AUC Score :
Short-term Tactic1 :
Dominant Strategy :
Time series to forecast n:
ML Model Testing : Modular Neural Network (DNN Layer)
Hypothesis Testing : Independent T-Test
Surveillance : Major exchange and OTC
1Short-term revised.
2Time series is updated based on short-term trends.
Key Points
BHLD's future appears cautiously optimistic, contingent on successful execution of its digital experience platform strategy. Predictions suggest potential revenue growth stemming from increased demand for digital solutions and the company's ability to secure and retain clients. Strong expansion within the e-commerce sector could further boost performance, especially as businesses prioritize online presence. However, several risks exist. Intense competition from established technology companies and smaller, agile firms poses a significant challenge. Economic downturns could curtail client spending on digital projects, impacting revenue. Furthermore, BHLD's ability to integrate acquisitions seamlessly and manage its cost structure effectively will be crucial. The company must innovate and evolve its offerings to retain its competitive edge within the dynamic tech landscape.About Bridgeline Digital
Bridgeline Digital, Inc. (BLIN) is a software and services company that specializes in digital experience management. Founded in 2000, the company provides cloud-based web content management, e-commerce, and marketing automation solutions. Its products are designed to help businesses enhance their online presence, improve customer engagement, and drive revenue growth. The company primarily serves mid-market and enterprise-level businesses across various industries.
BLIN's core offerings include iAPPS, a platform for managing web content, commerce, and marketing campaigns, as well as related professional services such as implementation, training, and ongoing support. Bridgeline focuses on providing its clients with tools to streamline their digital operations, improve user experiences, and achieve their business objectives through technology. The company operates with a focus on innovation and customer service, striving to deliver value to its customers in an evolving digital landscape.
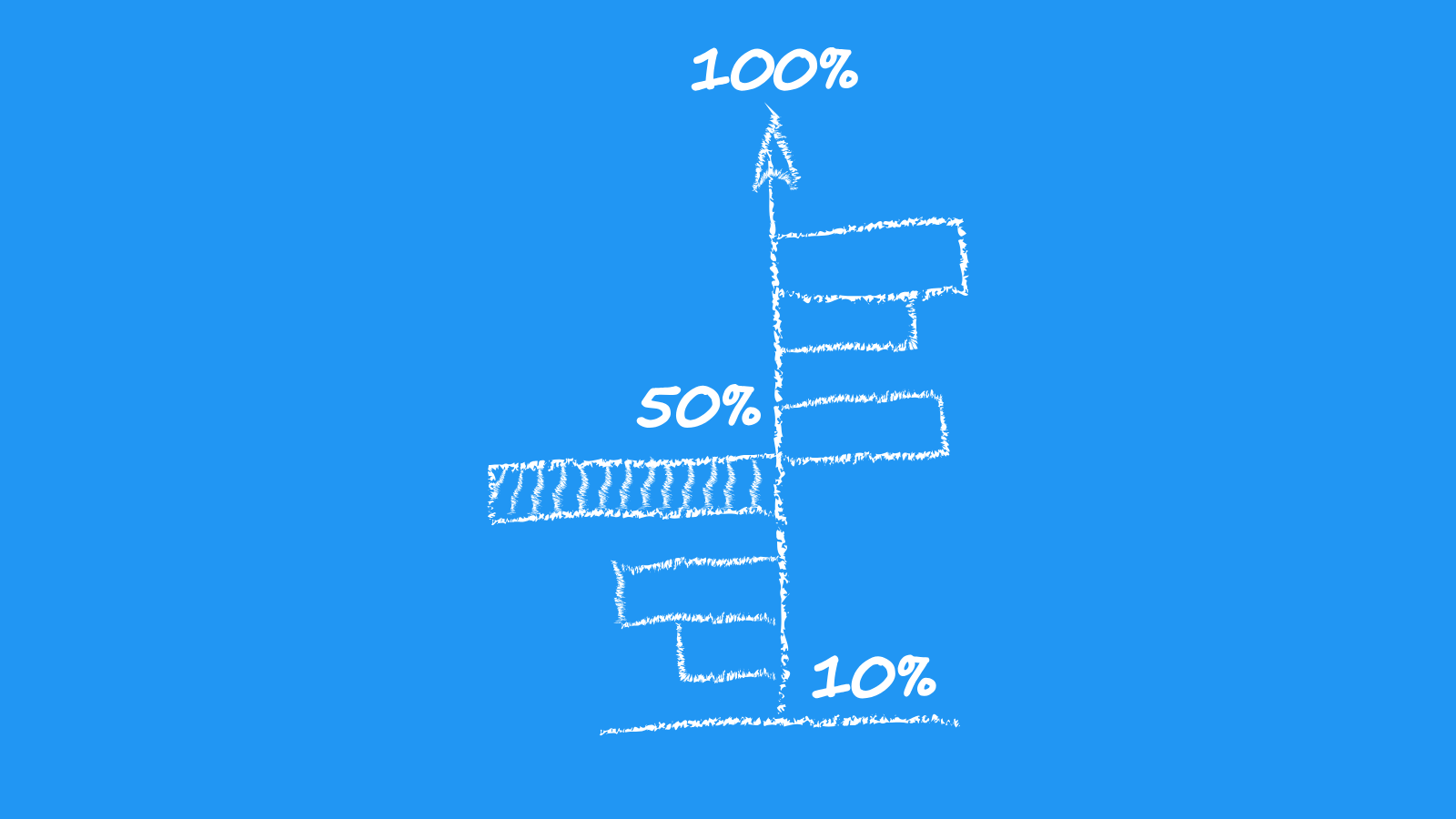
BLIN Stock Forecast Machine Learning Model
Our team of data scientists and economists has developed a machine learning model to forecast the performance of Bridgeline Digital Inc. (BLIN) common stock. The model utilizes a comprehensive set of financial and economic indicators, including but not limited to: historical stock price data, trading volume, company financial statements (revenue, earnings, debt-to-equity ratio), industry-specific metrics (e.g., digital marketing spending), macroeconomic indicators (GDP growth, inflation rates, interest rates), and sentiment analysis of news articles and social