AUC Score :
Short-term Tactic1 :
Dominant Strategy :
Time series to forecast n:
ML Model Testing : Reinforcement Machine Learning (ML)
Hypothesis Testing : Wilcoxon Sign-Rank Test
Surveillance : Major exchange and OTC
1Short-term revised.
2Time series is updated based on short-term trends.
Key Points
Crescent Energy's Class A Common Stock is expected to experience moderate growth, fueled by expanding oil and gas production, particularly from its strategic acquisitions. The company's financial performance is predicted to be positive, supported by rising energy prices and efficient operational strategies. However, potential risks include volatility in global oil prices, which could negatively impact revenues and profitability. Additionally, the company faces challenges from stringent environmental regulations and the necessity for continuous capital investments to maintain production levels. Increased interest rates may also negatively affect the company's debt. Despite these potential headwinds, Crescent's diversified asset portfolio and focus on cost management should allow it to weather these challenges, although the company's growth may be slower than some investors expect.About Crescent Energy Company Class A
Crescent Energy, a prominent player in the oil and gas industry, focuses on the acquisition, development, and operation of upstream assets. The company concentrates its activities in major U.S. onshore basins, including the Eagle Ford, Rockies, and Permian Basin. Crescent Energy's operational strategy involves identifying and integrating strategic acquisitions to expand its portfolio and capitalize on production efficiencies. The company aims to deliver value through responsible resource management and operational excellence, focusing on long-term sustainable growth.
Crescent Energy is structured as a company that acquires and manages its assets, driving revenue through production and sales of oil, natural gas, and natural gas liquids. The company emphasizes environmental stewardship and community engagement as integral parts of its operations. Crescent Energy Class A Common Stock represents an ownership stake in the company, allowing investors to participate in its financial performance and growth trajectory within the energy sector.
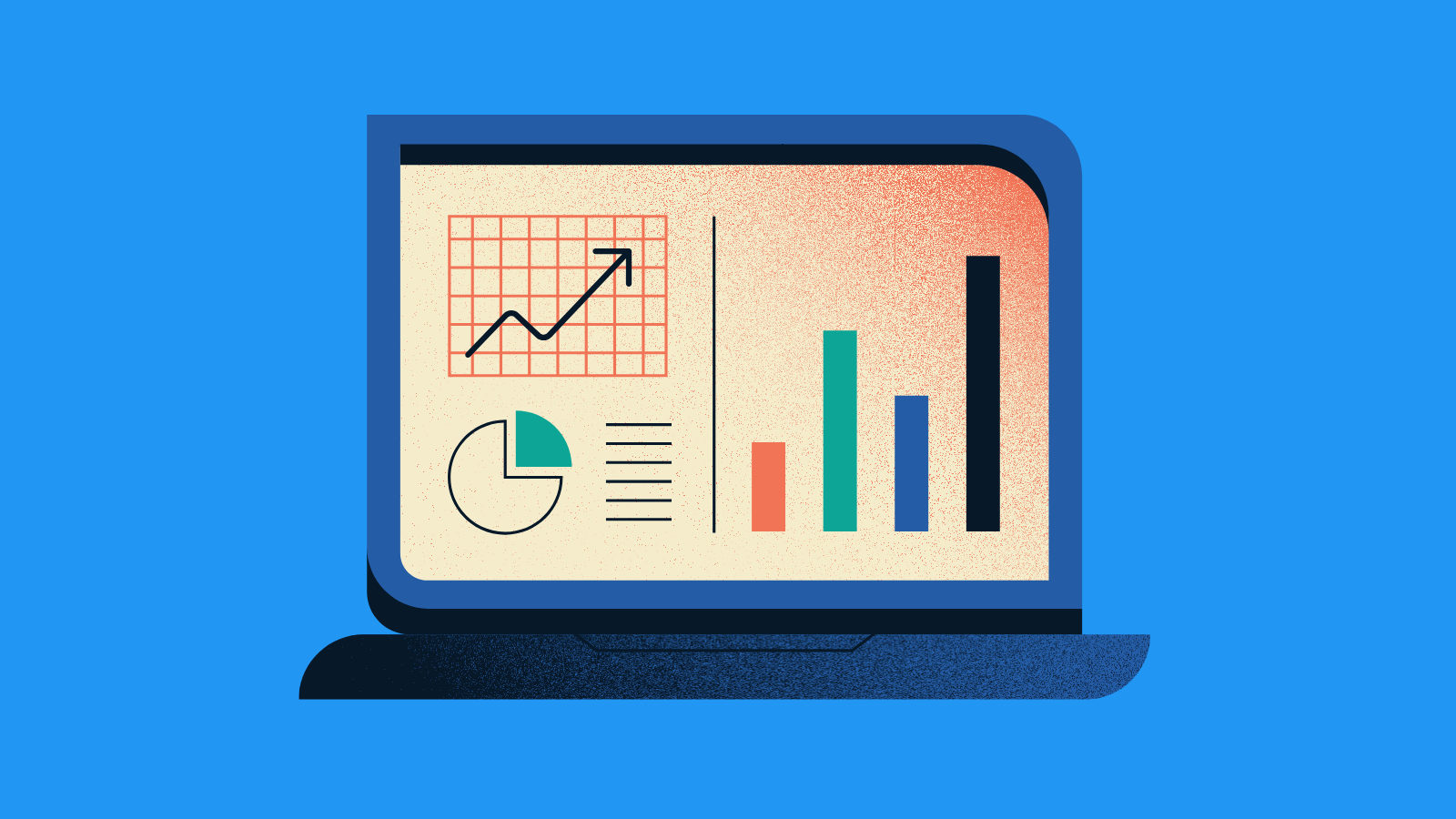
CRGY Stock Forecast Machine Learning Model
Our team of data scientists and economists has developed a machine learning model to forecast the performance of Crescent Energy Company Class A Common Stock (CRGY). The model integrates a diverse set of features categorized into three key areas: historical stock performance data, economic indicators, and company-specific financial metrics. Historical data encompasses technical indicators like moving averages, Relative Strength Index (RSI), and trading volume, along with past stock price movements. Economic indicators incorporated include GDP growth, inflation rates, interest rate fluctuations, and crude oil price trends, which are significant for energy companies. Company-specific financial metrics considered are quarterly and annual revenue, earnings per share (EPS), debt levels, and operational efficiency ratios. We employ a combination of algorithms including Recurrent Neural Networks (RNNs), specifically Long Short-Term Memory (LSTM) networks, to capture temporal dependencies in time-series data, alongside Gradient Boosting methods, such as XGBoost, for their ability to handle complex relationships and non-linearities within the dataset.
