AUC Score :
Short-term Tactic1 :
Dominant Strategy :
Time series to forecast n:
ML Model Testing : Inductive Learning (ML)
Hypothesis Testing : Linear Regression
Surveillance : Major exchange and OTC
1Short-term revised.
2Time series is updated based on short-term trends.
Key Points
Intchains stock's future is uncertain, with predictions ranging from moderate growth to substantial volatility. The company, operating in a niche sector, faces potential for expansion if it successfully capitalizes on evolving market trends and secures significant partnerships. Conversely, the stock is at risk from intense competition and regulatory scrutiny. Failure to innovate or swiftly adapt to technological shifts could severely impede profitability. The level of investor confidence and broader market conditions also introduce an element of unpredictability. The company's financial performance and ability to scale its operations quickly will significantly determine its trajectory.About Intchains Group: ADS
Intchains Group (ICG) is a company specializing in the development and sale of custom Application-Specific Integrated Circuits (ASICs) and related products. Primarily serving the cryptocurrency mining sector, ICG designs and offers ASICs used in the mining of digital currencies, as well as providing related services. Its product portfolio also includes network equipment and other solutions designed to support blockchain infrastructure. The company's primary focus is on delivering high-performance, energy-efficient computing solutions for blockchain applications.
ICG has operations and research and development centers focused on improving its ASIC designs and developing new products to meet the evolving demands of the blockchain technology market. The company competes within the highly competitive semiconductor industry, where technological advancements and energy efficiency are significant differentiating factors. Its business strategy encompasses technological innovation, and establishing and maintaining strategic partnerships to navigate the dynamic landscape of the digital currency ecosystem.
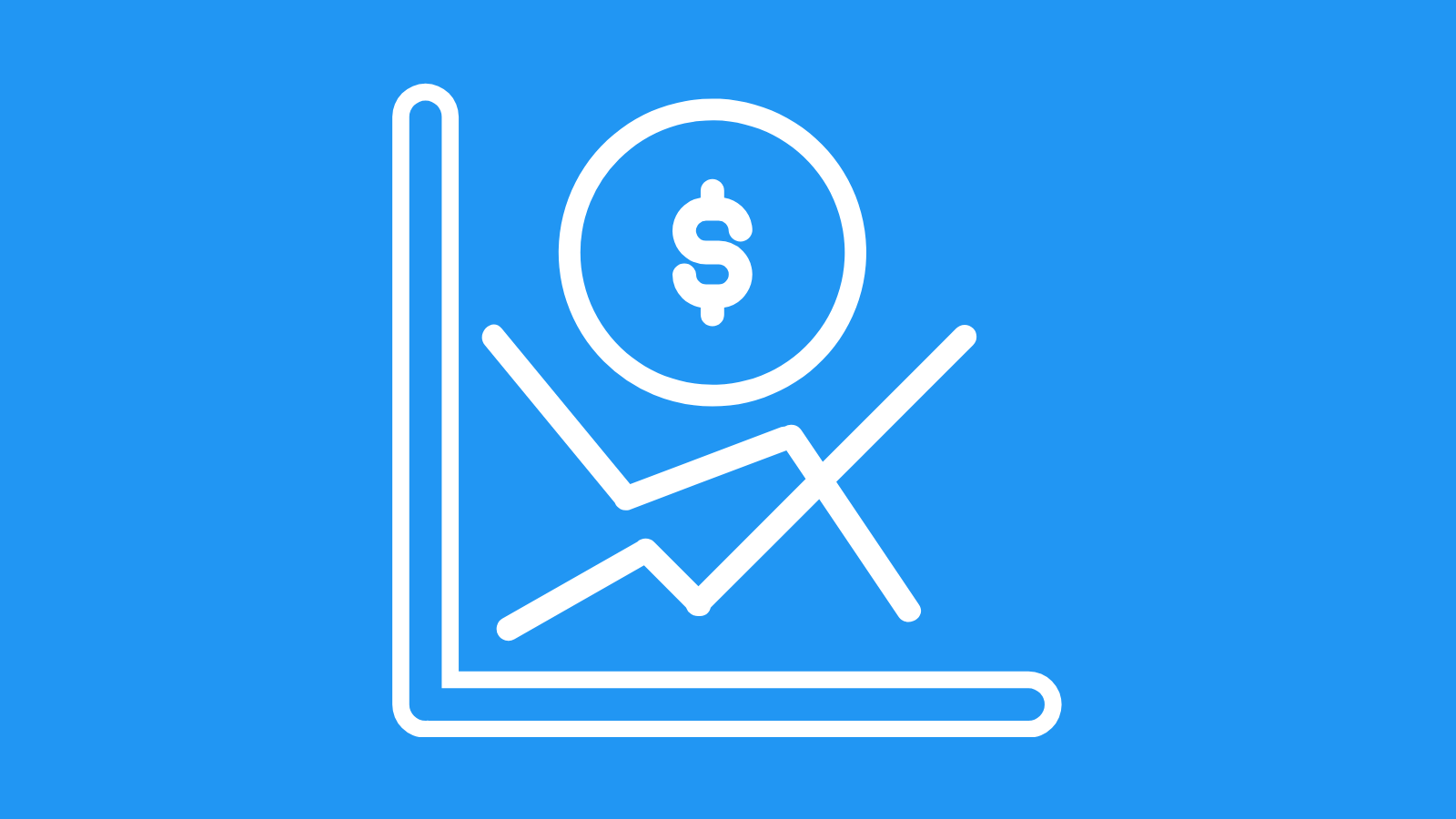
ICG Stock Forecast Model
Our team, comprised of data scientists and economists, has developed a machine learning model to forecast the performance of Intchains Group Limited American Depositary Shares (ICG). The model incorporates a diverse set of features, including historical trading volumes, sentiment analysis of financial news and social media, and macroeconomic indicators such as interest rates and inflation data. We have utilized a combination of regression algorithms, specifically Long Short-Term Memory (LSTM) networks due to their effectiveness in capturing temporal dependencies in time-series data, and Gradient Boosting Machines (GBM) to analyze the importance of each feature to the model. The model is trained on a comprehensive dataset spanning several years, ensuring robustness and adaptability to changing market conditions. Furthermore, we have implemented rigorous cross-validation techniques to optimize hyperparameters and evaluate the model's predictive accuracy.
