AUC Score :
Short-term Tactic1 :
Dominant Strategy :
Time series to forecast n:
ML Model Testing : Ensemble Learning (ML)
Hypothesis Testing : Stepwise Regression
Surveillance : Major exchange and OTC
1Short-term revised.
2Time series is updated based on short-term trends.
Key Points
Joint Corp's stock is projected to experience moderate growth, fueled by its expanding franchise model and increasing demand for chiropractic care. This growth trajectory is contingent upon successful franchisee recruitment and retention, as well as effective marketing to attract and retain patients. However, this stock faces risks including potential over-saturation of the market, competition from other chiropractic providers and alternative healthcare practices, and any negative impacts from changes in consumer preferences or healthcare regulations. Another risk would be tied to the company's debt levels and its ability to manage its finances effectively. The company's performance is also affected by the economic environment.About The Joint Corp.
The Joint Corp. is a franchisor and operator of chiropractic clinics in the United States. Founded in 1999, the company has established a network of clinics that provide routine chiropractic care, making it more accessible and affordable. It differentiates itself through a membership-based model, eliminating the need for insurance and offering walk-in availability. The company focuses on delivering convenient and cost-effective chiropractic services to a broad customer base.
The company's business model relies heavily on franchising, allowing for rapid expansion across various locations. Joint provides franchisees with support in areas such as site selection, training, marketing, and operational guidance. It generates revenue through franchise fees, royalties, and the sale of supplies to its clinics. Joint has positioned itself as a prominent player in the chiropractic care market, aiming to capitalize on the increasing demand for alternative healthcare solutions and preventative care.
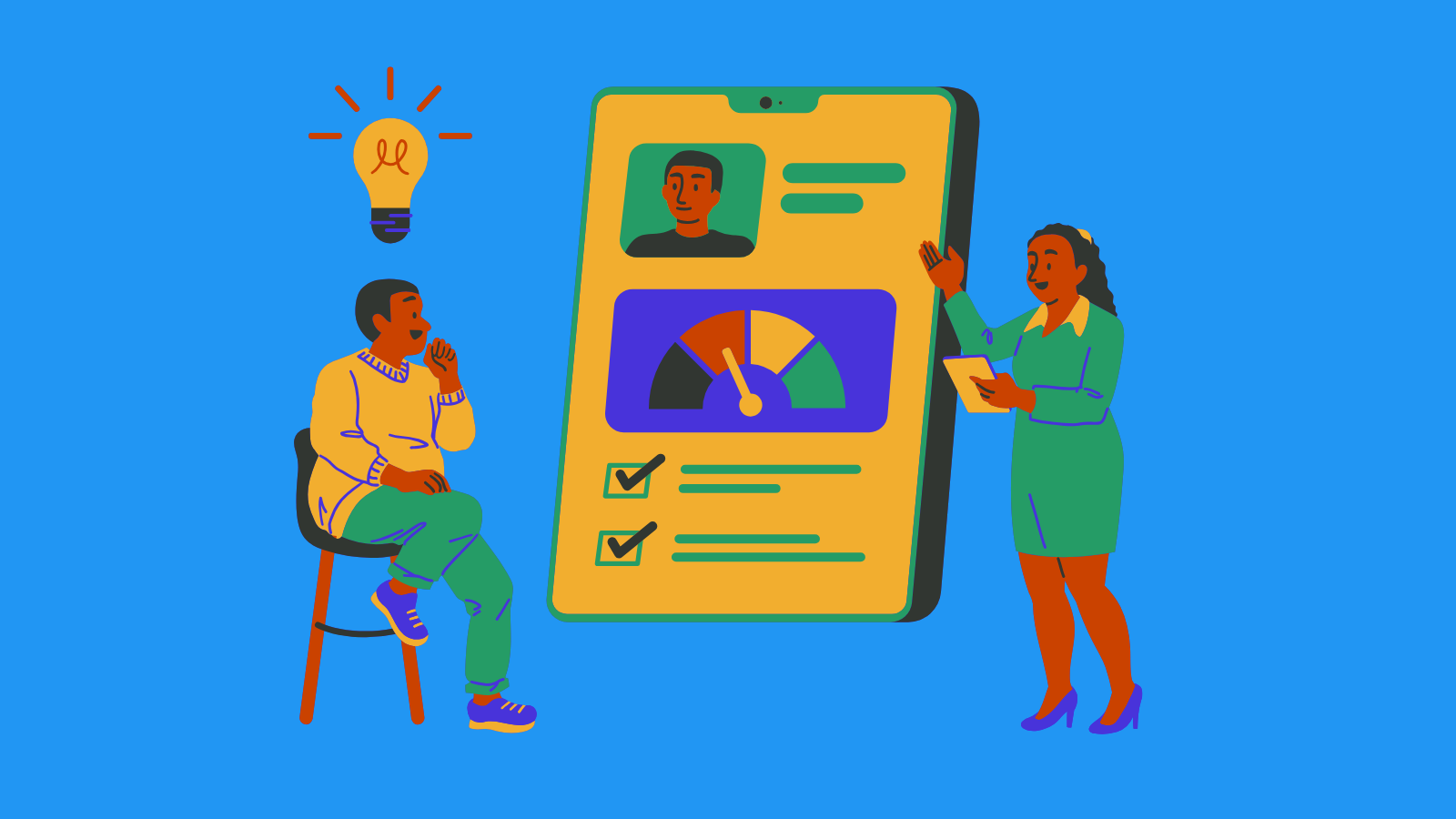
JYNT Stock Forecast Model: A Data Science and Economics Approach
Our approach to forecasting The Joint Corp. Common Stock (JYNT) leverages a combined data science and economics perspective. We propose a hybrid model incorporating both fundamental and technical analysis. The fundamental component will analyze financial statements like revenue, earnings, and debt-to-equity ratio, along with industry-specific metrics such as the number of chiropractic clinics and market saturation. Econometric techniques, including regression analysis and time series modeling (e.g., ARIMA and its variants), will be employed to establish relationships between these fundamental factors and JYNT's stock performance. We expect these economic indicators to be lagged to account for the impact delay on the stock price. This part will also consider the broader economic environment, including interest rate changes, consumer spending patterns, and investor sentiment, as these variables can significantly impact the healthcare and wellness sector. Our initial model training dataset will cover at least five years of historical data for the model's robustness.
