AUC Score :
Short-term Tactic1 :
Dominant Strategy :
Time series to forecast n:
ML Model Testing : Supervised Machine Learning (ML)
Hypothesis Testing : Sign Test
Surveillance : Major exchange and OTC
1Short-term revised.
2Time series is updated based on short-term trends.
Key Points
HCA's future appears cautiously optimistic. Predictions suggest continued solid performance driven by aging demographics and ongoing demand for healthcare services, potentially leading to moderate revenue growth and sustained profitability. However, risks include increased labor costs, fluctuations in government reimbursement rates, and potential regulatory changes impacting the healthcare industry. The company is also susceptible to competition from other healthcare providers and the impact of economic downturns which could curtail elective procedures and patient volumes, possibly leading to earnings volatility.About HCA Healthcare
HCA Healthcare, Inc. is a leading provider of healthcare services, operating a vast network of hospitals, freestanding surgery centers, and other healthcare facilities across the United States and the United Kingdom. The company focuses on delivering a comprehensive range of medical services, including acute care, outpatient care, and specialized treatments. HCA Healthcare emphasizes quality patient care, technological advancements, and operational efficiency to maintain a competitive edge in the healthcare industry. The company is dedicated to enhancing the healthcare experience for patients and providing support to medical professionals.
With a significant presence in numerous markets, HCA Healthcare has a broad geographic footprint. Its diverse portfolio of facilities enables it to cater to a wide array of patient needs, from routine medical services to complex surgical procedures. The company is committed to community involvement and invests in programs that promote health and wellness. Furthermore, it prioritizes maintaining a skilled workforce and fostering a culture of continuous improvement to meet the evolving demands of the healthcare sector.
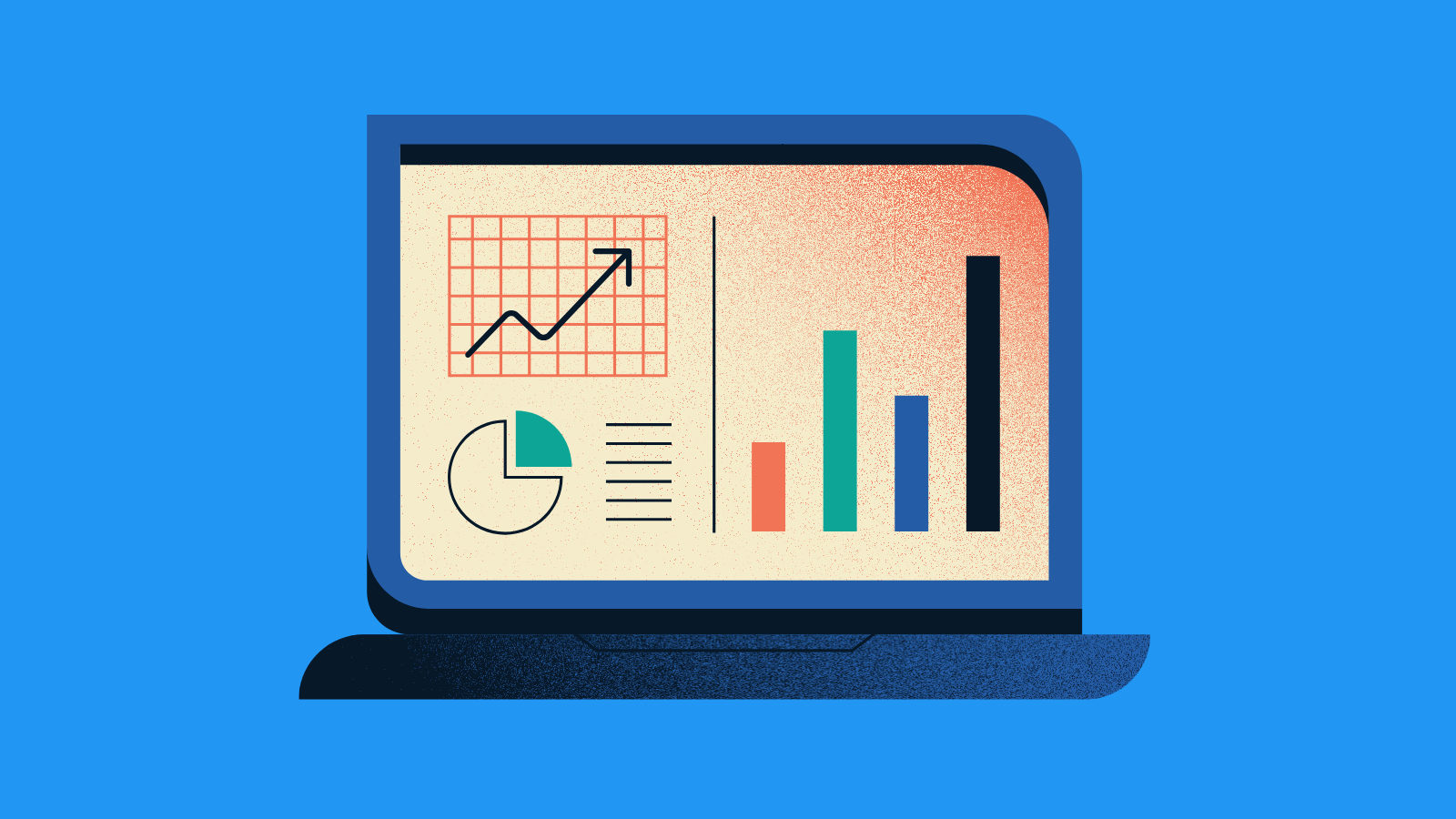
HCA: A Machine Learning Model for Stock Forecast
Our team, comprised of data scientists and economists, has developed a machine learning model to forecast the performance of HCA Healthcare Inc. (HCA) common stock. The model leverages a diverse dataset encompassing both fundamental and technical indicators. We incorporate financial statements data like revenue, earnings per share (EPS), debt-to-equity ratio, and cash flow from operations. This data is crucial for assessing the company's financial health and growth potential. Further, we integrate macroeconomic variables, including interest rates, inflation rates, and healthcare spending trends, which