Feature engineering is crucial to the model's performance. We have conducted thorough exploratory data analysis to identify and create relevant features. This includes calculating technical indicators like moving averages, Relative Strength Index (RSI), and the Moving Average Convergence Divergence (MACD). We also incorporate sentiment scores derived from news articles, earnings reports, and social media activity (e.g., Twitter) related to ICG and the broader technology sector. Moreover, macroeconomic features like the US Dollar Index and the performance of similar companies in the technology space are included to capture external influences. Regular data updates and model retraining are essential to maintain predictive accuracy and adaptability to market evolution.
The forecasting model's output provides a probabilistic prediction of ICG's performance over a specified horizon. The model's outputs will include predicted direction and magnitude of change for ICG's performance over several intervals. The model provides insights for risk assessment, portfolio diversification strategies, and informed investment decisions. The outputs are designed to be easily interpreted, including visualizations, making it useful for investment professionals. It is important to understand that this model, like any predictive tool, is subject to limitations. We are continuously monitoring the model's performance, updating the training data, and refining the feature set to improve its accuracy and maintain its value.
ML Model Testing
n:Time series to forecast
p:Price signals of Intchains Group: ADS stock
j:Nash equilibria (Neural Network)
k:Dominated move of Intchains Group: ADS stock holders
a:Best response for Intchains Group: ADS target price
For further technical information as per how our model work we invite you to visit the article below:
How do KappaSignal algorithms actually work?
Intchains Group: ADS Stock Forecast (Buy or Sell) Strategic Interaction Table
Strategic Interaction Table Legend:
X axis: *Likelihood% (The higher the percentage value, the more likely the event will occur.)
Y axis: *Potential Impact% (The higher the percentage value, the more likely the price will deviate.)
Z axis (Grey to Black): *Technical Analysis%
Financial Outlook and Forecast for Intchains Group Limited (INTC)
Intchains Group Limited, a company specializing in digital and blockchain technology solutions, presents a complex financial outlook. The company's performance is intrinsically linked to the volatile cryptocurrency market and the broader adoption of blockchain technology. The financial forecast hinges on several key factors, including the demand for their hardware and software offerings, the regulatory landscape surrounding digital assets in key markets like China and the United States, and the competitive environment within the blockchain infrastructure space. INTC's revenue streams are likely to fluctuate depending on the global economic conditions, the progression of blockchain adoption, and its ability to secure and maintain key partnerships and client agreements. Recent financial reports have shown that Intchains has been making efforts to broaden its scope from being purely a hardware provider to a company which also engages in software development. The success of that plan, as well as its ability to adapt and innovate within a rapidly evolving technological landscape, will be critical.
