AUC Score :
Short-term Tactic1 :
Dominant Strategy :
Time series to forecast n:
ML Model Testing : Reinforcement Machine Learning (ML)
Hypothesis Testing : Spearman Correlation
Surveillance : Major exchange and OTC
1Short-term revised.
2Time series is updated based on short-term trends.
Key Points
BlackSky's stock faces a mixed outlook. The company's focus on high-frequency satellite imagery and analytics presents significant growth potential, especially within government and commercial sectors requiring real-time intelligence. However, BlackSky operates in a competitive landscape with established players and faces risks including execution challenges in scaling its satellite constellation, securing consistent government contracts, and maintaining technological advancement. The stock's performance hinges on the company's ability to successfully integrate its acquisitions, effectively manage its debt, and demonstrate sustainable profitability, which are key drivers for investor confidence.About BlackSky Technology
BlackSky Technology Inc., a geospatial intelligence provider, utilizes a constellation of Earth observation satellites and a software platform to deliver real-time, high-resolution imagery and analytics. The company focuses on providing insights related to global events, infrastructure monitoring, and other areas where timely, data-driven decisions are crucial. Their services cater to governmental and commercial clients, offering capabilities like identifying changes in infrastructure, tracking global events, and supporting disaster response.
BlackSky's business model centers around providing on-demand, space-based intelligence. It differentiates itself through its rapid image acquisition and analysis capabilities, which allow customers to access data and insights swiftly. The company aims to enhance decision-making across various sectors by providing actionable intelligence derived from its satellite constellation and analytics platform. BlackSky continues to develop its capabilities and expand its customer base to capitalize on the growing demand for geospatial intelligence.
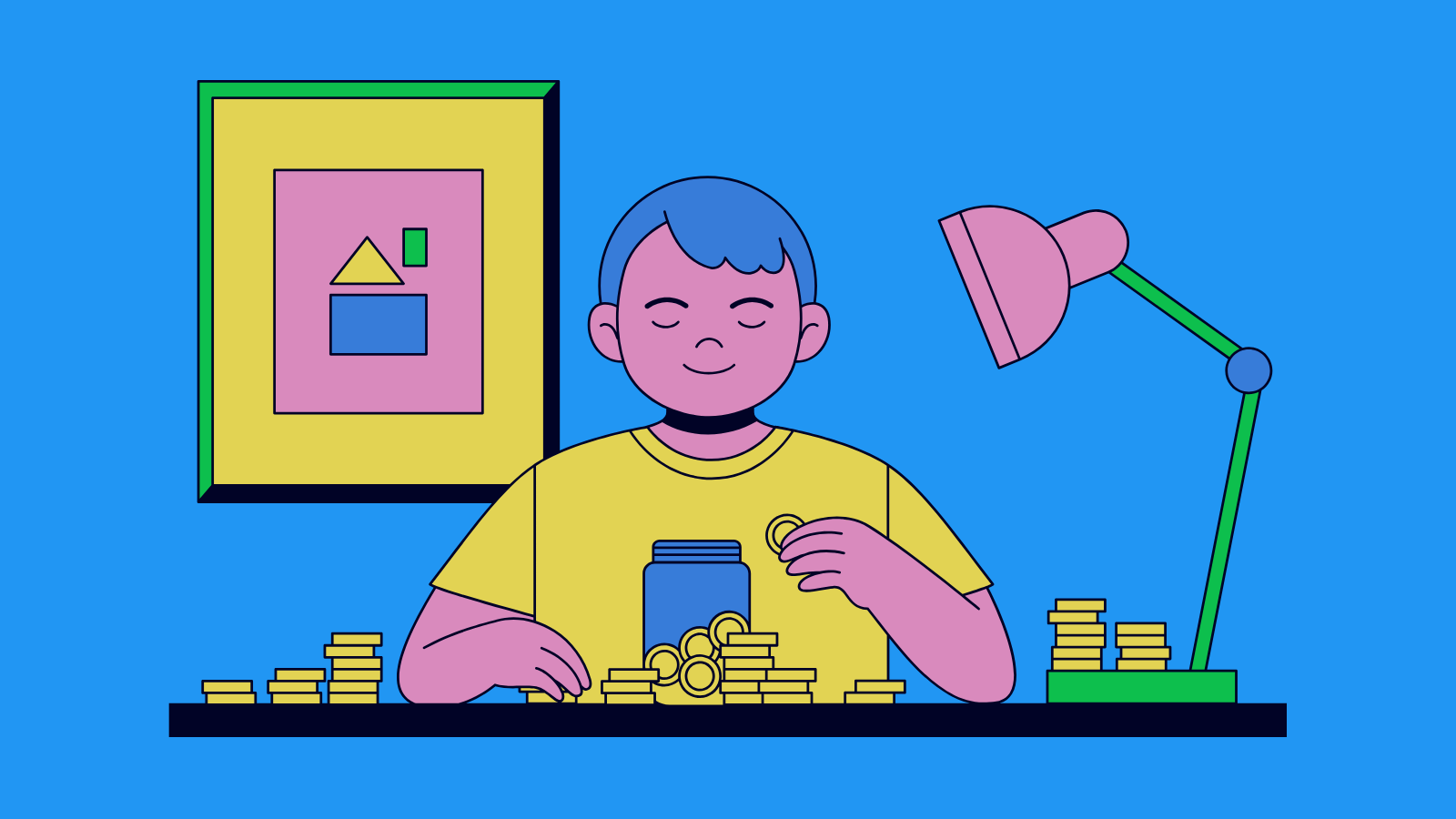
BKSY Stock Prediction: A Machine Learning Model Approach
Our team of data scientists and economists proposes a comprehensive machine learning model for forecasting the future performance of BlackSky Technology Inc. Class A Common Stock (BKSY). This model will leverage a diverse dataset encompassing historical stock trading data (volume, open, close, high, low), fundamental financial metrics (revenue, earnings per share, debt-to-equity ratio), and external macroeconomic indicators (GDP growth, inflation rates, interest rates) to capture the multi-faceted drivers of BKSY's stock behavior. We will employ a combination of supervised learning techniques, including Recurrent Neural Networks (RNNs), specifically LSTMs (Long Short-Term Memory), known for their ability to process sequential data and identify temporal patterns. Additionally, we will integrate ensemble methods like Random Forests or Gradient Boosting to enhance prediction accuracy and robustness by combining the strengths of multiple models. Feature engineering will play a crucial role; we will derive technical indicators such as moving averages, RSI, and MACD from the trading data, and consider sentiment analysis of news articles and social media to gauge investor sentiment, a potentially strong predictor of short-term price movements.
