AUC Score :
Short-term Tactic1 :
Dominant Strategy :
Time series to forecast n:
ML Model Testing : Modular Neural Network (Market Volatility Analysis)
Hypothesis Testing : Independent T-Test
Surveillance : Major exchange and OTC
1Short-term revised.
2Time series is updated based on short-term trends.
Key Points
The DJ Commodity Nickel index is expected to experience moderate volatility. The prediction is for a gradual upward trend, driven by increased demand from the electric vehicle sector, particularly in battery production, while supply constraints could provide support. This trend could be susceptible to shifts in global economic growth, influencing overall industrial demand, and is exposed to potential disruptions in major nickel-producing regions. Geopolitical tensions impacting trade could introduce further fluctuations, and fluctuations in currency exchange rates could influence its movements. A significant economic slowdown or a rapid surge in supply could bring sharp corrections or even a downturn.About DJ Commodity Nickel Index
The Dow Jones Commodity Nickel Index is a financial benchmark that tracks the performance of nickel futures contracts. It's designed to reflect the price movements of this essential industrial metal within the broader commodities market. The index serves as a valuable tool for investors, analysts, and businesses seeking to gauge nickel's price trends and understand its role in the global economy.
The index methodology typically involves weighting nickel futures contracts based on factors such as liquidity and trading volume. This weighting helps ensure that the index accurately represents the market's price discovery process. Tracking this index provides a transparent and accessible way to monitor nickel's price fluctuations. It is often used for purposes such as hedging, investment and risk management, and for gaining insights into the industrial metals sector.
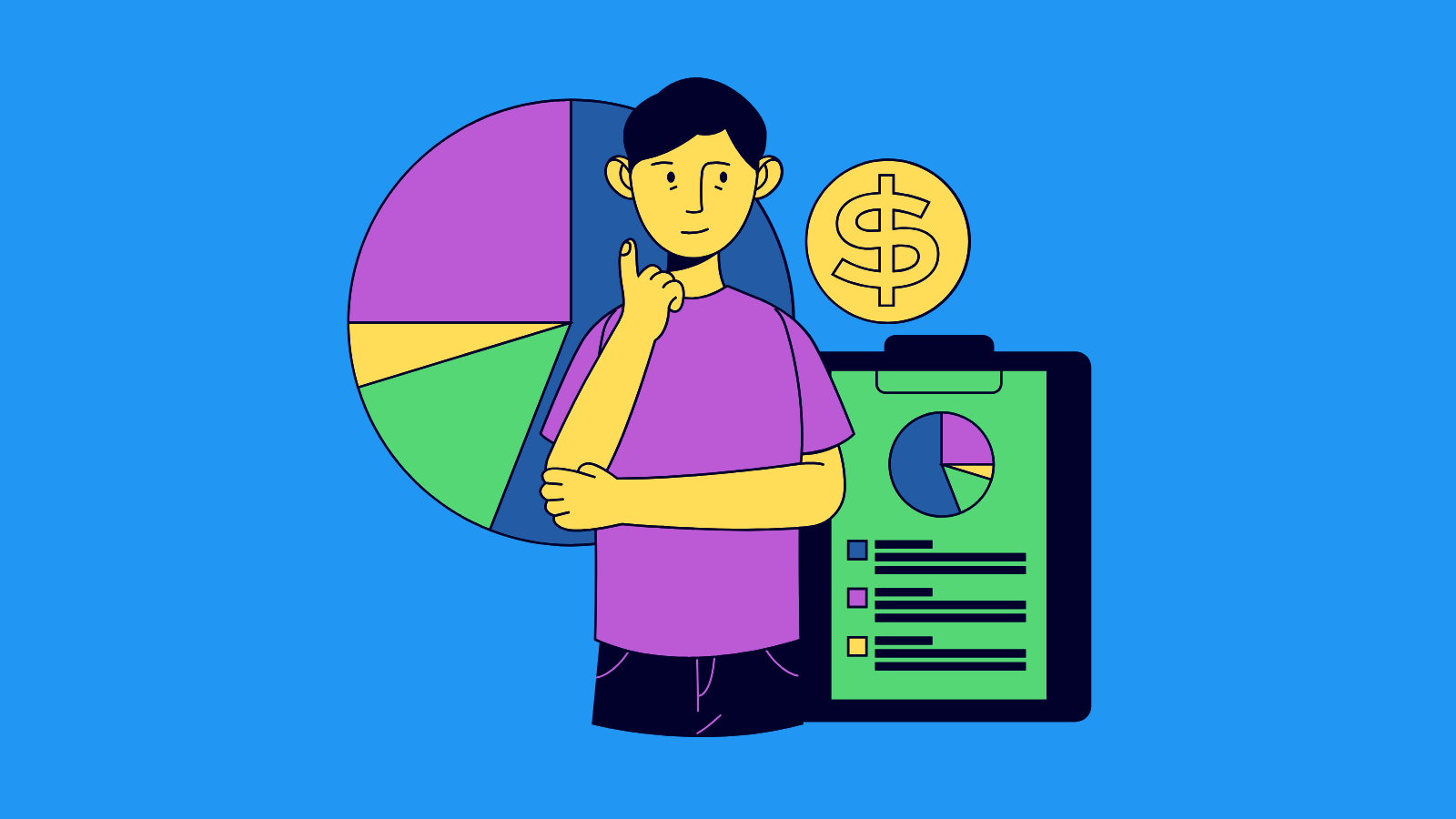
DJ Commodity Nickel Index Forecasting Machine Learning Model
Our team of data scientists and economists has developed a machine learning model to forecast the DJ Commodity Nickel index. The model's architecture incorporates a hybrid approach, combining time series analysis with predictive features derived from economic indicators. We employ a recurrent neural network (RNN), specifically a Long Short-Term Memory (LSTM) network, due to its ability to capture complex temporal dependencies inherent in commodity prices. Crucially, the LSTM network allows the model to retain information over extended periods, adapting to the dynamic nature of the nickel market. This model is trained on a comprehensive dataset spanning several years, including historical DJ Commodity Nickel index values, global economic data (GDP growth, inflation rates), industrial production indices (steel production, battery manufacturing), inventory levels, and currency exchange rates (USD/other major currencies). We use techniques such as data normalization and feature engineering to prepare the data for optimal model performance, and we validated the model's predictive power using rigorous statistical testing, including holdout set and cross-validation
