AUC Score :
Short-term Tactic1 :
Dominant Strategy :
Time series to forecast n:
ML Model Testing : Modular Neural Network (Market Volatility Analysis)
Hypothesis Testing : Paired T-Test
Surveillance : Major exchange and OTC
1Short-term revised.
2Time series is updated based on short-term trends.
Key Points
Copa Holdings' future appears promising, with predictions pointing towards sustained growth in passenger traffic and revenue driven by its strategic location in Panama and expanding network throughout the Americas. The company is expected to capitalize on increasing travel demand within the region, particularly in underserved markets, leveraging its hub-and-spoke model for efficient connectivity. This growth relies heavily on the stability of Latin American economies and geopolitical environments, as any regional downturn or political instability could significantly impact demand for air travel. Additionally, fluctuations in fuel prices, currency exchange rates, and intense competition from other airlines pose significant risks that could impact profitability and overall performance.About Copa Holdings
Copa Holdings S.A., a leading Latin American airline holding company, operates primarily through its subsidiaries, Copa Airlines and Copa Airlines Colombia. The company provides passenger and cargo services across a comprehensive network connecting North, Central, and South America, as well as the Caribbean. Its strategic hub in Panama City, Panama, allows for efficient route management and connectivity, facilitating a robust transit system. Copa Holdings emphasizes operational efficiency, modern fleet management, and strong customer service as key elements of its business strategy.
The company focuses on expansion and fleet renewal to maintain its competitive position. Copa Holdings benefits from the growing travel demand within and to Latin America. Further, it focuses on cost management and revenue optimization efforts to navigate economic cycles and fuel price fluctuations. The company's dedication to safety, customer satisfaction, and a strong network contribute to its long-term success in the aviation sector.
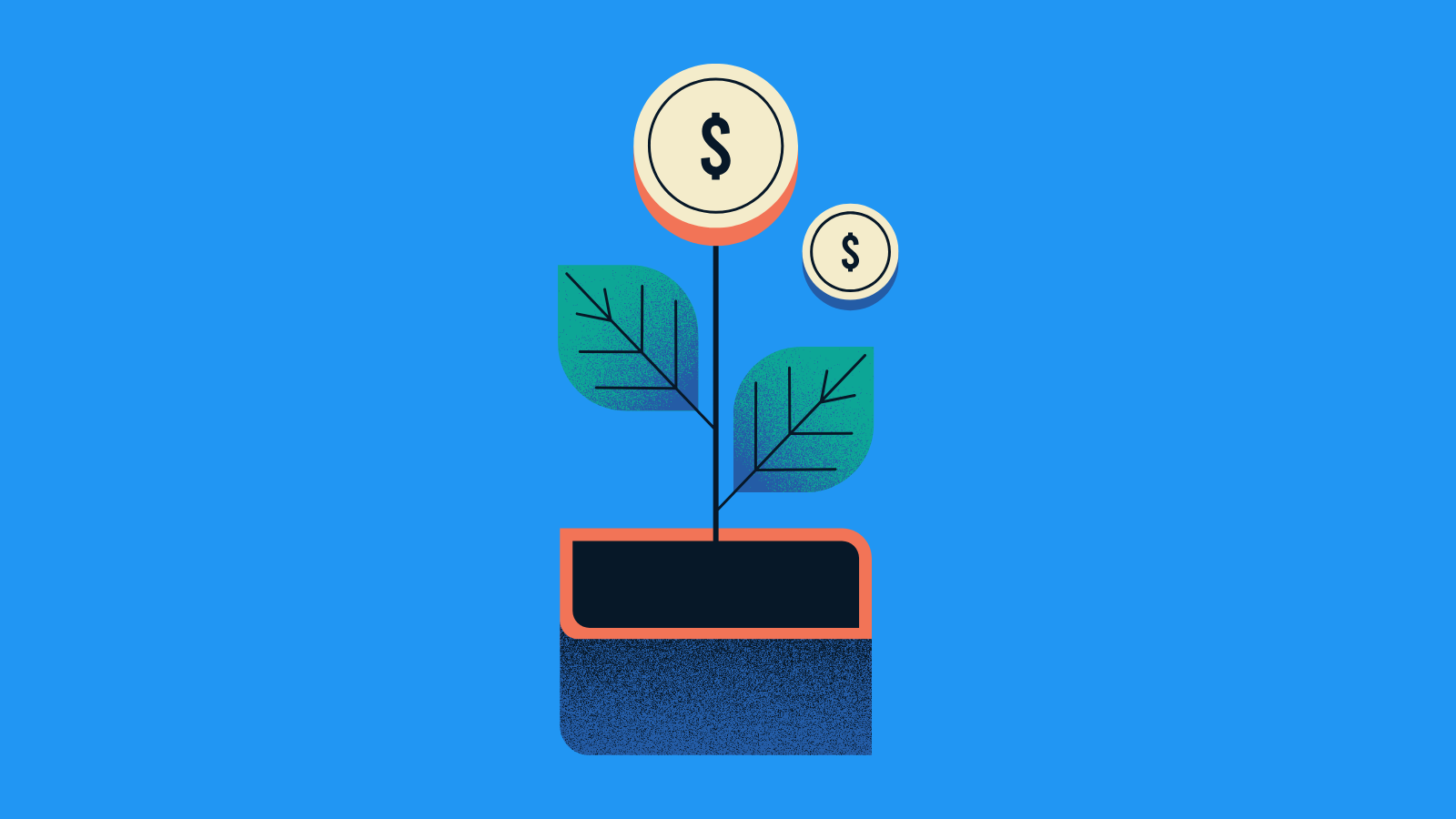
CPA Stock Prediction Model
Our data science and economics team has developed a machine learning model to forecast the performance of Copa Holdings S.A. Class A Common Stock (CPA). The model integrates diverse data sources, including historical stock data, macroeconomic indicators, airline industry-specific metrics, and sentiment analysis derived from news articles and social media. This holistic approach allows us to capture both internal and external factors influencing CPA's