AUC Score :
Short-term Tactic1 :
Dominant Strategy :
Time series to forecast n:
ML Model Testing : Statistical Inference (ML)
Hypothesis Testing : Factor
Surveillance : Major exchange and OTC
1Short-term revised.
2Time series is updated based on short-term trends.
Key Points
Encore's future prospects appear moderately positive, driven by continued debt purchasing activity and potential improvements in collection efficiency. It is predicted that the company will demonstrate steady revenue growth, albeit at a moderate pace, and maintain profitability due to a stable market for distressed debt. The primary risk factors involve regulatory changes impacting debt collection practices, which could lead to higher compliance costs or limits on collection methods, potentially reducing revenue. Furthermore, economic downturns could negatively impact consumer credit performance, leading to decreased debt availability and reduced returns on purchased debt portfolios. Intense competition within the debt buying industry represents another challenge, as this could compress margins, impacting overall profitability.About Encore Capital Group
Encore Capital (ECPG) is a publicly traded financial services company specializing in the acquisition and management of defaulted consumer debt. The firm operates primarily in the United States and also has a presence in several international markets. Encore Capital purchases portfolios of debt from banks, credit card companies, and other financial institutions at a discount to face value. Subsequently, they attempt to collect on these debts through various methods, including phone calls, letters, and legal action. Their core business model revolves around identifying and acquiring potentially profitable debt portfolios, optimizing collection strategies, and managing the associated risks.
ECPG's operations are significantly influenced by economic cycles, consumer credit trends, and evolving regulatory landscapes. They are subject to regulations regarding debt collection practices, consumer protection, and financial reporting. The company continually invests in technology and analytics to improve collection efficiency and compliance. Encore Capital's business strategy involves maintaining a diversified debt portfolio, optimizing collection processes, and seeking opportunities for growth through acquisitions and expansion into new markets, all while maintaining compliance with debt collection rules.
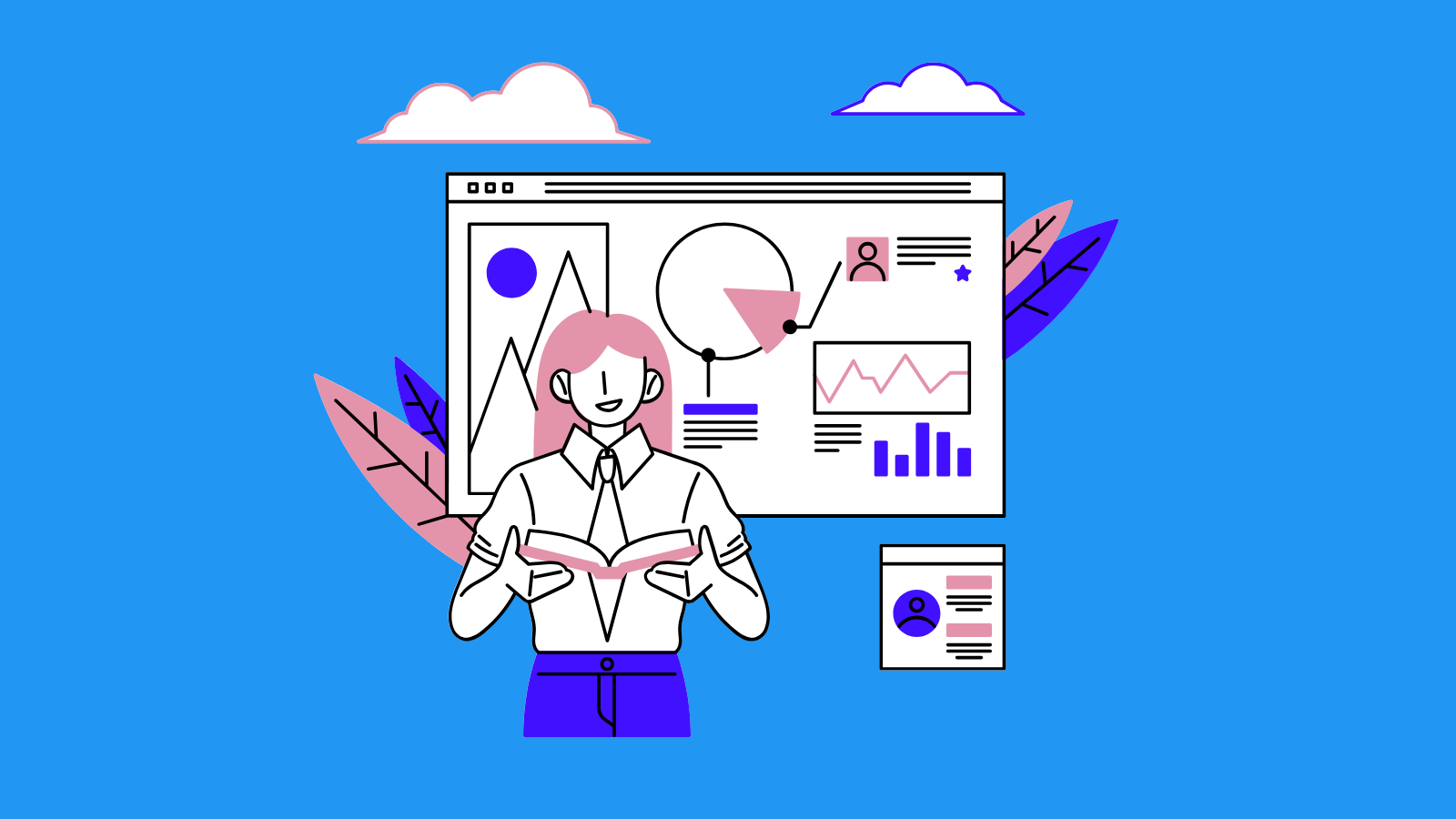
ECPG Stock Forecast Machine Learning Model
Our team of data scientists and economists has developed a machine learning model to forecast the performance of Encore Capital Group Inc. (ECPG) common stock. The core of our model leverages a comprehensive set of features, categorized into several key areas. First, we incorporate historical stock data, including price volatility, trading volume, and moving averages. Secondly, macroeconomic indicators play a significant role. These include interest rates, inflation rates, GDP growth, and consumer confidence indices, which have a demonstrated impact on financial services firms like Encore Capital. Thirdly, we use financial statement data from ECPG, focusing on revenues, earnings per share, debt levels, and cash flow. Furthermore, we consider sentiment analysis from news articles, social media, and financial reports to gauge market perception.