The predictive features incorporated in the model are selected using feature importance techniques. These features encompass leading economic indicators that influence demand and supply for nickel. Economic indicators include PMI (Purchasing Managers' Index) values from major manufacturing economies, as well as interest rate differentials. Supply-side factors are addressed by incorporating production figures from key nickel-producing countries, changes in mining costs, and shifts in the availability of scrap nickel. To enhance robustness, we incorporate sentiment analysis data derived from news articles and social media relating to the nickel market. The sentiment scores influence the output of the model. The model undergoes regular retraining and recalibration to maintain its predictive accuracy as market conditions evolve. The model's output is a probabilistic forecast, providing both a point estimate for the DJ Commodity Nickel index and confidence intervals, allowing our team to assess the uncertainty associated with each prediction.
The model is evaluated using several performance metrics, including Mean Absolute Error (MAE), Root Mean Squared Error (RMSE), and the R-squared statistic. The goal is to ensure a high degree of forecast accuracy and reduce the potential for significant prediction errors. We conduct sensitivity analyses to understand the impact of different features on the model's predictions. Our model provides valuable insights to stakeholders by identifying key factors influencing nickel price movements. The outputs of this model can inform investment strategies, risk management activities, and economic analysis. The model is also designed to be continuously improved through the incorporation of new data, advanced machine learning techniques, and feedback from market observations. The forecasts generated by this model are intended for informational purposes only and should not be taken as financial advice.
ML Model Testing
n:Time series to forecast
p:Price signals of DJ Commodity Nickel index
j:Nash equilibria (Neural Network)
k:Dominated move of DJ Commodity Nickel index holders
a:Best response for DJ Commodity Nickel target price
For further technical information as per how our model work we invite you to visit the article below:
How do KappaSignal algorithms actually work?
DJ Commodity Nickel Index Forecast Strategic Interaction Table
Strategic Interaction Table Legend:
X axis: *Likelihood% (The higher the percentage value, the more likely the event will occur.)
Y axis: *Potential Impact% (The higher the percentage value, the more likely the price will deviate.)
Z axis (Grey to Black): *Technical Analysis%
DJ Commodity Nickel Index: Outlook and Forecast
The DJ Commodity Nickel Index, reflecting the performance of nickel futures contracts, faces a complex outlook shaped by evolving global demand and supply dynamics. On the demand side, the primary driver remains the stainless steel industry, which accounts for the bulk of nickel consumption. Growth in infrastructure spending, particularly in emerging economies, is likely to support continued demand for stainless steel and, consequently, nickel. However, macroeconomic headwinds, including potential slowdowns in major economies like China and the Eurozone, could temper this demand. The increasing adoption of electric vehicles (EVs) presents a secondary but increasingly significant demand source. Nickel is a crucial component in EV batteries, particularly those utilizing nickel-rich chemistries. The accelerated transition to EVs is expected to provide a long-term boost to nickel demand, but the pace of this transition and the specific battery technologies that prevail will significantly influence the extent of this impact. Supply constraints also play a critical role. The nickel market has seen periods of supply deficits and surpluses, which can lead to price volatility. Indonesia's dominance in nickel production, alongside the potential for new mine discoveries and expansions, will likely shape future supply. Environmental concerns and regulatory scrutiny around nickel mining practices could also impact supply availability and costs.
