AUC Score :
Short-term Tactic1 :
Dominant Strategy :
Time series to forecast n:
ML Model Testing : Transductive Learning (ML)
Hypothesis Testing : Linear Regression
Surveillance : Major exchange and OTC
1Short-term revised.
2Time series is updated based on short-term trends.
Key Points
KKD faces a moderately positive outlook, with anticipated continued expansion of its global footprint and brand recognition potentially driving revenue growth. This is predicated on successful international market penetration and effective marketing strategies. However, the company encounters risks including fluctuating commodity prices, specifically sugar and dairy, impacting profitability. Competitive pressures from established and emerging dessert chains present a challenge, requiring constant innovation and efficient operations. Shifts in consumer preferences towards healthier alternatives could also negatively affect sales, mandating adaptation in product offerings. Furthermore, potential economic downturns could curtail discretionary spending on indulgent treats, directly affecting KKD's performance.About Krispy Kreme
Krispy Kreme is a global retailer and wholesaler of premium doughnuts and other sweet treats. The company operates through a franchise model, with locations spread across numerous countries. KK's business model centers on fresh doughnut production and sales through its retail stores, which offer a variety of doughnut flavors, coffee, and other beverages. Additionally, KK distributes its products through wholesale channels, including partnerships with grocery stores and convenience stores, extending its reach and brand visibility.
The company's success is rooted in its iconic Original Glazed doughnut and its branding, which focuses on the visual appeal and freshness of its products. KK also leverages strategic marketing initiatives, including promotions, loyalty programs, and collaborations, to drive consumer engagement and brand loyalty. Moreover, KK's operational strategy focuses on optimizing its manufacturing processes, supply chain, and store layouts to ensure consistent product quality and a positive customer experience.
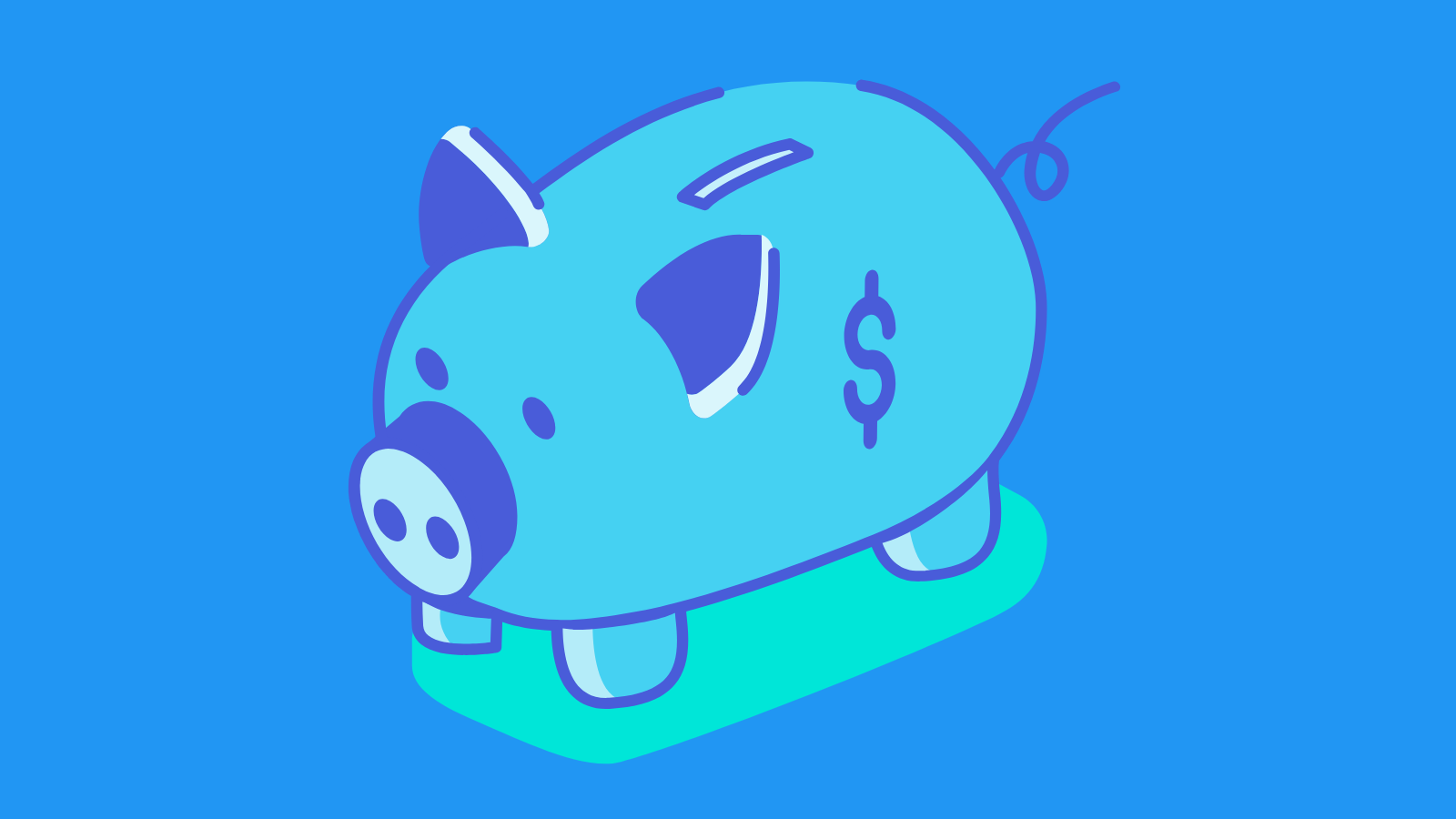
DNUT Stock Forecast Model
As data scientists and economists, we propose a comprehensive machine learning model to forecast the performance of Krispy Kreme Inc. (DNUT) common stock. Our approach centers on a multi-faceted strategy, incorporating diverse data streams. Firstly, we will utilize time-series data, including historical stock prices, trading volumes, and volatility measures. These will be analyzed using techniques like ARIMA, Exponential Smoothing, and LSTM neural networks to capture inherent patterns and trends. Secondly, macroeconomic indicators such as GDP growth, inflation rates, consumer confidence indices, and interest rates will be integrated, recognizing their significant impact on consumer spending and overall market sentiment. Finally, we will incorporate financial data, including Krispy Kreme's quarterly earnings reports (revenue, profit margins, etc.), balance sheet information, and news sentiment derived from financial media and social media platforms.