The model employs a ensemble approach, combining the predictive power of several machine learning algorithms. This includes Recurrent Neural Networks (RNNs), particularly LSTMs (Long Short-Term Memory) to capture temporal dependencies in time series data. We also use Gradient Boosting Machines (GBMs), such as XGBoost and LightGBM, known for their ability to handle complex relationships and non-linearities in the data. Before training the model, we apply extensive data preprocessing techniques, including data cleaning, handling missing values, feature scaling, and feature engineering to enhance model accuracy and efficiency. The model is rigorously validated using backtesting and out-of-sample testing. We also employ cross-validation techniques to ensure the model's robustness.
The output of our model is a probabilistic forecast, including a predicted direction for ECPG stock performance (e.g., increase, decrease, or no change), as well as confidence intervals. The forecasts are generated for the upcoming periods. The model is designed to be regularly updated and re-trained with the latest available data to maintain its predictive accuracy and adaptability to changing market conditions. We constantly monitor and refine the model by incorporating new relevant data and feedback loops to address any model drift. Our intention is that the model will provide investors with an analytical advantage in making informed investment decisions related to ECPG common stock.
ML Model Testing
n:Time series to forecast
p:Price signals of Encore Capital Group stock
j:Nash equilibria (Neural Network)
k:Dominated move of Encore Capital Group stock holders
a:Best response for Encore Capital Group target price
For further technical information as per how our model work we invite you to visit the article below:
How do KappaSignal algorithms actually work?
Encore Capital Group Stock Forecast (Buy or Sell) Strategic Interaction Table
Strategic Interaction Table Legend:
X axis: *Likelihood% (The higher the percentage value, the more likely the event will occur.)
Y axis: *Potential Impact% (The higher the percentage value, the more likely the price will deviate.)
Z axis (Grey to Black): *Technical Analysis%
Encore Capital Group Inc. Financial Outlook and Forecast
Encore Capital (ECPG) operates within the consumer debt market, specializing in acquiring and managing defaulted consumer debt portfolios. The company's financial outlook is influenced by several key factors, primarily encompassing the economic climate, regulatory landscape, and its operational efficiency. A robust economy, characterized by low unemployment and manageable inflation, generally benefits ECPG. This is due to an increase in the availability of defaulted debt portfolios for acquisition. Conversely, a recession or economic downturn can reduce the supply of debt available for purchase and increase the risk of consumers defaulting on their existing obligations. Furthermore, ECPG's profitability is tightly linked to its ability to effectively collect on its acquired debt portfolios. This includes managing the costs of collection, which involve legal fees, staffing costs, and the implementation of sophisticated analytics to improve collection rates. The company's ability to adapt to technological advancements and compliance with the constantly changing consumer protection regulations also plays a crucial role.
