AUC Score :
Short-term Tactic1 :
Dominant Strategy :
Time series to forecast n:
ML Model Testing : Transfer Learning (ML)
Hypothesis Testing : Pearson Correlation
Surveillance : Major exchange and OTC
1Short-term revised.
2Time series is updated based on short-term trends.
Key Points
The DJ Commodity Heating Oil index is expected to experience moderate volatility. Supply chain disruptions and geopolitical tensions may cause significant price fluctuations. Increased demand from winter heating could exert upward pressure, but potential economic slowdowns could curb consumption. There is a risk of sudden price spikes due to unforeseen events in key production regions or shifts in global energy policies. Conversely, a milder-than-expected winter coupled with increased production capacity could lead to price corrections, potentially impacting profitability for stakeholders.About DJ Commodity Heating Oil Index
The Dow Jones Commodity Heating Oil Index is a benchmark that tracks the price movements of heating oil futures contracts. It serves as a valuable tool for investors, analysts, and industry participants to monitor the performance of this specific commodity. The index reflects the changes in the spot price of heating oil, providing insights into the market's sentiment towards this crucial energy product. Its composition is based on futures contracts, ensuring that the index is actively traded and offers a degree of price discovery, which can aid in hedging and risk management strategies. The index's construction and methodology are designed to provide a transparent and reliable representation of the heating oil market.
Furthermore, the Dow Jones Commodity Heating Oil Index is often used in conjunction with other commodity indices to gain a broader perspective on the energy sector. By tracking the fluctuations of heating oil, this index contributes to a more comprehensive understanding of global economic trends, particularly those influenced by energy consumption and production. It facilitates analyses of supply and demand dynamics, geopolitical influences, and seasonal variations that impact the pricing of this important heating fuel. The index's data is publicly available, allowing for informed decision-making by diverse stakeholders within the financial markets and the heating oil industry.
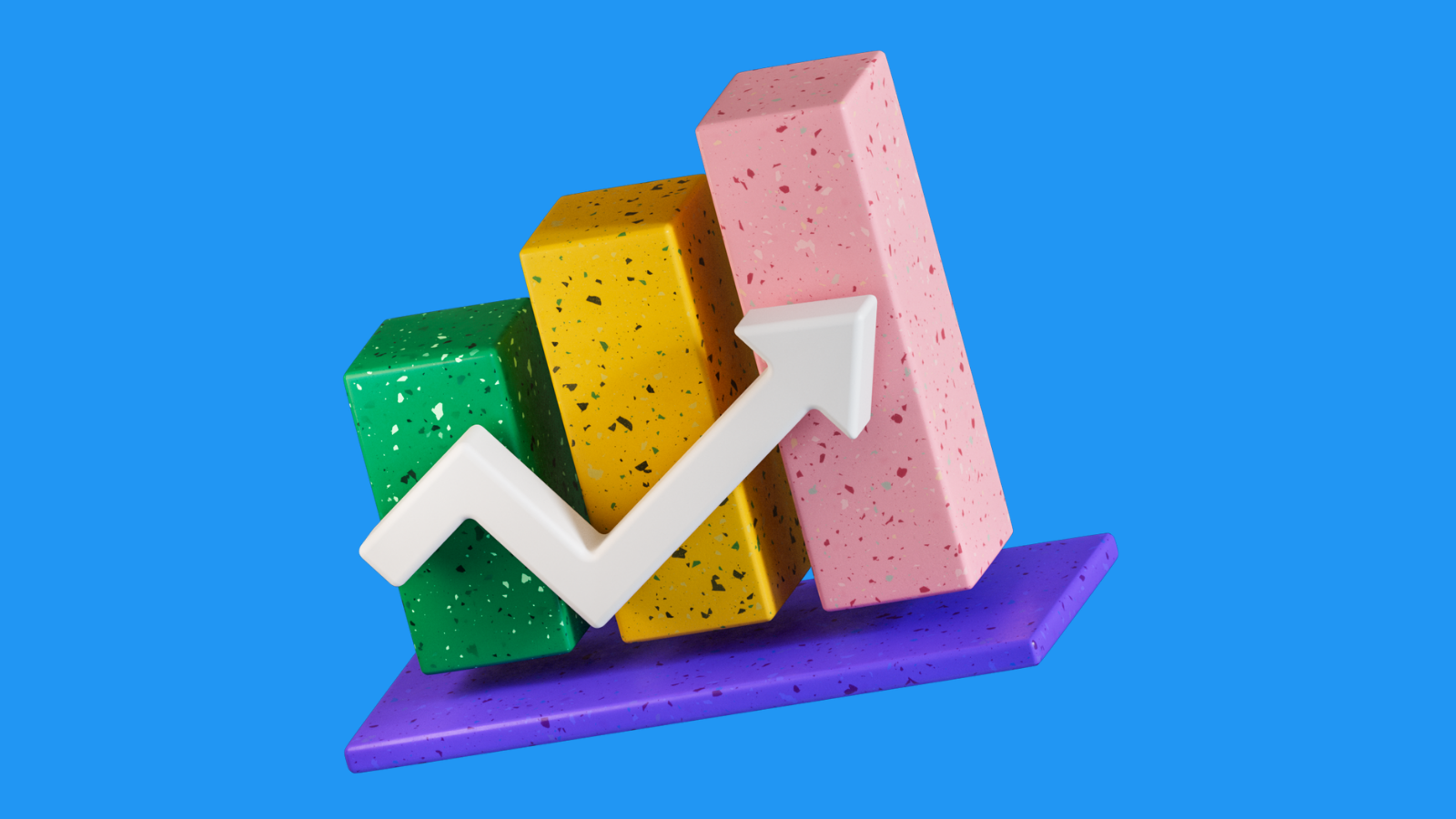
Machine Learning Model for DJ Commodity Heating Oil Index Forecasting
Our team, composed of data scientists and economists, has developed a robust machine learning model to forecast the DJ Commodity Heating Oil index. The model leverages a comprehensive set of features, meticulously selected based on their economic significance and statistical relevance. These features include, but are not limited to, historical heating oil prices, crude oil prices (WTI and Brent), global economic indicators such as GDP growth and industrial production indices, inventory data from the Energy Information Administration (EIA), seasonal factors accounting for heating demand fluctuations, and geopolitical events. We employ a blend of advanced time series analysis techniques and machine learning algorithms to capture both linear and non-linear relationships within the data. The core of our model architecture comprises a combination of Recurrent Neural Networks (RNNs), particularly Long Short-Term Memory (LSTM) networks, to effectively handle sequential data and identify patterns over time, along with ensemble methods like Gradient Boosting for improved predictive accuracy and robustness.