media regarding BLIN and its industry. The model selection process involves exploring various algorithms, such as time series models (ARIMA, SARIMA), recurrent neural networks (LSTMs), and ensemble methods (Random Forests, Gradient Boosting). We evaluate these models based on performance metrics like mean squared error (MSE), root mean squared error (RMSE), and mean absolute error (MAE) using a rolling-window cross-validation approach to ensure robustness and generalizability.
The model's architecture consists of multiple layers of feature engineering and model training. Feature engineering is crucial and includes techniques like calculating technical indicators (moving averages, RSI, MACD), transforming financial statement data to growth rates and ratios, and incorporating economic forecasts. We employ dimensionality reduction techniques like Principal Component Analysis (PCA) to manage multicollinearity and reduce noise. The training phase involves optimizing model parameters using historical data and validating performance on unseen data. We also integrate a risk management component, estimating the probability of extreme events using techniques like Value at Risk (VaR) and Expected Shortfall (ES). Finally, the model's output is a probability distribution of potential stock performance, allowing us to provide a range of possible outcomes rather than a single point forecast.
To ensure the model's continued relevance and accuracy, we implement a rigorous monitoring and updating strategy. The model's performance is tracked regularly and recalibrated using new data and potentially refined features. We conduct periodic backtesting and stress testing to evaluate its robustness under different market conditions. Moreover, we incorporate feedback from financial analysts and market experts to enhance our understanding of the stock's behavior and incorporate any new information that may impact its future performance. We also monitor regulatory changes and industry trends that can affect the company's prospects. This holistic approach allows us to provide insightful forecasts, informing investment decisions and risk management for BLIN stock.
ML Model Testing
n:Time series to forecast
p:Price signals of Bridgeline Digital stock
j:Nash equilibria (Neural Network)
k:Dominated move of Bridgeline Digital stock holders
a:Best response for Bridgeline Digital target price
For further technical information as per how our model work we invite you to visit the article below:
How do KappaSignal algorithms actually work?
Bridgeline Digital Stock Forecast (Buy or Sell) Strategic Interaction Table
Strategic Interaction Table Legend:
X axis: *Likelihood% (The higher the percentage value, the more likely the event will occur.)
Y axis: *Potential Impact% (The higher the percentage value, the more likely the price will deviate.)