The regulatory environment presents both opportunities and challenges for ECPG. Stringent regulations focused on consumer protection, such as those related to debt collection practices and data privacy, may elevate operational expenses and potentially reduce collection rates. However, a well-defined regulatory framework, providing clarity and stability, can also foster confidence within the market. ECPG's ability to effectively navigate these complex regulations is essential to preserving its position. Moreover, the competitive landscape within the debt buying industry is quite intense. Several players, including both large and small entities, actively pursue the acquisition of debt portfolios. ECPG's success depends on its capacity to compete effectively in debt acquisition, which can be determined by pricing and the nature of its portfolio selection. Factors such as the quality of debt acquired, the effectiveness of collection strategies, and the efficiency of operational systems are significant.
Considering these factors, the financial forecast for ECPG necessitates a balanced perspective. A key component of any forecast is to understand trends in overall consumer credit health. Changes in consumer debt levels, payment trends, and rates of default provide important context. Moreover, the Company's acquisitions pipeline is crucial for future revenue growth, and the ability to execute strategic acquisitions at attractive prices can significantly impact earnings. Therefore, analysts continually evaluate management's expertise and proficiency in assessing and integrating acquired debt portfolios. Furthermore, the impact of litigation and the resolution of any legal disputes are material. Significant settlements or judgments against the company can adversely affect its financial performance. Finally, investment in technology and data analytics is paramount. ECPG must adapt to technological advancements to improve collection efficiency, reduce costs, and maintain regulatory compliance.
Overall, the outlook for ECPG appears cautiously optimistic, given the current economic environment. The company is well-positioned within its industry, however, success hinges on the company's operational prowess. Risk factors include fluctuations in the macroeconomy, increased regulatory scrutiny, and the competitive intensity of the debt buying landscape. If the economic conditions deteriorate or regulations become too restrictive, the company's profitability could be substantially impacted. Additionally, any failure to adapt to advancements in technology and competition in the debt acquisition market could present a substantial risk. ECPG must demonstrate an ability to efficiently manage debt portfolios, adeptly navigate regulatory challenges, and remain adaptable in order to maintain its financial health.
Rating | Short-Term | Long-Term Senior |
---|---|---|
Outlook | Ba2 | B1 |
Income Statement | Baa2 | B1 |
Balance Sheet | Baa2 | Baa2 |
Leverage Ratios | Caa2 | Caa2 |
Cash Flow | Baa2 | Caa2 |
Rates of Return and Profitability | Ba3 | Caa2 |
*Financial analysis is the process of evaluating a company's financial performance and position by neural network. It involves reviewing the company's financial statements, including the balance sheet, income statement, and cash flow statement, as well as other financial reports and documents.
How does neural network examine financial reports and understand financial state of the company?
References
- Bai J. 2003. Inferential theory for factor models of large dimensions. Econometrica 71:135–71
- R. Rockafellar and S. Uryasev. Conditional value-at-risk for general loss distributions. Journal of Banking and Finance, 26(7):1443 – 1471, 2002
- Zou H, Hastie T. 2005. Regularization and variable selection via the elastic net. J. R. Stat. Soc. B 67:301–20
- R. Rockafellar and S. Uryasev. Optimization of conditional value-at-risk. Journal of Risk, 2:21–42, 2000.
- D. S. Bernstein, S. Zilberstein, and N. Immerman. The complexity of decentralized control of Markov Decision Processes. In UAI '00: Proceedings of the 16th Conference in Uncertainty in Artificial Intelligence, Stanford University, Stanford, California, USA, June 30 - July 3, 2000, pages 32–37, 2000.
- Hill JL. 2011. Bayesian nonparametric modeling for causal inference. J. Comput. Graph. Stat. 20:217–40
- Chernozhukov V, Demirer M, Duflo E, Fernandez-Val I. 2018b. Generic machine learning inference on heteroge- nous treatment effects in randomized experiments. NBER Work. Pap. 24678