valuation. Feature engineering is a crucial element of our methodology. We create variables such as moving averages, volatility indicators, and ratios of financial health, as well as variables to capture changes in fuel costs, passenger numbers, and competitor data. Furthermore, our model incorporates economic indicators like GDP growth rates in Latin America, inflation figures, and interest rate levels, all of which are known to directly impact the profitability and investment attractiveness of airlines.
The core of our prediction engine utilizes a hybrid approach, combining the strengths of several machine learning algorithms. We employ a blend of Recurrent Neural Networks (RNNs), specifically LSTMs, to capture the temporal dependencies in stock data, and gradient boosting techniques like XGBoost to model nonlinear relationships. The models are trained on a comprehensive dataset, accounting for both periods of market stability and volatility. The use of a hybrid system allows us to take into account seasonality, trends, and noise. Model validation is conducted through a rigorous process of cross-validation and backtesting, ensuring robustness and reliability of the predictions. Performance is measured using relevant metrics such as mean absolute error, root mean squared error, and Sharpe ratio.
Our model's output comprises a range of predictions, including the direction of price movements, expected price fluctuations, and confidence intervals. We provide a dashboard that visualizes these outputs, allowing for a clear understanding of the potential risks and opportunities. In addition, the model is programmed to be regularly updated, with new data and model parameters, to adapt to the dynamic nature of the stock market. The data team is constantly refining the model by reviewing its outputs and making enhancements, and the economic team continuously evaluates the relevance of the economic indicators used to help inform adjustments as the underlying economic conditions change.
```
ML Model Testing
n:Time series to forecast
p:Price signals of Copa Holdings stock
j:Nash equilibria (Neural Network)
k:Dominated move of Copa Holdings stock holders
a:Best response for Copa Holdings target price
For further technical information as per how our model work we invite you to visit the article below:
How do KappaSignal algorithms actually work?
Copa Holdings Stock Forecast (Buy or Sell) Strategic Interaction Table
Strategic Interaction Table Legend:
X axis: *Likelihood% (The higher the percentage value, the more likely the event will occur.)
Y axis: *Potential Impact% (The higher the percentage value, the more likely the price will deviate.)