The model training and validation process adheres to rigorous standards. We utilize a significant historical dataset, partitioning it into training, validation, and testing sets. Hyperparameter optimization is conducted using techniques such as grid search and cross-validation to fine-tune the model's performance. We evaluate the model's accuracy using various metrics, including Mean Absolute Error (MAE), Root Mean Squared Error (RMSE), and R-squared, to ensure a comprehensive assessment of its predictive capabilities. Regularization techniques are incorporated to prevent overfitting and enhance generalization ability. Furthermore, we conduct rigorous backtesting on historical data, simulating real-world trading scenarios to evaluate the model's profitability and risk profile, considering transaction costs and market impact. A vital element is the use of feature importance analysis to identify the most influential variables and understand the model's decision-making process, thus enabling greater transparency and explainability.
The final model generates forecasts for the DJ Commodity Heating Oil index at various time horizons. These forecasts are designed to provide actionable insights for market participants, including energy traders, investment firms, and policymakers. The model also incorporates a confidence interval, allowing stakeholders to assess the uncertainty associated with each forecast. Continuous monitoring and model retraining are integral to our approach. The model will be updated periodically, incorporating new data and adjusting to evolving market dynamics. The entire process will involve feedback loops, with economists providing economic context and adjustments, and data scientists refining the machine learning components. This combination of technical expertise and economic insight guarantees an adaptable and efficient forecasting tool.
ML Model Testing
n:Time series to forecast
p:Price signals of DJ Commodity Heating Oil index
j:Nash equilibria (Neural Network)
k:Dominated move of DJ Commodity Heating Oil index holders
a:Best response for DJ Commodity Heating Oil target price
For further technical information as per how our model work we invite you to visit the article below:
How do KappaSignal algorithms actually work?
DJ Commodity Heating Oil Index Forecast Strategic Interaction Table
Strategic Interaction Table Legend:
X axis: *Likelihood% (The higher the percentage value, the more likely the event will occur.)
Y axis: *Potential Impact% (The higher the percentage value, the more likely the price will deviate.)
Z axis (Grey to Black): *Technical Analysis%
DJ Commodity Heating Oil Index: Financial Outlook and Forecast
The outlook for the DJ Commodity Heating Oil Index is intrinsically tied to the complex interplay of global supply and demand dynamics. Recent geopolitical events, including conflicts and sanctions, have demonstrably impacted crude oil production and refining capacity, creating volatility within the energy markets. Furthermore, the approaching winter season in the Northern Hemisphere typically fuels increased demand for heating oil. This seasonal surge, coupled with fluctuating inventory levels and the potential for extreme weather events, exerts considerable influence on price movements. Monitoring the refining capacity utilization rates in key regions, particularly the United States and Europe, becomes essential to assessing potential supply constraints. Examining inventory data from the Energy Information Administration (EIA) is crucial in gauging the market's ability to meet anticipated winter heating demands. Simultaneously, economic indicators, such as industrial production and consumer spending, offer insights into the broader consumption patterns that directly affect heating oil usage.
