AUC Score :
Short-term Tactic1 :
Dominant Strategy :
Time series to forecast n:
ML Model Testing : Supervised Machine Learning (ML)
Hypothesis Testing : Independent T-Test
Surveillance : Major exchange and OTC
1Short-term revised.
2Time series is updated based on short-term trends.
Key Points
NICE Ltd ADS faces a future with both promising opportunities and inherent risks. The company is likely to experience growth driven by increasing demand for its cloud-based customer experience solutions, fueled by digital transformation trends across various industries; however, competition from established players and emerging rivals could intensify, potentially eroding market share and profitability. NICE's ability to successfully integrate acquisitions and expand its product offerings to stay relevant will also be crucial. Furthermore, economic downturns and reduced enterprise spending on technology present significant risks, which could negatively impact financial performance. Any significant cybersecurity breaches or data privacy issues could significantly harm the company's reputation.About NICE Ltd
NICE Ltd. is a global provider of cloud and on-premises software solutions, specializing in customer experience (CX) and enterprise applications. The company offers a comprehensive suite of tools designed to optimize customer interactions, improve operational efficiency, and enhance business performance. NICE's offerings cater to various industries, including financial services, healthcare, retail, telecommunications, and the public sector. Their products focus on areas like contact center operations, workforce optimization, analytics, and fraud prevention. The company's focus is on enabling organizations to deliver exceptional customer experiences.
The company operates on a global scale, serving clients in numerous countries with a significant presence in North America, Europe, and the Asia-Pacific region. NICE continually invests in research and development to innovate and provide cutting-edge solutions. The company's strategy centers around helping businesses leverage data, technology, and automation to transform customer service, boost agent productivity, and mitigate risks. NICE strives to be a leading player in the customer experience technology market.
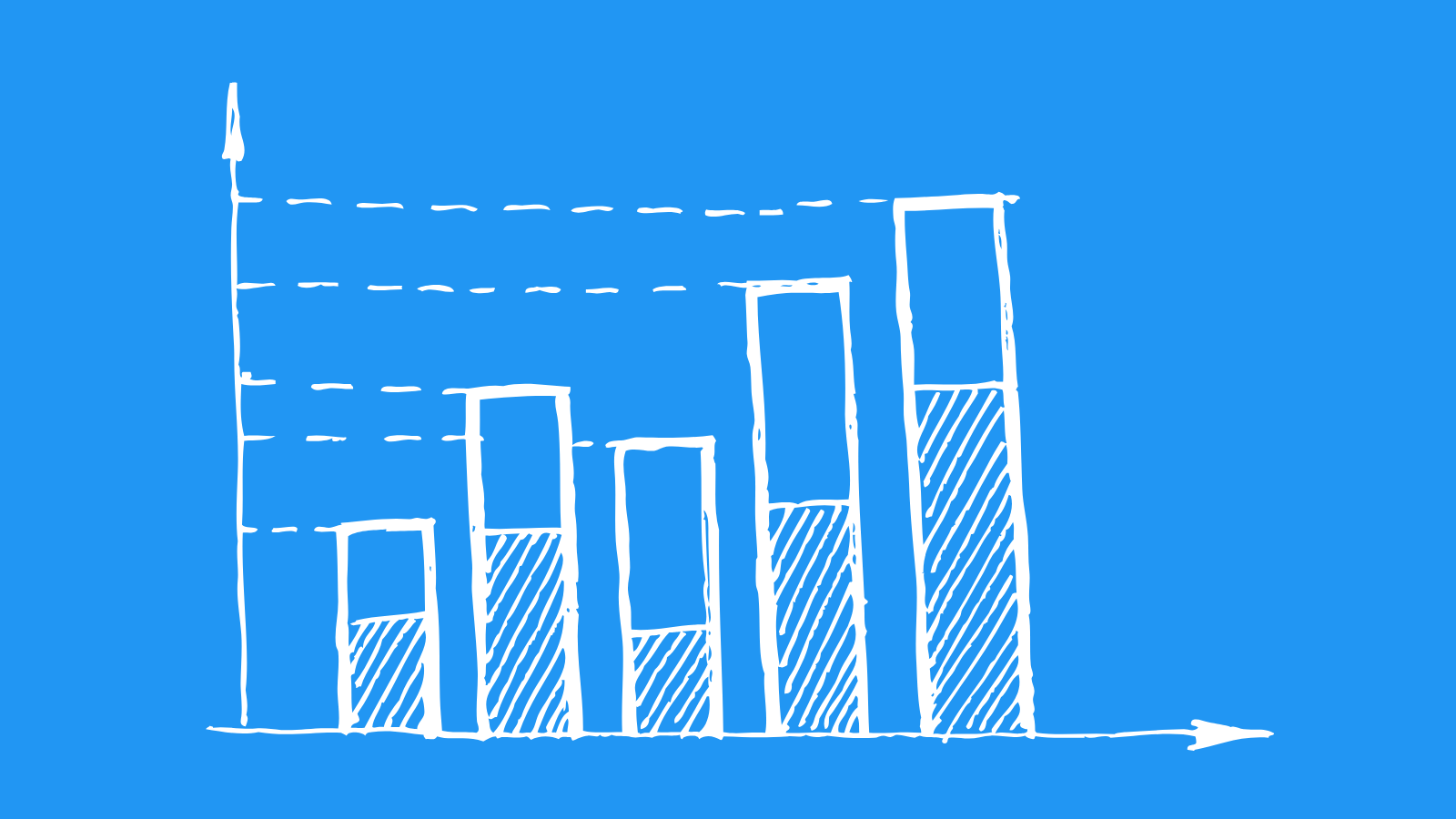
NICE (NICE) Stock Forecast Model
Our data science and economics team proposes a machine learning model for forecasting the performance of NICE Ltd American Depositary Shares (NICE). The core of our model leverages a comprehensive dataset encompassing both internal and external factors. Internal data will include financial statements (revenue, earnings, debt), management guidance, and insider trading activity. External data will incorporate macroeconomic indicators