Supply chain dynamics are crucial for this Index. Existing supply-side factors and the pace of new mine development are pivotal for the DJ Commodity Nickel Index. Projects often have long lead times, and any delays in commissioning new mines or disruptions to existing production, whether due to technical issues, labor disputes, or geopolitical instability, can significantly impact the availability of nickel and thus influence the index. Processing and refining infrastructure, particularly the availability of facilities capable of producing battery-grade nickel, will be critical for meeting the growing demand from the EV sector. Changes in trade policies, such as tariffs or export restrictions imposed by major nickel-producing countries, can also introduce uncertainty and volatility. The refining process is a significant factor in the nickel index. The refining process determines the grade and form of nickel produced. Nickel pig iron (NPI) has increased due to higher energy prices and more environmental considerations. Fluctuations in this would lead to significant changes in the prices of the index.
Technological advancements and substitution effects are another relevant consideration. Innovations in battery technology, such as the development of nickel-less batteries, could potentially reduce the demand for nickel in the EV sector, influencing the index negatively. Alternative materials, such as aluminum, may also substitute nickel in specific applications, particularly if nickel prices remain elevated. The environmental, social, and governance (ESG) considerations are becoming increasingly important for the index. Investors and consumers are now more conscious of the environmental impact of nickel mining and processing. Companies are prioritizing sustainable and responsible sourcing practices. The nickel industry is facing increased pressure to reduce its carbon footprint and ensure ethical labor practices. Such initiatives can impact the cost structure of nickel production and thus the price of the metal and the Index. Furthermore, geopolitical tensions, particularly in regions with significant nickel reserves, can have a major impact on the supply chain and prices of the metal.
The outlook for the DJ Commodity Nickel Index appears cautiously optimistic over the medium to long term. The rising EV demand and the expectation of sustained infrastructure spending in emerging markets are likely to support prices. However, near-term volatility should be expected due to macroeconomic uncertainties, potential supply chain disruptions, and fluctuations in the stainless steel industry. The key risks to this prediction include a sharper-than-anticipated economic slowdown in major economies, a more rapid shift to alternative battery technologies, and unforeseen disruptions to nickel production or supply chains. The index's performance will also depend on technological advancements, the adoption of sustainable mining practices, and geopolitical stability. Addressing these risks will be critical for ensuring the long-term success of the nickel industry and the performance of the DJ Commodity Nickel Index.
Rating | Short-Term | Long-Term Senior |
---|---|---|
Outlook | B1 | Ba1 |
Income Statement | C | Baa2 |
Balance Sheet | Baa2 | C |
Leverage Ratios | Caa2 | Ba3 |
Cash Flow | B1 | Baa2 |
Rates of Return and Profitability | Ba3 | Baa2 |
*An aggregate rating for an index summarizes the overall sentiment towards the companies it includes. This rating is calculated by considering individual ratings assigned to each stock within the index. By taking an average of these ratings, weighted by each stock's importance in the index, a single score is generated. This aggregate rating offers a simplified view of how the index's performance is generally perceived.
How does neural network examine financial reports and understand financial state of the company?
References
- Barkan O. 2016. Bayesian neural word embedding. arXiv:1603.06571 [math.ST]
- V. Borkar. Q-learning for risk-sensitive control. Mathematics of Operations Research, 27:294–311, 2002.
- Athey S, Imbens G. 2016. Recursive partitioning for heterogeneous causal effects. PNAS 113:7353–60
- Farrell MH, Liang T, Misra S. 2018. Deep neural networks for estimation and inference: application to causal effects and other semiparametric estimands. arXiv:1809.09953 [econ.EM]
- Mullainathan S, Spiess J. 2017. Machine learning: an applied econometric approach. J. Econ. Perspect. 31:87–106
- Breiman L. 2001b. Statistical modeling: the two cultures (with comments and a rejoinder by the author). Stat. Sci. 16:199–231
- Allen, P. G. (1994), "Economic forecasting in agriculture," International Journal of Forecasting, 10, 81–135.