The key drivers of Intchains' financial performance are likely to be the growth of the blockchain ecosystem and the demand for its blockchain-related hardware and software. The company could benefit from growing adoption rates of digital assets, as this will likely fuel demand for its products and services. Furthermore, increased institutional interest in blockchain technology could lead to larger projects and collaborations, boosting Intchains' revenue. The strategic development and deployment of the company's own software solutions, rather than solely depending on hardware sales, is an important strategic maneuver that can improve its gross margin. The extent of China's policies on digital assets will also have a significant impact on the company's performance. Intchains must develop its customer base outside of China, because of any limitations in the Chinese markets.
Intchains' financial forecast is subject to significant risks. The cryptocurrency market is known for its high volatility, and sudden downturns could have a negative impact on revenue. Changes in regulatory environments, particularly concerning cryptocurrencies and blockchain technology, could severely disrupt the company's operations. Furthermore, the company operates in a highly competitive landscape with established players and numerous emerging competitors. The ability to innovate, secure market share, and maintain cost efficiency will be critical for survival. Risks tied to potential intellectual property disputes or technological disruptions could also hinder the company's growth trajectory. The company's ability to secure long-term funding and manage its cash flow efficiently will be crucial for its sustained performance.
Based on these factors, the financial outlook for INTC is cautiously optimistic. The prediction is that Intchains will achieve moderate growth in the coming years. This growth will be driven by the continued expansion of the blockchain industry and its own strategic initiatives in software. However, this optimistic outlook is contingent on effective risk management, particularly concerning regulatory changes, volatile market conditions, and competition. The significant risks include a market downturn, negative regulatory developments, and the failure of the company to innovate and adapt. Any major regulatory clampdowns could severely impair operations. The success of any new technology projects will be very important to achieve any growth.
Rating | Short-Term | Long-Term Senior |
---|---|---|
Outlook | B3 | Ba3 |
Income Statement | Caa2 | B2 |
Balance Sheet | C | Ba1 |
Leverage Ratios | Ba3 | Baa2 |
Cash Flow | Caa2 | Ba2 |
Rates of Return and Profitability | B2 | B1 |
*Financial analysis is the process of evaluating a company's financial performance and position by neural network. It involves reviewing the company's financial statements, including the balance sheet, income statement, and cash flow statement, as well as other financial reports and documents.
How does neural network examine financial reports and understand financial state of the company?
References
- C. Claus and C. Boutilier. The dynamics of reinforcement learning in cooperative multiagent systems. In Proceedings of the Fifteenth National Conference on Artificial Intelligence and Tenth Innovative Applications of Artificial Intelligence Conference, AAAI 98, IAAI 98, July 26-30, 1998, Madison, Wisconsin, USA., pages 746–752, 1998.
- Semenova V, Goldman M, Chernozhukov V, Taddy M. 2018. Orthogonal ML for demand estimation: high dimensional causal inference in dynamic panels. arXiv:1712.09988 [stat.ML]
- Chernozhukov V, Newey W, Robins J. 2018c. Double/de-biased machine learning using regularized Riesz representers. arXiv:1802.08667 [stat.ML]
- Candès E, Tao T. 2007. The Dantzig selector: statistical estimation when p is much larger than n. Ann. Stat. 35:2313–51
- E. Altman, K. Avrachenkov, and R. N ́u ̃nez-Queija. Perturbation analysis for denumerable Markov chains with application to queueing models. Advances in Applied Probability, pages 839–853, 2004
- Chen X. 2007. Large sample sieve estimation of semi-nonparametric models. In Handbook of Econometrics, Vol. 6B, ed. JJ Heckman, EE Learner, pp. 5549–632. Amsterdam: Elsevier
- Bottou L. 1998. Online learning and stochastic approximations. In On-Line Learning in Neural Networks, ed. D Saad, pp. 9–42. New York: ACM