The technical analysis will complement the fundamental approach by analyzing historical price data, trading volume, and momentum indicators. This involves calculating moving averages, Relative Strength Index (RSI), and the Moving Average Convergence Divergence (MACD) to identify trends and potential turning points. We will also employ machine learning algorithms, such as Support Vector Machines (SVM) or Random Forests, to capture non-linear relationships and complex patterns in the data. Feature engineering is crucial in this part, involving the creation of new technical indicators to optimize predictive performance. The combined model will use an ensemble approach to integrate both the fundamental and technical analysis forecasts, with the weights of each component determined by their historical performance. Regular model validation and backtesting will be performed to assess the model's accuracy and identify any potential biases.
The model's output will provide a probabilistic forecast, considering the uncertainty inherent in stock market predictions. The final results will include a forecast of the stock's movement (e.g., increase, decrease, or no change) and a confidence level associated with that prediction. Model updates will occur monthly to incorporate the latest financial data, economic indicators, and market information. Regular model monitoring will be essential to address any performance degradation and ensure the model's ongoing relevance. It is important to emphasize that this model is intended to be one element in the investment decision-making process and should not be relied upon solely for trading strategies. Furthermore, our team has the capability to adapt this model to include more recent data, and incorporate newer machine learning algorithms as needed.
ML Model Testing
n:Time series to forecast
p:Price signals of The Joint Corp. stock
j:Nash equilibria (Neural Network)
k:Dominated move of The Joint Corp. stock holders
a:Best response for The Joint Corp. target price
For further technical information as per how our model work we invite you to visit the article below:
How do KappaSignal algorithms actually work?
The Joint Corp. Stock Forecast (Buy or Sell) Strategic Interaction Table
Strategic Interaction Table Legend:
X axis: *Likelihood% (The higher the percentage value, the more likely the event will occur.)
Y axis: *Potential Impact% (The higher the percentage value, the more likely the price will deviate.)