The model's development will involve a rigorous methodology. First, the data will be meticulously cleaned, preprocessed, and normalized to ensure data quality and consistency. Then, we will divide the dataset into training, validation, and test sets. The training set will be used to train the machine learning models, the validation set will tune hyperparameters and prevent overfitting, and the test set will evaluate the model's predictive performance on unseen data. We will employ cross-validation techniques to ensure the model's generalizability. The model's performance will be assessed using various metrics appropriate for time series forecasting, including Mean Absolute Error (MAE), Root Mean Squared Error (RMSE), and R-squared. The most important part is backtesting in different market scenarios to identify the model's limitations and refine its parameters for optimal performance, which involves simulating its performance on past data to assess its accuracy and reliability.
The final output of this project will be a forecasting model, providing probabilistic predictions of BKSY's future stock performance, and accompanying visualizations. These outputs will include forecasted price movements, volatility estimates, and confidence intervals. To facilitate the model's integration and usability, we aim to build an interactive dashboard enabling easy monitoring and analysis of predictions, along with real-time data feeds. The model will be continuously monitored and updated with new data and re-trained periodically to maintain its predictive accuracy and adapt to changing market conditions. We will document all code, data processing steps, model parameters, and results with full transparency. The intention is to equip BlackSky with a sophisticated tool to inform strategic decisions, manage risk, and help investors make well-informed trading decisions. This is a predictive model, not an investment advise.
```
ML Model Testing
n:Time series to forecast
p:Price signals of BlackSky Technology stock
j:Nash equilibria (Neural Network)
k:Dominated move of BlackSky Technology stock holders
a:Best response for BlackSky Technology target price
For further technical information as per how our model work we invite you to visit the article below:
How do KappaSignal algorithms actually work?
BlackSky Technology Stock Forecast (Buy or Sell) Strategic Interaction Table
Strategic Interaction Table Legend:
X axis: *Likelihood% (The higher the percentage value, the more likely the event will occur.)
Y axis: *Potential Impact% (The higher the percentage value, the more likely the price will deviate.)
Z axis (Grey to Black): *Technical Analysis%
BlackSky Technology Inc. Financial Outlook and Forecast
BlackSky's outlook is intricately tied to its ability to capitalize on the burgeoning demand for real-time geospatial intelligence and its execution of its growth strategy. The company is strategically positioned within the rapidly expanding space-based data and analytics market, catering to both government and commercial clients.
