AUC Score :
Short-term Tactic1 :
Dominant Strategy :
Time series to forecast n:
ML Model Testing : Reinforcement Machine Learning (ML)
Hypothesis Testing : ElasticNet Regression
Surveillance : Major exchange and OTC
1Short-term revised.
2Time series is updated based on short-term trends.
Key Points
Apogee Enterprises faces a mixed outlook. Predictions suggest modest revenue growth driven by its architectural glass and framing segments, potentially boosted by increased construction activity. Furthermore, streamlining operations could marginally improve profitability. However, Apogee's performance is exposed to several risks. Economic downturns could negatively impact demand for its products, particularly in the construction sector. Fluctuations in raw material costs and supply chain disruptions could also compress profit margins. Intensified competition within the architectural glass market poses a threat to market share and pricing power. Regulatory changes and environmental concerns surrounding the construction industry might further complicate business operations.About Apogee Enterprises Inc.
Apogee Enterprises, Inc. is a prominent player in the architectural glass and framing systems industry. The company designs, engineers, and installs architectural glass products and services for commercial buildings. Its operations are segmented into four primary business units: Architectural Glass, Architectural Framing Systems, Architectural Services, and Large-Scale Optical Technologies. These divisions cater to various architectural needs, including curtain walls, storefronts, and other specialized glass applications, as well as related services like installation and design support. The company's products are often found in high-profile commercial construction projects across North America and beyond.
Founded in 1949, Apogee has a long-standing history in the glass industry. Through strategic acquisitions and internal growth, the company has expanded its capabilities and geographic reach over the decades. Apogee's commitment to innovation and sustainability plays a role in its operations and product offerings. The company aims to provide advanced solutions that meet the evolving demands of the construction market while also considering environmental factors.
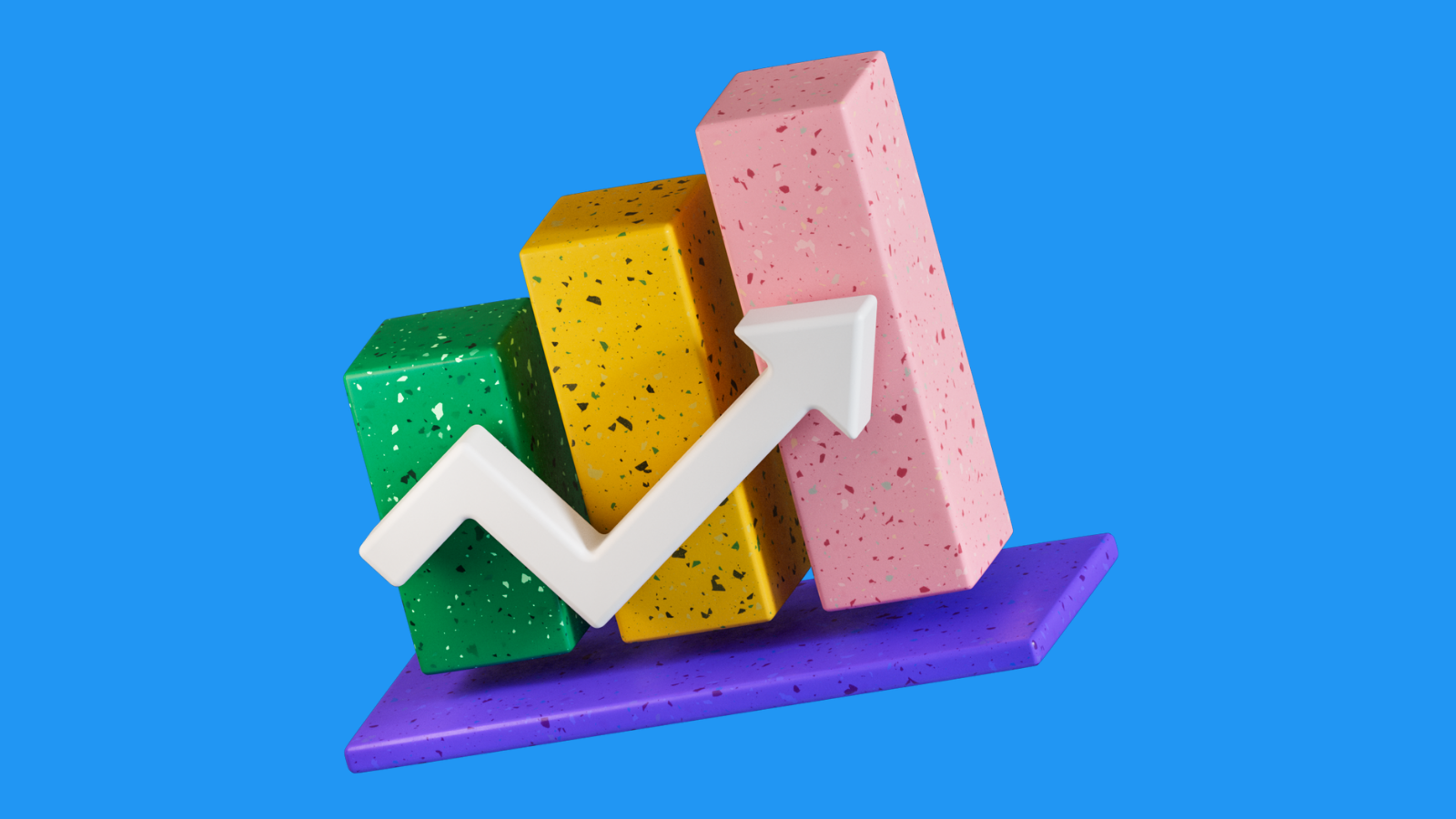
APOG Stock Forecasting Model
Our team of data scientists and economists has developed a machine learning model to forecast the performance of Apogee Enterprises Inc. (APOG) common stock. This model integrates diverse data sources, including historical stock prices, financial statements (revenue, earnings per share, debt levels), macroeconomic indicators (GDP growth, inflation rates, interest rates), industry-specific factors (construction spending, architectural trends), and sentiment analysis derived from news articles and social media discussions. We utilize a combination of techniques, including time series analysis (e.g., ARIMA models, LSTM networks) to capture temporal dependencies, regression models to assess the impact of financial and macroeconomic variables, and natural language processing to gauge market sentiment. Feature engineering plays a crucial role in refining the model, as we create composite variables and transformations to enhance predictive accuracy.