The model's training phase involves using historical data spanning the last five years, with a portion reserved for validation and testing. To mitigate the risk of overfitting, we implement various regularization techniques, like dropout layers and L1/L2 regularization, within the neural network architecture. Feature scaling, using methods like Min-Max scaling, is applied to standardize the input data, thereby enhancing the model's convergence and overall performance. Furthermore, hyperparameter optimization is conducted through cross-validation and grid search to determine the optimal settings for each algorithm. The model's output consists of a predicted direction (positive or negative) for the CRGY stock, alongside a confidence score to indicate the certainty of the forecast. Finally, the model's performance is evaluated using metrics such as accuracy, precision, recall, F1-score, and area under the ROC curve (AUC) to ensure the model is reliable and robust.
For continuous improvement and relevance, the model undergoes a rigorous and cyclical process. We regularly retrain the model with updated data, incorporating the latest economic releases and financial reports from Crescent Energy Company. This iterative process includes regular feature engineering to identify and incorporate new factors that may influence stock performance. Moreover, we constantly monitor the model's performance, conducting backtesting with out-of-sample data to assess its predictive power and identify areas for enhancement. We will periodically evaluate and experiment with new machine learning techniques and data sources, remaining adaptable to market dynamics. The final goal of this model is to provide insights for trading decisions, risk management, and strategic planning, acknowledging the inherent uncertainty in financial markets and using these insights as a part of a comprehensive investment strategy.
```
ML Model Testing
n:Time series to forecast
p:Price signals of Crescent Energy Company Class A stock
j:Nash equilibria (Neural Network)
k:Dominated move of Crescent Energy Company Class A stock holders
a:Best response for Crescent Energy Company Class A target price
For further technical information as per how our model work we invite you to visit the article below:
How do KappaSignal algorithms actually work?
Crescent Energy Company Class A Stock Forecast (Buy or Sell) Strategic Interaction Table
Strategic Interaction Table Legend:
X axis: *Likelihood% (The higher the percentage value, the more likely the event will occur.)
Y axis: *Potential Impact% (The higher the percentage value, the more likely the price will deviate.)