such as GDP growth, inflation rates, and interest rates, as well as industry-specific data concerning cloud computing, customer experience, and competition within the Software-as-a-Service (SaaS) space. Sentiment analysis of news articles, social media, and analyst reports related to NICE will also be integrated to capture market sentiment, which can significantly impact short-term price movements. This multifaceted approach aims to capture the multifaceted drivers of stock behavior.
The model architecture will employ a combination of machine learning techniques. We propose utilizing a Recurrent Neural Network (RNN), specifically a Long Short-Term Memory (LSTM) network, to process time-series data effectively. LSTMs are well-suited for capturing complex temporal dependencies present in financial markets. Furthermore, we will incorporate Gradient Boosting Machines (GBMs), such as XGBoost or LightGBM, to handle non-linear relationships and potential feature interactions. These models can learn from the interplay of diverse predictors. Feature engineering is critical to the model's success; deriving technical indicators from the historical price data and creating relevant ratios from financial data will improve the model's predictive power. The model's performance will be rigorously assessed using metrics like mean absolute error (MAE), root mean squared error (RMSE), and the R-squared value.
To implement the model, we will use Python programming language with the libraries like TensorFlow/Keras, scikit-learn, and pandas. The model will undergo rigorous backtesting with out-of-sample data. To mitigate overfitting and ensure robustness, cross-validation and regularization techniques will be employed during the training phase. We will constantly monitor the model's performance and retrain it periodically with updated data to account for evolving market dynamics. We will also provide insights, not just predictions, incorporating economic context. Regular reports and dashboards will visualize the model's predictions, key drivers, and associated uncertainty bands, which would facilitate well-informed decision-making.
```
ML Model Testing
n:Time series to forecast
p:Price signals of NICE Ltd stock
j:Nash equilibria (Neural Network)
k:Dominated move of NICE Ltd stock holders
a:Best response for NICE Ltd target price
For further technical information as per how our model work we invite you to visit the article below:
How do KappaSignal algorithms actually work?
NICE Ltd Stock Forecast (Buy or Sell) Strategic Interaction Table
Strategic Interaction Table Legend:
X axis: *Likelihood% (The higher the percentage value, the more likely the event will occur.)
Y axis: *Potential Impact% (The higher the percentage value, the more likely the price will deviate.)