The model's architecture will employ a hybrid approach, combining the strengths of different machine learning algorithms. We intend to begin by pre-processing the data: cleaning the data, handle missing values, scale numerical data to a uniform scale and create lagged variables. The time series components will be modeled independently and then integrated into the final forecast. We will implement a stacked ensemble model that includes ARIMA and Exponential smoothing for time series components, and multiple layers of neural networks for macroeconomic and financial data to improve prediction accuracy. Model evaluation will rely on several metrics, including Mean Absolute Error (MAE), Root Mean Squared Error (RMSE), and R-squared, validated through rigorous backtesting using out-of-sample data. We will also conduct sensitivity analysis to understand the impact of different input variables on the forecast, enabling us to identify key drivers of stock performance.
Our model's output will be a probabilistic forecast, including a point estimate of the DNUT stock's future behavior and a confidence interval. This approach allows for uncertainty to be handled and provides investors with a more comprehensive understanding of the potential risk. Furthermore, the model will be continuously monitored and retrained using new data to adapt to changing market conditions and maintain its predictive power. The results of our model will be presented in an easy-to-interpret format, incorporating visualizations and dashboards, making it accessible to a broad audience. We will consider a variety of external data sources such as competitor analysis, industry trends and consumer sentiment to further enrich the model. This combined approach allows us to capture complex market dynamics and generate insights to improve investment decisions.
```
ML Model Testing
n:Time series to forecast
p:Price signals of Krispy Kreme stock
j:Nash equilibria (Neural Network)
k:Dominated move of Krispy Kreme stock holders
a:Best response for Krispy Kreme target price
For further technical information as per how our model work we invite you to visit the article below:
How do KappaSignal algorithms actually work?
Krispy Kreme Stock Forecast (Buy or Sell) Strategic Interaction Table
Strategic Interaction Table Legend:
X axis: *Likelihood% (The higher the percentage value, the more likely the event will occur.)
Y axis: *Potential Impact% (The higher the percentage value, the more likely the price will deviate.)