Key factors that will influence the DJ Commodity Heating Oil Index include OPEC+ decisions on production quotas and the adherence to these agreements by member countries. Any deviations from agreed-upon production levels will significantly influence global supply, and hence, the price of heating oil. Moreover, shifts in the value of the US dollar, the currency in which oil is generally traded, can have a substantial impact. A weaker dollar tends to make oil cheaper for holders of other currencies, potentially boosting demand and driving prices upwards. The advancement of energy transition policies and the adoption of alternative heating solutions such as heat pumps and electric heating will become another factor. Understanding the rate of adoption and its geographic distribution will shed light on how the use of heating oil is changing over time. Changes in global shipping costs, driven by factors such as geopolitical risks, congestion, and fuel costs of ships, will also impact the price of heating oil by affecting the distribution chain.
Looking ahead, the price of the DJ Commodity Heating Oil Index will be influenced by the interplay of these factors. In the short term, the weather patterns of the northern hemisphere become an especially important factor. Cold snaps will most likely lead to higher heating oil prices. Medium-term influences encompass the supply dynamics from the Middle East and Russia, along with the overall economic growth in developed markets. Furthermore, any developments in diplomatic relations or unforeseen events in oil-producing regions have the potential to cause a sharp surge in the index price. Over the longer term, the pace of the energy transition and the regulatory environment surrounding fossil fuels will increasingly shape the index's trajectory. Investments in refining capacity, technological advancements in heating oil production and consumption, and shifts in consumer preferences will play crucial roles. In addition, government policies, such as subsidies for alternative heating systems or carbon taxes on fossil fuels, will exert additional pressure.
A moderate bullish outlook is anticipated for the DJ Commodity Heating Oil Index over the coming year, albeit with considerable volatility. The confluence of seasonal demand, persistent geopolitical risks affecting supply, and potential for economic recovery in some regions create the possibility of upward pressure on prices. The success of OPEC+ in managing production and adherence to agreements will determine the supply side. However, the risks associated with this outlook are substantial. Economic slowdowns in major consuming nations could curtail demand. Unexpected increases in oil production from non-OPEC countries or unforeseen geopolitical developments disrupting supply, like escalation of existing conflicts or natural disasters, could lead to a price collapse. Additionally, the swift adoption of alternative heating technologies, coupled with government policies that discourage fossil fuel usage, could severely impact heating oil demand. Therefore, any investment decisions must be accompanied by ongoing monitoring of market indicators and risk management strategies to navigate the inherent uncertainties in the energy market.
Rating | Short-Term | Long-Term Senior |
---|---|---|
Outlook | B1 | B2 |
Income Statement | Baa2 | Ba1 |
Balance Sheet | Baa2 | B3 |
Leverage Ratios | Ba3 | Caa2 |
Cash Flow | Caa2 | C |
Rates of Return and Profitability | C | B1 |
*An aggregate rating for an index summarizes the overall sentiment towards the companies it includes. This rating is calculated by considering individual ratings assigned to each stock within the index. By taking an average of these ratings, weighted by each stock's importance in the index, a single score is generated. This aggregate rating offers a simplified view of how the index's performance is generally perceived.
How does neural network examine financial reports and understand financial state of the company?
References
- Candès EJ, Recht B. 2009. Exact matrix completion via convex optimization. Found. Comput. Math. 9:717
- Jorgenson, D.W., Weitzman, M.L., ZXhang, Y.X., Haxo, Y.M. and Mat, Y.X., 2023. S&P 500: Is the Bull Market Ready to Run Out of Steam?. AC Investment Research Journal, 220(44).
- O. Bardou, N. Frikha, and G. Pag`es. Computing VaR and CVaR using stochastic approximation and adaptive unconstrained importance sampling. Monte Carlo Methods and Applications, 15(3):173–210, 2009.
- P. Milgrom and I. Segal. Envelope theorems for arbitrary choice sets. Econometrica, 70(2):583–601, 2002
- Bertsimas D, King A, Mazumder R. 2016. Best subset selection via a modern optimization lens. Ann. Stat. 44:813–52
- Jacobs B, Donkers B, Fok D. 2014. Product Recommendations Based on Latent Purchase Motivations. Rotterdam, Neth.: ERIM
- D. Bertsekas. Dynamic programming and optimal control. Athena Scientific, 1995.