Z axis (Grey to Black): *Technical Analysis%
Financial Outlook and Forecast for NICE Ltd. ADS
NICE Ltd., a leading provider of cloud and on-premises enterprise software, faces a complex but generally positive financial outlook. The company's core business revolves around customer experience (CX) and workforce optimization (WFO) solutions, areas experiencing robust growth. The shift towards cloud-based deployments, as NICE accelerates its transition, is expected to generate recurring revenue streams, improving predictability and margins. Investments in artificial intelligence (AI) and analytics capabilities, particularly within its Enlighten AI platform, are key drivers of innovation and competitive advantage. These enhance the company's product offerings and enable the delivery of advanced solutions for customer service, fraud prevention, and compliance. Moreover, NICE's strategy to pursue strategic acquisitions that complement its existing product suite demonstrates a proactive approach to market expansion and technological advancement. The continued expansion of its global footprint also underpins the company's potential for sustained revenue growth, with emerging markets presenting significant opportunities.
The financial forecast for NICE is favorable, supported by trends in the CX and WFO markets. Revenue growth is anticipated, fueled by increasing demand for cloud solutions and the company's innovative offerings. The shift to cloud will contribute to improving profitability margins, allowing NICE to generate strong cash flow. The demand for CX and WFO solutions is expected to remain strong across a variety of industries as businesses compete for and retain customer loyalty. Strong investment in Research and Development, especially within areas of AI, is expected to maintain and improve its market position. Strategic acquisitions can also bolster NICE's revenue profile and expand its target market. Furthermore, continued investments in cybersecurity measures and data privacy are expected to preserve customer confidence and minimize business risk.
Several factors can influence NICE's financial performance. Competition within the CX and WFO software market is intense, with established players and emerging challengers. The success of its product portfolio will also be impacted by the ability of NICE to consistently innovate. Changes in economic conditions and cybersecurity are other external factors that may impact customer spending and IT investments. The geopolitical environment may also pose risks through supply chain disruptions, fluctuations in currency exchange rates, and regional regulatory adjustments. The company's capacity to successfully integrate acquired businesses and achieve operational synergies will be critical for long-term success. Any challenges encountered in transitioning existing customers to cloud-based platforms can lead to service issues and damage the company's customer relationship.
Overall, the financial outlook for NICE is positive, owing to its leading market position, technological innovation, and recurring revenue model. NICE is predicted to experience consistent revenue growth and margin improvement. Risks include competitive pressures, macroeconomic instability, and the need for continuous product development. The company is well positioned to execute its strategic objectives, with positive future returns. However, investors should closely monitor the company's ability to execute its strategies and manage potential risks. The company's strategic investments in AI, cloud technology, and acquisitions are expected to drive sustainable growth, although the competitive landscape requires continuous attention and adaptability. Any delays or setbacks in product implementation or customer adoption can impact the forecast.
```
Rating | Short-Term | Long-Term Senior |
---|---|---|
Outlook | B2 | Ba3 |
Income Statement | B1 | Baa2 |
Balance Sheet | B3 | Baa2 |
Leverage Ratios | C | C |
Cash Flow | B1 | Baa2 |
Rates of Return and Profitability | Caa2 | B1 |
*Financial analysis is the process of evaluating a company's financial performance and position by neural network. It involves reviewing the company's financial statements, including the balance sheet, income statement, and cash flow statement, as well as other financial reports and documents.
How does neural network examine financial reports and understand financial state of the company?
References
- Keane MP. 2013. Panel data discrete choice models of consumer demand. In The Oxford Handbook of Panel Data, ed. BH Baltagi, pp. 54–102. Oxford, UK: Oxford Univ. Press
- Thomas P, Brunskill E. 2016. Data-efficient off-policy policy evaluation for reinforcement learning. In Pro- ceedings of the International Conference on Machine Learning, pp. 2139–48. La Jolla, CA: Int. Mach. Learn. Soc.
- Hirano K, Porter JR. 2009. Asymptotics for statistical treatment rules. Econometrica 77:1683–701
- Belsley, D. A. (1988), "Modelling and forecast reliability," International Journal of Forecasting, 4, 427–447.
- Byron, R. P. O. Ashenfelter (1995), "Predicting the quality of an unborn grange," Economic Record, 71, 40–53.
- K. Tuyls and G. Weiss. Multiagent learning: Basics, challenges, and prospects. AI Magazine, 33(3): 41–52, 2012
- Dudik M, Langford J, Li L. 2011. Doubly robust policy evaluation and learning. In Proceedings of the 28th International Conference on Machine Learning, pp. 1097–104. La Jolla, CA: Int. Mach. Learn. Soc.