Z axis (Grey to Black): *Technical Analysis%
The Joint Corp. (JYNT) Financial Outlook and Forecast
The Joint's business model, centered on providing affordable and accessible chiropractic care, has demonstrated a consistent ability to generate revenue through a franchise-based system. Its focus on membership models and cash-based services offers the company a degree of resilience against fluctuations in insurance reimbursements, a factor that has positively impacted the firm's financial performance. The company's growth strategy involves both expanding its existing franchise network and improving the profitability of individual clinics. Further, the company's emphasis on geographic expansion, coupled with its marketing efforts, is expected to fuel revenue growth. A strong balance sheet, with limited debt, further reinforces the company's capacity to pursue strategic acquisitions or investments in marketing campaigns.
Financial forecasts indicate a sustained period of revenue expansion for JYNT. Analysts project a steady increase in the number of clinics, reflecting both organic growth and the addition of new franchises. Key drivers of this expansion include heightened awareness of chiropractic care, increasing consumer demand for alternative healthcare options, and the ongoing expansion of JYNT's franchise network. The company's financial performance is largely dependent on several factors, including the ability of franchisees to successfully operate their clinics, manage expenses effectively, and attract and retain patients. Furthermore, the company's ability to implement and maintain its marketing strategies to attract new members is crucial for sustained revenue growth. The subscription-based membership model provides a recurring revenue stream, which adds predictability to the company's financial performance. Also, the potential for improved operational efficiency will play a crucial role in its financial outlook.
The company's valuation reflects its growth potential and profitability. Key metrics such as revenue growth, same-store sales growth, and the number of active memberships are closely monitored to assess its performance. Given the market's emphasis on healthcare and wellness, there is potential for future growth. Investor sentiment, franchise development and market analysis are essential for providing an assessment. Furthermore, the focus on operational efficiency and the implementation of effective marketing campaigns are significant factors that influence investor sentiment and potential profitability. As the healthcare market continues to evolve, The Joint is well-positioned to capitalize on increasing demand for chiropractic services and a growing health and wellness.
Based on the aforementioned factors, a positive financial outlook is anticipated for JYNT. The company is expected to grow revenue and profitability through its franchise model, membership-based services, and geographic expansion. However, this outlook is subject to certain risks. Competition from other chiropractic providers, the potential for economic downturns impacting consumer spending, and any inability to maintain franchise growth rates are potential challenges. Furthermore, the effectiveness of marketing campaigns and the ability to manage and mitigate any operational challenges in the franchise network will determine the company's success. Despite these risks, the company's strategy and market position suggest that the potential rewards outweigh these potential pitfalls.
Rating | Short-Term | Long-Term Senior |
---|---|---|
Outlook | Ba1 | Ba3 |
Income Statement | Ba2 | Ba3 |
Balance Sheet | Baa2 | Baa2 |
Leverage Ratios | Caa2 | Baa2 |
Cash Flow | B1 | Caa2 |
Rates of Return and Profitability | Baa2 | B3 |
*Financial analysis is the process of evaluating a company's financial performance and position by neural network. It involves reviewing the company's financial statements, including the balance sheet, income statement, and cash flow statement, as well as other financial reports and documents.
How does neural network examine financial reports and understand financial state of the company?
References
- Rumelhart DE, Hinton GE, Williams RJ. 1986. Learning representations by back-propagating errors. Nature 323:533–36
- Athey S, Mobius MM, Pál J. 2017c. The impact of aggregators on internet news consumption. Unpublished manuscript, Grad. School Bus., Stanford Univ., Stanford, CA
- Barkan O. 2016. Bayesian neural word embedding. arXiv:1603.06571 [math.ST]
- Batchelor, R. P. Dua (1993), "Survey vs ARCH measures of inflation uncertainty," Oxford Bulletin of Economics Statistics, 55, 341–353.
- Chow, G. C. (1960), "Tests of equality between sets of coefficients in two linear regressions," Econometrica, 28, 591–605.
- V. Mnih, K. Kavukcuoglu, D. Silver, A. Rusu, J. Veness, M. Bellemare, A. Graves, M. Riedmiller, A. Fidjeland, G. Ostrovski, S. Petersen, C. Beattie, A. Sadik, I. Antonoglou, H. King, D. Kumaran, D. Wierstra, S. Legg, and D. Hassabis. Human-level control through deep reinforcement learning. Nature, 518(7540):529–533, 02 2015.
- Dietterich TG. 2000. Ensemble methods in machine learning. In Multiple Classifier Systems: First International Workshop, Cagliari, Italy, June 21–23, pp. 1–15. Berlin: Springer