Z axis (Grey to Black): *Technical Analysis%
Bridgeline Digital Inc. (BLIN) Financial Outlook and Forecast
The financial outlook for BLIN is shaped by its position in the digital experience management (DXM) space, a market that is experiencing continued growth driven by the increasing importance of online presence and customer engagement. BLIN provides cloud-based solutions, including web content management, e-commerce, and marketing automation tools, catering to businesses aiming to enhance their digital strategies. The company's ability to secure and retain customers is critical for its success. BLIN's financial performance depends on its ability to differentiate its offerings and compete effectively. Positive factors include the trend toward digital transformation, expansion of its platform's capabilities, and potential for strategic partnerships or acquisitions.
Forecasts for BLIN's revenue and profitability are subject to several factors. The company's subscription-based business model provides recurring revenue streams, offering a degree of stability. Growth is fueled by new customer acquisition, upselling existing customers, and expansion into new markets. The company's ability to cross-sell its different services is also key. Market analysts forecast moderate revenue growth over the next few years, driven by its product offerings and its ability to integrate with larger digital platforms. Profitability is affected by the cost of goods sold, operational expenses, and investment in research and development. Increased automation and operational efficiencies can also impact profitability. The company's focus on cloud services will influence both its top-line growth and its cost structure. It is important to consider the company's competitive environment, including the pricing strategies of its competitors.
Several key indicators will shape BLIN's financial performance. Customer acquisition costs and customer lifetime value (CLTV) are crucial metrics. Higher CLTV indicates the effectiveness of its solutions. Churn rates, the percentage of customers discontinuing their services, are important; a low churn rate is indicative of strong customer satisfaction. BLIN's ability to maintain a competitive edge requires ongoing innovation and investment in its product roadmap. Key performance indicators (KPIs) to monitor will include customer acquisition costs, churn rates, and the percentage of revenue generated from new services. BLIN must also prioritize efficiency and cost management to optimize its margins. The impact of macroeconomic conditions, such as interest rates and inflation, also influences the outlook for the company, especially on its financing needs.
Overall, the financial forecast for BLIN is cautiously optimistic, with moderate growth and improved profitability. However, this prediction faces several risks. Competition within the DXM space is intense, with established players and new entrants competing for market share. BLIN must continually innovate to remain competitive, which involves significant investment and the risk of failing to anticipate market needs. Another risk is the reliance on a limited number of customers. Economic downturns may slow down customer spending on digital transformation initiatives. Strategic execution risk and the risk of delays in development also exist. ```
Rating | Short-Term | Long-Term Senior |
---|---|---|
Outlook | B2 | Ba1 |
Income Statement | B3 | Baa2 |
Balance Sheet | Baa2 | Ba3 |
Leverage Ratios | Ba3 | Baa2 |
Cash Flow | C | Ba3 |
Rates of Return and Profitability | C | B1 |
*Financial analysis is the process of evaluating a company's financial performance and position by neural network. It involves reviewing the company's financial statements, including the balance sheet, income statement, and cash flow statement, as well as other financial reports and documents.
How does neural network examine financial reports and understand financial state of the company?
References
- Breiman L. 2001b. Statistical modeling: the two cultures (with comments and a rejoinder by the author). Stat. Sci. 16:199–231
- Athey S, Blei D, Donnelly R, Ruiz F. 2017b. Counterfactual inference for consumer choice across many prod- uct categories. AEA Pap. Proc. 108:64–67
- Athey S, Imbens G. 2016. Recursive partitioning for heterogeneous causal effects. PNAS 113:7353–60
- J. Hu and M. P. Wellman. Nash q-learning for general-sum stochastic games. Journal of Machine Learning Research, 4:1039–1069, 2003.
- F. A. Oliehoek, M. T. J. Spaan, and N. A. Vlassis. Optimal and approximate q-value functions for decentralized pomdps. J. Artif. Intell. Res. (JAIR), 32:289–353, 2008
- Dudik M, Langford J, Li L. 2011. Doubly robust policy evaluation and learning. In Proceedings of the 28th International Conference on Machine Learning, pp. 1097–104. La Jolla, CA: Int. Mach. Learn. Soc.
- Harris ZS. 1954. Distributional structure. Word 10:146–62