AUC Score :
Short-term Tactic1 :
Dominant Strategy :
Time series to forecast n:
ML Model Testing : Transfer Learning (ML)
Hypothesis Testing : Stepwise Regression
Surveillance : Major exchange and OTC
1Short-term revised.
2Time series is updated based on short-term trends.
Key Points
Haverty's future appears cautiously optimistic, projecting moderate growth driven by increased housing starts and consumer spending on home furnishings. This could lead to a steady rise in revenue and potentially improved profitability margins, especially if the company successfully navigates supply chain challenges and maintains its strong brand reputation. However, risks include economic downturns impacting consumer discretionary spending, increased competition from online retailers and other furniture chains, and potential fluctuations in raw material costs. Furthermore, changes in consumer preferences and evolving industry trends also pose challenges that the company must adapt to effectively in order to sustain positive results.About Haverty Furniture
Haverty Furniture Companies, Inc. (HVT) is a prominent retailer specializing in the sale of home furnishings and accessories. Founded in 1885, the company has a long-standing presence in the furniture retail industry, operating a chain of stores primarily located in the Southeastern, South Central, and Midwestern United States. Haverty's offers a wide selection of products, including furniture for living rooms, bedrooms, dining rooms, and home offices, alongside a variety of decorative items, mattresses, and home accents.
The company's business model centers on providing a comprehensive shopping experience, emphasizing customer service and financing options. Haverty's typically operates from large-format retail locations and has a well-established brand reputation built on quality merchandise. As a publicly traded company, it focuses on maintaining its market position through strategic store expansions, merchandise assortment, and adapting to evolving consumer preferences in the home furnishings sector.
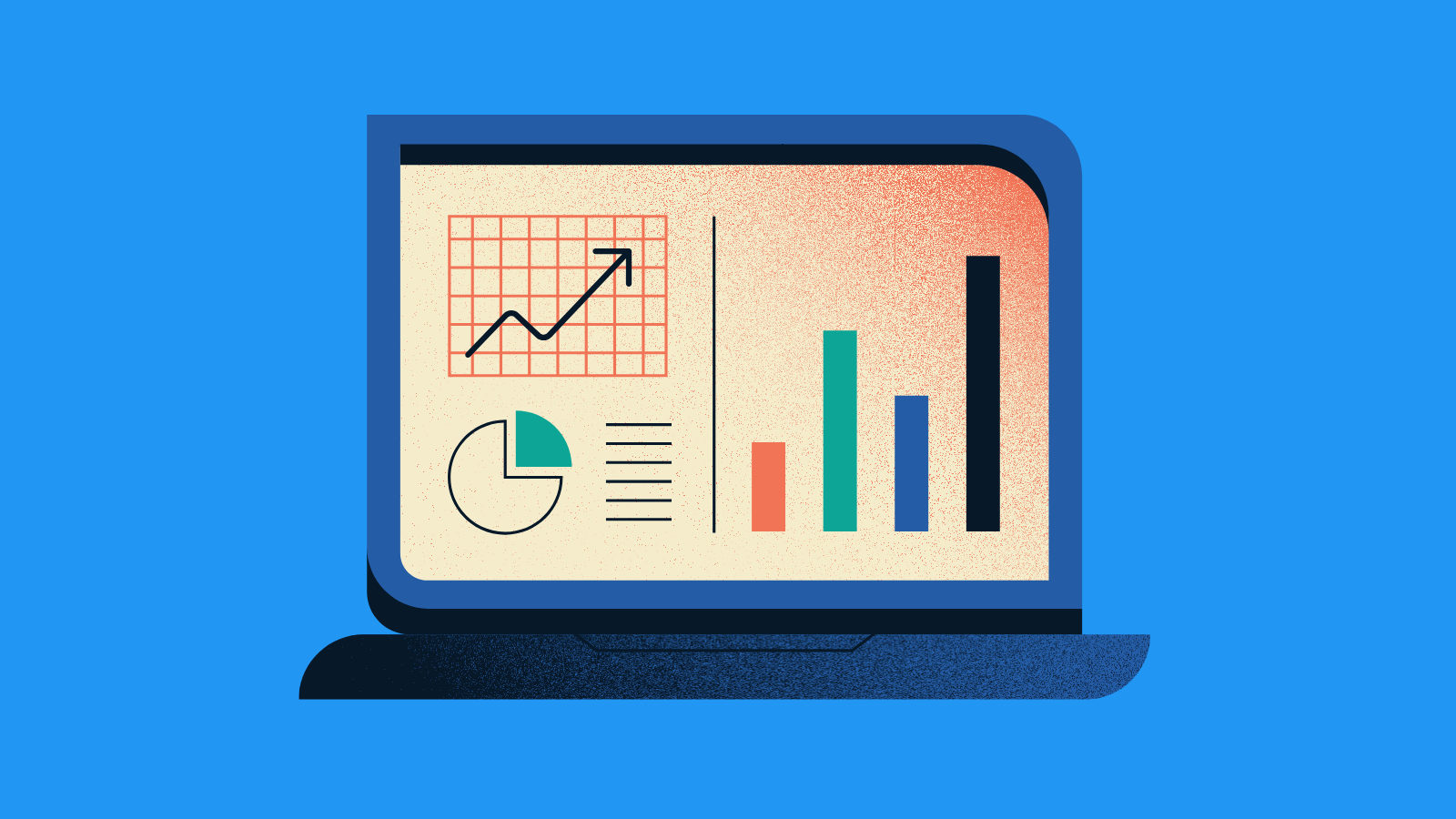
HVT Stock Forecast Model
The development of a predictive model for Haverty Furniture Companies Inc. (HVT) stock performance necessitates a multifaceted approach, integrating both fundamental and technical analysis. Our model will leverage a comprehensive dataset encompassing historical stock prices, trading volume, and market sentiment indicators. Crucially, we will incorporate financial metrics such as revenue, earnings per share (EPS), debt-to-equity ratio, and dividend yield. These fundamental indicators offer insights into the company's financial health and growth potential, while technical indicators such as moving averages, Relative Strength Index (RSI), and trading patterns will be utilized to identify trends and potential buy/sell signals. The model will be trained using a combination of machine learning algorithms, including Long Short-Term Memory (LSTM) networks for time series forecasting and support vector machines (SVM) for classification, to generate accurate and reliable predictions.