Its competitive advantage lies in its constellation of high-resolution satellites and its proprietary software platform, Spectra AI, which enables rapid data collection, processing, and analysis. The core of its strategy involves scaling its satellite constellation, increasing data collection capabilities, and expanding its customer base across various sectors, including defense, intelligence, infrastructure, and environmental monitoring. BlackSky aims to transform raw satellite imagery into actionable intelligence, facilitating timely decision-making for its clients. Additionally, its service-based model, with recurring revenue streams from subscription services, provides stability and predictability to its financial performance. The company is also actively exploring strategic partnerships and acquisitions to enhance its technology and market reach.
The financial forecast for BlackSky is expected to be driven by its expansion plans. Key drivers for revenue growth include the deployment of additional satellites, increasing data availability, and expanding its sales and marketing efforts. The growth prospects are predicated on its ability to secure significant contracts with governmental and commercial customers. Projections typically envision a significant increase in revenue over the coming years, reflecting the anticipated market expansion and the growing adoption of its services.
Profitability, however, remains a key concern, with a projected trajectory towards profitability as the company achieves economies of scale. Investments in the satellite constellation and Spectra AI platform have led to substantial capital expenditures, impacting near-term earnings. Management has signaled a focus on cost optimization and operational efficiency, with the aim of improving margins and ultimately achieving sustainable profitability. Analysts will likely closely monitor metrics such as backlog, customer acquisition cost, and revenue per user to gauge the company's success in executing its growth plan.
BlackSky's forecast is influenced by several operational aspects. The company's operational success hinges on its capacity to launch and operate its satellite constellation effectively, maintain data quality, and continuously innovate its technology. The ability to secure reliable and cost-effective launch services is critical for expanding the satellite network. The ongoing development of Spectra AI and its integration with its satellite data is essential for differentiation.
The strength of BlackSky's sales and marketing efforts will play a pivotal role in customer acquisition and retention. Furthermore, the ability to adapt to rapidly evolving market needs and technological advancements is imperative. The company is also vulnerable to competition from established players and other emerging companies in the geospatial intelligence market. Strong relationships with government agencies and commercial entities are crucial for generating revenue and fostering sustainable growth. Maintaining stringent cybersecurity measures is also important to safeguard the integrity of data and customer relationships.
Considering the factors mentioned, the prediction for BlackSky is moderately positive. The anticipated increase in demand for real-time geospatial intelligence and its strategic positioning in a high-growth market, combined with the expansion of the satellite constellation and the continuous development of its software platform, support the prospects for substantial revenue growth in the medium to long term. However, the company faces significant risks.
Key risks include the challenges associated with satellite launches, competitive pressures, the potential for delays in the deployment of new satellites, and the need for continuous technological advancements. Moreover, the company's profitability faces challenges, especially related to the upfront costs for the space segment. The ability to secure sufficient funding to support its growth strategy is also essential. Investors should also carefully monitor the competitive environment, regulatory changes, and potential macroeconomic factors that could influence BlackSky's financial performance.
Rating | Short-Term | Long-Term Senior |
---|---|---|
Outlook | B1 | Ba3 |
Income Statement | Caa2 | C |
Balance Sheet | C | Baa2 |
Leverage Ratios | Baa2 | B1 |
Cash Flow | Ba3 | Baa2 |
Rates of Return and Profitability | Baa2 | B2 |
*Financial analysis is the process of evaluating a company's financial performance and position by neural network. It involves reviewing the company's financial statements, including the balance sheet, income statement, and cash flow statement, as well as other financial reports and documents.
How does neural network examine financial reports and understand financial state of the company?
References
- Burgess, D. F. (1975), "Duality theory and pitfalls in the specification of technologies," Journal of Econometrics, 3, 105–121.
- Varian HR. 2014. Big data: new tricks for econometrics. J. Econ. Perspect. 28:3–28
- M. J. Hausknecht. Cooperation and Communication in Multiagent Deep Reinforcement Learning. PhD thesis, The University of Texas at Austin, 2016
- Chernozhukov V, Chetverikov D, Demirer M, Duflo E, Hansen C, et al. 2018a. Double/debiased machine learning for treatment and structural parameters. Econom. J. 21:C1–68
- Mnih A, Kavukcuoglu K. 2013. Learning word embeddings efficiently with noise-contrastive estimation. In Advances in Neural Information Processing Systems, Vol. 26, ed. Z Ghahramani, M Welling, C Cortes, ND Lawrence, KQ Weinberger, pp. 2265–73. San Diego, CA: Neural Inf. Process. Syst. Found.
- Mnih A, Hinton GE. 2007. Three new graphical models for statistical language modelling. In International Conference on Machine Learning, pp. 641–48. La Jolla, CA: Int. Mach. Learn. Soc.
- Challen, D. W. A. J. Hagger (1983), Macroeconomic Systems: Construction, Validation and Applications. New York: St. Martin's Press.