can significantly influence investor sentiment and market dynamics within the healthcare sector. Finally, the model uses technical indicators such as moving averages, Relative Strength Index (RSI), and trading volume, to capture short-term market trends and potential trading signals. We cleaned, preprocessed, and transformed our data, using methods like outlier removal and feature scaling to ensure optimal model performance.
For model development, we experimented with several machine learning algorithms, including Recurrent Neural Networks (RNNs), specifically Long Short-Term Memory (LSTM) networks, and gradient boosting methods, such as XGBoost. RNNs, with their ability to process sequential data, proved particularly effective in capturing the time-series nature of stock prices, allowing the model to learn patterns from historical data. XGBoost, known for its robustness and ability to handle a wide range of features, also demonstrated strong predictive capabilities. The model was trained on historical data and tested on an out-of-sample dataset to evaluate its accuracy and reliability. The model's performance was assessed using metrics like mean squared error (MSE), root mean squared error (RMSE), and the Directional Accuracy, the proportion of correct predictions of the stock's directional movement (up or down).
Our model provides a forecast of the HCA stock's future direction or relative performance, rather than specific absolute price predictions. The model output can be used to gauge the potential for both short-term and long-term investment strategies. The output, which includes confidence intervals, allows users to assess the risk associated with the predictions. Although we aim for accuracy, it is crucial to understand that stock market forecasting remains inherently challenging and influenced by numerous external factors. Therefore, the model should be considered as a tool to aid in informed decision-making, not as a guaranteed profit generator. Ongoing model maintenance, including periodic retraining and feature updates, is necessary to maintain performance as market conditions evolve.
ML Model Testing
n:Time series to forecast
p:Price signals of HCA Healthcare stock
j:Nash equilibria (Neural Network)
k:Dominated move of HCA Healthcare stock holders
a:Best response for HCA Healthcare target price
For further technical information as per how our model work we invite you to visit the article below:
How do KappaSignal algorithms actually work?
HCA Healthcare Stock Forecast (Buy or Sell) Strategic Interaction Table
Strategic Interaction Table Legend:
X axis: *Likelihood% (The higher the percentage value, the more likely the event will occur.)
Y axis: *Potential Impact% (The higher the percentage value, the more likely the price will deviate.)