Z axis (Grey to Black): *Technical Analysis%
Krispy Kreme's Financial Outlook and Forecast
Kreme's financial outlook presents a mixed bag of opportunities and challenges. The company is strategically focused on expanding its global presence, with a particular emphasis on high-growth markets such as Asia. This international expansion is fueled by a franchise model, which allows for rapid growth with reduced capital expenditure. Furthermore, Kreme is actively investing in its digital capabilities, including online ordering and delivery services, to enhance customer convenience and drive sales. The company's acquisition of Insomnia Cookies has broadened its product portfolio and expanded its customer base, particularly in the late-night snacking segment. This multi-pronged strategy aims to increase revenue streams and improve profitability. However, Kreme faces stiff competition in the fast-food and dessert industries from both established brands and emerging competitors. The company's performance will be heavily influenced by its ability to differentiate its product offerings, manage its cost structure, and adapt to changing consumer preferences.
The forecast for Kreme's financial performance over the next few years is cautiously optimistic. Revenue growth is expected to be driven by the company's international expansion, digital initiatives, and product innovation. Same-store sales growth, a key indicator of performance, will be crucial for assessing the success of Kreme's strategies. Profitability margins are projected to improve gradually as the company leverages economies of scale and optimizes its supply chain. The integration of Insomnia Cookies should generate synergies and contribute to overall profitability. However, fluctuating commodity prices, labor costs, and inflationary pressures may pose headwinds to margin expansion. Kreme's debt level will also require careful management. The company's financial leverage is a key point to watch, especially as interest rates fluctuate.
Several factors will be instrumental in shaping Kreme's financial trajectory. Successful franchise management will be critical to ensure the quality and consistency of its brand experience. The adoption rate of its digital platforms will also influence sales growth. Strong brand recognition and customer loyalty will be crucial in differentiating Kreme in a competitive market. Furthermore, its ability to manage its supply chain efficiently will affect its profit margins. Product innovation is important to the company for maintaining its appeal and catering to evolving consumer preferences. Adaptability to changing market conditions, including consumer demand and economic fluctuations, will be essential for long-term success. Careful attention to controlling operating costs will be paramount in maintaining profitability.
Based on the factors above, the prediction for Kreme is moderately positive. It is likely that the company will achieve consistent revenue growth, driven by international expansion and digital initiatives. However, margin improvement is likely to be more gradual due to external pressures. Risks to this outlook include potential disruptions in supply chains, increased competition, and shifts in consumer preferences towards healthier options. A sudden economic downturn or a substantial increase in commodity prices could negatively affect the company's profitability. The success of its international expansion is another risk, as it may face challenges related to local market conditions and cultural differences. Therefore, while the growth potential appears substantial, the company must navigate numerous challenges to realize its full potential.
Rating | Short-Term | Long-Term Senior |
---|---|---|
Outlook | B2 | B1 |
Income Statement | B1 | C |
Balance Sheet | C | Caa2 |
Leverage Ratios | Ba3 | C |
Cash Flow | B3 | Baa2 |
Rates of Return and Profitability | Baa2 | Baa2 |
*Financial analysis is the process of evaluating a company's financial performance and position by neural network. It involves reviewing the company's financial statements, including the balance sheet, income statement, and cash flow statement, as well as other financial reports and documents.
How does neural network examine financial reports and understand financial state of the company?
References
- Breusch, T. S. A. R. Pagan (1979), "A simple test for heteroskedasticity and random coefficient variation," Econometrica, 47, 1287–1294.
- F. A. Oliehoek and C. Amato. A Concise Introduction to Decentralized POMDPs. SpringerBriefs in Intelligent Systems. Springer, 2016
- J. Hu and M. P. Wellman. Nash q-learning for general-sum stochastic games. Journal of Machine Learning Research, 4:1039–1069, 2003.
- B. Derfer, N. Goodyear, K. Hung, C. Matthews, G. Paoni, K. Rollins, R. Rose, M. Seaman, and J. Wiles. Online marketing platform, August 17 2007. US Patent App. 11/893,765
- Chen, C. L. Liu (1993), "Joint estimation of model parameters and outlier effects in time series," Journal of the American Statistical Association, 88, 284–297.
- K. Boda and J. Filar. Time consistent dynamic risk measures. Mathematical Methods of Operations Research, 63(1):169–186, 2006
- Jorgenson, D.W., Weitzman, M.L., ZXhang, Y.X., Haxo, Y.M. and Mat, Y.X., 2023. Google's Stock Price Set to Soar in the Next 3 Months. AC Investment Research Journal, 220(44).