The modeling process begins with data collection, cleaning, and feature engineering. We will gather data from reputable sources, including financial news outlets, SEC filings, and financial data providers. The collected data will undergo rigorous cleaning to address missing values and outliers. Feature engineering will involve creating new variables from existing ones, such as calculating growth rates, profitability ratios, and momentum indicators. The model will be trained on a portion of the data (the training set) and validated on a separate portion (the validation set) to assess its performance. Evaluation metrics like Mean Absolute Error (MAE), Root Mean Squared Error (RMSE), and classification accuracy will be employed to compare the performance of different algorithms and optimize model parameters. To mitigate the risks associated with market volatility, we will continuously monitor the model's performance and retrain it periodically with updated data, thus enabling dynamic adjustment of the prediction outputs.
The output of the model will provide a forecast of HVT stock performance. For instance, the model may provide a predicted price trend over a specified timeframe (e.g., next quarter), as well as generate buy, sell, or hold recommendations. The interpretation of the output must consider the inherent uncertainties of stock market forecasting, which are greatly impacted by unpredictable external factors and market conditions. Although the machine learning model seeks to provide highly accurate predictions, our team of data scientists and economists will monitor the model to ensure it meets our specific requirements and we will consider qualitative factors, such as industry trends, economic outlook, and management's strategic initiatives, which will be used to interpret the model's outputs. This approach will help with the generation of a final investment recommendation.
ML Model Testing
n:Time series to forecast
p:Price signals of Haverty Furniture stock
j:Nash equilibria (Neural Network)
k:Dominated move of Haverty Furniture stock holders
a:Best response for Haverty Furniture target price
For further technical information as per how our model work we invite you to visit the article below:
How do KappaSignal algorithms actually work?
Haverty Furniture Stock Forecast (Buy or Sell) Strategic Interaction Table
Strategic Interaction Table Legend:
X axis: *Likelihood% (The higher the percentage value, the more likely the event will occur.)
Y axis: *Potential Impact% (The higher the percentage value, the more likely the price will deviate.)