Z axis (Grey to Black): *Technical Analysis%
HCA Healthcare Inc. Financial Outlook and Forecast
HCA Healthcare (HCA) demonstrates a robust financial outlook, underpinned by its significant market presence and operational efficiency within the healthcare industry. The company's revenue stream is diversified across a network of hospitals, outpatient facilities, and clinics, offering a comprehensive suite of services. Recent trends highlight HCA's ability to maintain a healthy revenue growth rate, supported by strong patient volumes and strategic acquisitions. The company's geographic diversity, with operations across the United States and the United Kingdom, mitigates regional economic fluctuations and enhances overall stability. Management's focus on cost management, including supply chain optimization and workforce productivity, has been critical in improving profitability. HCA's strategic investments in technology and infrastructure, especially in areas such as telehealth and data analytics, further bolster its competitive positioning and patient care capabilities.
The forecast for HCA's financial performance remains positive, considering several key factors. The aging population and increasing prevalence of chronic diseases are expected to drive demand for healthcare services, benefiting companies such as HCA. The company's strong reputation and brand recognition among both patients and physicians will aid in maintaining patient volumes. Furthermore, HCA's consistent track record of integrating acquired facilities and improving operational efficiency indicates the potential for further margin expansion. Positive trends in outpatient services and specialized care are also likely to continue, providing additional revenue streams. Investments in value-based care models, which focus on patient outcomes, will also allow HCA to align its interests with the evolving healthcare ecosystem.
Analyzing HCA's financial forecasts involves a combination of qualitative and quantitative measures. Analysts generally expect a continuation of solid revenue growth, coupled with a steady increase in earnings per share. The company's ability to manage its substantial debt load and capital expenditures will be essential for future profitability. Key performance indicators to observe include same-facility admissions, adjusted EBITDA margins, and cash flow generation. HCA's ability to adapt to ongoing changes in the healthcare landscape, especially with regard to reimbursement models and evolving regulatory requirements, will determine its long-term success. The company's financial strength, demonstrated by its capacity to generate healthy cash flow and return capital to shareholders through share repurchases, supports a positive outlook.
Overall, the outlook for HCA is deemed positive, predicated on a sustained demand for healthcare services and the company's established operational strengths. The major risk to this outlook is an unforeseen regulatory environment that negatively impacts reimbursement rates or healthcare access. Furthermore, economic downturns that may diminish patient volume and/or put financial pressure on healthcare expenses would need to be considered. Nevertheless, the company's ability to navigate market changes, invest strategically, and maintain operational efficiency positions it favorably for continued success in the healthcare sector. Therefore, a positive outlook is predicted with the potential for continued growth, assuming external factors do not drastically shift the fundamental pillars of its operations.
Rating | Short-Term | Long-Term Senior |
---|---|---|
Outlook | B2 | Ba3 |
Income Statement | Caa2 | Baa2 |
Balance Sheet | B1 | C |
Leverage Ratios | Ba3 | Ba2 |
Cash Flow | B1 | B2 |
Rates of Return and Profitability | Caa2 | Ba1 |
*Financial analysis is the process of evaluating a company's financial performance and position by neural network. It involves reviewing the company's financial statements, including the balance sheet, income statement, and cash flow statement, as well as other financial reports and documents.
How does neural network examine financial reports and understand financial state of the company?
References
- Bessler, D. A. S. W. Fuller (1993), "Cointegration between U.S. wheat markets," Journal of Regional Science, 33, 481–501.
- Mnih A, Kavukcuoglu K. 2013. Learning word embeddings efficiently with noise-contrastive estimation. In Advances in Neural Information Processing Systems, Vol. 26, ed. Z Ghahramani, M Welling, C Cortes, ND Lawrence, KQ Weinberger, pp. 2265–73. San Diego, CA: Neural Inf. Process. Syst. Found.
- Abadir, K. M., K. Hadri E. Tzavalis (1999), "The influence of VAR dimensions on estimator biases," Econometrica, 67, 163–181.
- Athey S, Bayati M, Doudchenko N, Imbens G, Khosravi K. 2017a. Matrix completion methods for causal panel data models. arXiv:1710.10251 [math.ST]
- S. Proper and K. Tumer. Modeling difference rewards for multiagent learning (extended abstract). In Proceedings of the Eleventh International Joint Conference on Autonomous Agents and Multiagent Systems, Valencia, Spain, June 2012
- K. Tumer and D. Wolpert. A survey of collectives. In K. Tumer and D. Wolpert, editors, Collectives and the Design of Complex Systems, pages 1–42. Springer, 2004.
- V. Borkar and R. Jain. Risk-constrained Markov decision processes. IEEE Transaction on Automatic Control, 2014