. Understanding these diverse consumption patterns is crucial for any accurate forecast. Furthermore, government policies and incentives, such as subsidies for EV purchases and regulations on emissions, will significantly influence nickel demand. These policies can either accelerate or decelerate the growth of the EV market, thus impacting the index's trajectory.
Considering all these factors, the outlook for the DJ Commodity Nickel Index is potentially positive, but with significant risks. The continued growth of the EV sector and the increasing demand for batteries should support the index's positive performance. However, this forecast is accompanied by substantial risks. Supply chain disruptions, geopolitical instability in nickel-producing countries, and the slow adoption of EVs could lead to price volatility and downward pressure on the index. The successful transition to more sustainable and environmentally friendly extraction and processing methods is vital for the index's long-term outlook. It is equally important to monitor consumer demand, as any decline in consumer sentiment toward EVs could reverse positive price forecasts.
Rating | Short-Term | Long-Term Senior |
---|---|---|
Outlook | B1 | B2 |
Income Statement | Baa2 | Ba2 |
Balance Sheet | Caa2 | C |
Leverage Ratios | B3 | Ba3 |
Cash Flow | Ba2 | B2 |
Rates of Return and Profitability | Ba3 | C |
*An aggregate rating for an index summarizes the overall sentiment towards the companies it includes. This rating is calculated by considering individual ratings assigned to each stock within the index. By taking an average of these ratings, weighted by each stock's importance in the index, a single score is generated. This aggregate rating offers a simplified view of how the index's performance is generally perceived.
How does neural network examine financial reports and understand financial state of the company?
References
- J. Baxter and P. Bartlett. Infinite-horizon policy-gradient estimation. Journal of Artificial Intelligence Re- search, 15:319–350, 2001.
- Clements, M. P. D. F. Hendry (1995), "Forecasting in cointegrated systems," Journal of Applied Econometrics, 10, 127–146.
- Alpaydin E. 2009. Introduction to Machine Learning. Cambridge, MA: MIT Press
- Scott SL. 2010. A modern Bayesian look at the multi-armed bandit. Appl. Stoch. Models Bus. Ind. 26:639–58
- Athey S, Imbens G, Wager S. 2016a. Efficient inference of average treatment effects in high dimensions via approximate residual balancing. arXiv:1604.07125 [math.ST]
- Artis, M. J. W. Zhang (1990), "BVAR forecasts for the G-7," International Journal of Forecasting, 6, 349–362.
- Jacobs B, Donkers B, Fok D. 2014. Product Recommendations Based on Latent Purchase Motivations. Rotterdam, Neth.: ERIM