Z axis (Grey to Black): *Technical Analysis%
Haverty Furniture Companies Inc. (HVT) Financial Outlook and Forecast
The financial outlook for HVT appears cautiously optimistic, driven by several key factors shaping the home furnishings market. The company is strategically positioned to benefit from the continued demand for home improvement and furnishing, a trend partially fueled by evolving consumer preferences towards comfortable and aesthetically pleasing living spaces. HVT's established brand recognition, extensive retail presence across a broad geographic footprint, and emphasis on customer service represent significant competitive advantages. The company's focus on providing a curated selection of quality furniture and home decor aligns well with consumer willingness to invest in their homes. Further, HVT has demonstrated a capacity for adapting its business model to navigate changing economic conditions, including the ability to manage its supply chain effectively and adapt to fluctuating consumer demand. The company's historical performance suggests its ability to withstand economic downturns, a critical strength in the volatile retail environment. Strong management is an important factor for the company.
Projected financial performance is anticipated to show moderate growth in revenue, reflecting ongoing consumer spending on home furnishings and HVT's strategic initiatives. The company's focus on omnichannel retail, leveraging both its physical stores and online platforms, is expected to facilitate continued sales growth. Investments in technology, including supply chain optimization and data analytics, should contribute to operational efficiencies, ultimately improving profitability. Furthermore, HVT's capacity to manage inventory levels and control expenses will be crucial in preserving its profit margins. Strategic partnerships and collaborations could further enhance its market reach and brand visibility. The home furnishings market is cyclical, and economic indicators will play a major role. HVT's performance will largely depend on the economy.
Looking ahead, analysts are projecting a stable outlook for HVT's profitability, with potential for modest improvements. Revenue growth is likely to continue, supported by the company's ongoing efforts to expand its product offerings and enhance the customer experience. Cost-control measures, coupled with supply chain efficiencies, are expected to help mitigate pressure on profit margins. While external factors like inflation and fluctuations in consumer sentiment pose potential headwinds, HVT's track record of adapting to market changes suggests resilience. Investing in digital infrastructure is very important for future growth, and it can help the company reach a wider range of customers.
Overall, the financial forecast for HVT is positive, assuming the company can manage economic challenges. We predict the company will likely see stable growth with increased revenue. However, there are risks to this prediction. Economic downturn, rising interest rates, and changes in consumer spending patterns pose potential challenges to the company's growth trajectory. Furthermore, increased competition from online retailers and other furniture brands could erode HVT's market share. Any unforeseen supply chain disruptions, geopolitical events, or shifts in consumer spending patterns could negatively affect financial performance. Careful monitoring of market conditions and proactive adaptation strategies will be vital for HVT to realize its growth potential and maintain its competitive position within the home furnishings market.
Rating | Short-Term | Long-Term Senior |
---|---|---|
Outlook | B1 | B1 |
Income Statement | Baa2 | Caa2 |
Balance Sheet | B2 | C |
Leverage Ratios | Ba1 | Ba2 |
Cash Flow | Ba3 | B2 |
Rates of Return and Profitability | C | Baa2 |
*Financial analysis is the process of evaluating a company's financial performance and position by neural network. It involves reviewing the company's financial statements, including the balance sheet, income statement, and cash flow statement, as well as other financial reports and documents.
How does neural network examine financial reports and understand financial state of the company?
References
- Imbens G, Wooldridge J. 2009. Recent developments in the econometrics of program evaluation. J. Econ. Lit. 47:5–86
- Byron, R. P. O. Ashenfelter (1995), "Predicting the quality of an unborn grange," Economic Record, 71, 40–53.
- R. Howard and J. Matheson. Risk sensitive Markov decision processes. Management Science, 18(7):356– 369, 1972
- K. Boda and J. Filar. Time consistent dynamic risk measures. Mathematical Methods of Operations Research, 63(1):169–186, 2006
- Breiman L. 1996. Bagging predictors. Mach. Learn. 24:123–40
- Y. Le Tallec. Robust, risk-sensitive, and data-driven control of Markov decision processes. PhD thesis, Massachusetts Institute of Technology, 2007.
- Mnih A, Teh YW. 2012. A fast and simple algorithm for training neural probabilistic language models. In Proceedings of the 29th International Conference on Machine Learning, pp. 419–26. La Jolla, CA: Int. Mach. Learn. Soc.