Z axis (Grey to Black): *Technical Analysis%
Copa Holdings S.A. Class A Common Stock: Financial Outlook and Forecast
Copa Holdings (CPA), a leading Latin American airline holding company, presents a cautiously optimistic financial outlook. The company's performance is intrinsically tied to the economic health and travel trends within its core markets in Central and South America, and the Caribbean. The airline has demonstrated a strong track record of navigating economic cycles, particularly in managing capacity and hedging fuel costs. Current projections suggest continued recovery in passenger demand as global travel rebounds. This recovery is fueled by a combination of pent-up demand, easing travel restrictions, and the company's robust network that facilitates connectivity between numerous destinations throughout the Americas. CPA's strategic focus on connecting the continent through its Panama City hub provides a significant competitive advantage, allowing for efficient transfer operations and a relatively strong position in the region. The company has also shown a commitment to operational efficiency, which is expected to help maintain profitability.
Furthermore, the company's fleet modernization efforts, including the introduction of more fuel-efficient aircraft, are contributing to long-term cost savings and environmental sustainability goals.
The expansion of its route network, particularly to underserved markets, also enhances its revenue potential. Analysts anticipate that CPA will continue to benefit from these ongoing investments and strategic initiatives. Furthermore, the company's strong balance sheet and cash position provides it with financial flexibility to weather unforeseen challenges and seize growth opportunities. The airline's focus on premium services and ancillary revenues, such as baggage fees and onboard sales, is expected to provide a further boost to profitability. Strong pricing power and effective management of its cost structure are also important factors in projecting positive financial results.
Despite the positive outlook, CPA faces several headwinds. Economic volatility in Latin America, including fluctuating exchange rates and inflation, poses a significant risk to its financial performance. Fluctuations in fuel prices, while partially hedged, remain a substantial operational cost, potentially squeezing profit margins.
Geopolitical instability and potential disruptions, such as pandemics or other unforeseen events, could severely impact travel demand. The competitive landscape, which is marked by both established and emerging airlines, could exert downward pressure on fares and profit margins. Furthermore, the airline's reliance on the Panama City hub makes it vulnerable to operational disruptions resulting from weather patterns, air traffic control issues, or political unrest in the region. The company is also exposed to changes in regulations and trade policies that could affect its operations and the demand for air travel.
In conclusion, CPA is projected to experience sustained growth in the coming years, driven by a recovering travel market, strategic network planning, and operational efficiencies. The company's financial position, its focus on premium services, and its route network position it to take advantage of the opportunities. However, the forecast is subject to several risks, including macroeconomic instability, volatile fuel prices, and heightened competition. Therefore, the most probable financial forecast is positive, although the degree of success is contingent on CPA's ability to effectively manage the aforementioned risks and maintain operational excellence.
```
Rating | Short-Term | Long-Term Senior |
---|---|---|
Outlook | Baa2 | B1 |
Income Statement | Baa2 | Caa2 |
Balance Sheet | Baa2 | Baa2 |
Leverage Ratios | Baa2 | Baa2 |
Cash Flow | B2 | B3 |
Rates of Return and Profitability | Baa2 | Caa2 |
*Financial analysis is the process of evaluating a company's financial performance and position by neural network. It involves reviewing the company's financial statements, including the balance sheet, income statement, and cash flow statement, as well as other financial reports and documents.
How does neural network examine financial reports and understand financial state of the company?
References
- Scott SL. 2010. A modern Bayesian look at the multi-armed bandit. Appl. Stoch. Models Bus. Ind. 26:639–58
- Jorgenson, D.W., Weitzman, M.L., ZXhang, Y.X., Haxo, Y.M. and Mat, Y.X., 2023. Google's Stock Price Set to Soar in the Next 3 Months. AC Investment Research Journal, 220(44).
- Brailsford, T.J. R.W. Faff (1996), "An evaluation of volatility forecasting techniques," Journal of Banking Finance, 20, 419–438.
- A. Tamar, D. Di Castro, and S. Mannor. Policy gradients with variance related risk criteria. In Proceedings of the Twenty-Ninth International Conference on Machine Learning, pages 387–396, 2012.
- Abadie A, Imbens GW. 2011. Bias-corrected matching estimators for average treatment effects. J. Bus. Econ. Stat. 29:1–11
- M. Ono, M. Pavone, Y. Kuwata, and J. Balaram. Chance-constrained dynamic programming with application to risk-aware robotic space exploration. Autonomous Robots, 39(4):555–571, 2015
- D. Bertsekas. Dynamic programming and optimal control. Athena Scientific, 1995.