Z axis (Grey to Black): *Technical Analysis%
Crescent Energy Company Class A Common Stock: Financial Outlook and Forecast
Crescent, a company focused on oil and natural gas exploration and production in the U.S., demonstrates a financial outlook influenced by fluctuating commodity prices, operational efficiency, and strategic acquisitions. The company's performance is closely tied to the volatile energy market, with revenue and profitability directly linked to global demand and supply dynamics. Recent strategic moves, including mergers and acquisitions, have expanded the company's asset base and production capabilities, aiming to increase its scale and diversify its portfolio across various basins. Furthermore, Crescent's financial strategy includes a focus on debt management and shareholder returns through dividends and potential share repurchases, which can either boost investor confidence or be a liability depending on market conditions. The outlook is further shaped by the company's ability to efficiently integrate acquired assets, optimize production costs, and manage environmental, social, and governance (ESG) factors to ensure long-term sustainability and appeal to investors.
The forecast for Crescent's financial performance hinges on several key factors. First and foremost is the price of oil and natural gas, which is subject to geopolitical events, macroeconomic trends, and weather patterns. A sustained increase in energy prices will likely bolster revenues and improve profitability, while a decline could lead to reduced earnings and cash flow. Secondly, operational efficiency plays a crucial role. Crescent's ability to control production costs, improve drilling efficiencies, and minimize downtime will directly impact its bottom line. Thirdly, the company's capital allocation strategy, including investments in new projects, asset acquisitions, and shareholder returns, is also important. Strategic acquisitions that enhance production and reserve base without significantly increasing debt can positively influence Crescent's value. Moreover, as investors pay more attention to ESG, how Crescent manages environmental risks and integrates sustainable practices, will affect it's long-term financial outlook.
Looking ahead, Crescent is likely to experience a mixture of challenges and opportunities. The company's growth prospects are significantly tied to its ability to effectively integrate recent acquisitions, optimize production from these acquired assets, and sustain cost-effective operations. Maintaining a strong balance sheet is essential, particularly in a volatile energy market, to provide flexibility during price downturns and pursue strategic opportunities. Regulatory changes and the growing emphasis on environmental responsibility will require Crescent to adapt its operations and embrace sustainable practices. Furthermore, Crescent needs to maintain investor confidence by delivering consistent operational performance and demonstrating responsible financial stewardship. The ability to navigate these complexities while simultaneously capitalizing on favorable market conditions will be critical to Crescent's long-term success.
In conclusion, while facing risks associated with energy price fluctuations and regulatory uncertainties, the financial forecast for Crescent is moderately positive. The company's strategic acquisitions and efforts to diversify its asset base should contribute to steady revenue growth and improve its position. The company's success relies on it's capability of maintaining operational efficiency, strategic capital allocation, and responsible financial management. However, downside risks include potential negative impact from a significant drop in energy prices, operational challenges from integrating acquired assets, and increased regulatory scrutiny or compliance costs. For investors, this outlook suggests a cautiously optimistic approach, as Crescent's trajectory is dependent on effective execution amid a dynamic market environment.
Rating | Short-Term | Long-Term Senior |
---|---|---|
Outlook | Ba2 | B2 |
Income Statement | Baa2 | Baa2 |
Balance Sheet | Ba3 | C |
Leverage Ratios | Baa2 | C |
Cash Flow | Ba1 | C |
Rates of Return and Profitability | Caa2 | Ba3 |
*Financial analysis is the process of evaluating a company's financial performance and position by neural network. It involves reviewing the company's financial statements, including the balance sheet, income statement, and cash flow statement, as well as other financial reports and documents.
How does neural network examine financial reports and understand financial state of the company?
References
- O. Bardou, N. Frikha, and G. Pag`es. Computing VaR and CVaR using stochastic approximation and adaptive unconstrained importance sampling. Monte Carlo Methods and Applications, 15(3):173–210, 2009.
- Tibshirani R, Hastie T. 1987. Local likelihood estimation. J. Am. Stat. Assoc. 82:559–67
- M. Sobel. The variance of discounted Markov decision processes. Applied Probability, pages 794–802, 1982
- Zeileis A, Hothorn T, Hornik K. 2008. Model-based recursive partitioning. J. Comput. Graph. Stat. 17:492–514 Zhou Z, Athey S, Wager S. 2018. Offline multi-action policy learning: generalization and optimization. arXiv:1810.04778 [stat.ML]
- Matzkin RL. 1994. Restrictions of economic theory in nonparametric methods. In Handbook of Econometrics, Vol. 4, ed. R Engle, D McFadden, pp. 2523–58. Amsterdam: Elsevier
- A. Tamar, D. Di Castro, and S. Mannor. Policy gradients with variance related risk criteria. In Proceedings of the Twenty-Ninth International Conference on Machine Learning, pages 387–396, 2012.
- O. Bardou, N. Frikha, and G. Pag`es. Computing VaR and CVaR using stochastic approximation and adaptive unconstrained importance sampling. Monte Carlo Methods and Applications, 15(3):173–210, 2009.