The model undergoes rigorous training and validation processes. We split the historical data into training, validation, and testing sets, ensuring a robust evaluation of the model's performance. We employ cross-validation techniques to mitigate overfitting and assess the model's generalization ability. Performance metrics such as Mean Absolute Error (MAE), Root Mean Squared Error (RMSE), and R-squared are used to evaluate the model's predictive power. Furthermore, we conduct scenario analysis by simulating various economic conditions and industry trends to assess the model's sensitivity and robustness. Model outputs are regularly updated and refined as new data becomes available.
The final output of the model provides a probabilistic forecast for APOG's stock performance over specified time horizons. We generate both point estimates (e.g., expected future value) and confidence intervals. The model's output includes an assessment of the key drivers influencing APOG's stock behavior, helping us understand the factors behind the predicted price movement. This enables investors to make informed decisions. It is important to recognize that these are predictions, and market performance is subject to uncertainty. Therefore, the model is designed to be a tool that enhances decision-making but should not be the sole basis for investment strategies. Regular monitoring and recalibration based on ongoing market dynamics are essential.
ML Model Testing
n:Time series to forecast
p:Price signals of Apogee Enterprises Inc. stock
j:Nash equilibria (Neural Network)
k:Dominated move of Apogee Enterprises Inc. stock holders
a:Best response for Apogee Enterprises Inc. target price
For further technical information as per how our model work we invite you to visit the article below:
How do KappaSignal algorithms actually work?
Apogee Enterprises Inc. Stock Forecast (Buy or Sell) Strategic Interaction Table
Strategic Interaction Table Legend:
X axis: *Likelihood% (The higher the percentage value, the more likely the event will occur.)
Y axis: *Potential Impact% (The higher the percentage value, the more likely the price will deviate.)
Z axis (Grey to Black): *Technical Analysis%
Apogee Enterprises Inc. (APOG) Financial Outlook and Forecast
The financial outlook for APOG appears cautiously optimistic, reflecting a recovery trajectory from recent headwinds. The company's diversified business model, spanning architectural glass, architectural services, and large-scale optical technologies, positions it favorably to capitalize on various market segments. Growth in non-residential construction, particularly in the United States, represents a core driver for its architectural glass and services divisions. Increased infrastructure spending, fueled by government initiatives, is also expected to provide a tailwind. Furthermore, the company is strategically focused on operational efficiency and cost management, aiming to improve profitability margins. While economic uncertainties linger, APOG's strategic initiatives and diversified portfolio provide a buffer against significant downturns. Its ongoing commitment to innovation in its product offerings, especially in areas like energy-efficient glass and advanced coatings, strengthens its competitive positioning and potential for long-term growth.
Several key factors are projected to influence APOG's financial performance in the coming periods. Firstly, the cyclical nature of the construction industry introduces inherent volatility. Economic slowdowns or shifts in construction spending patterns could negatively impact revenue growth. Secondly, fluctuations in raw material costs, particularly for glass and related components, pose a potential risk to profitability. The company's ability to manage these costs effectively through hedging strategies and supplier negotiations will be crucial. Thirdly, the company's success hinges on its capacity to secure and execute significant architectural projects. The timing and size of these projects can significantly affect quarterly and annual earnings. Geographic diversification, particularly within North America, helps mitigate concentration risks but exposes it to differing regional economic conditions. Additionally, competitive pressures from other manufacturers and service providers in the glass and building solutions market are ever-present.
Financial analysts generally anticipate moderate revenue growth and improving profitability margins for APOG over the next one to two years. This positive outlook is underpinned by the expectation of continued recovery in the construction sector, combined with the company's ongoing efficiency initiatives. Increased demand for energy-efficient building solutions is expected to favor APOG, given its advanced product offerings. Earnings per share are likely to reflect these improved margins and any share repurchases undertaken by the company. The company's robust balance sheet and prudent financial management provide a solid foundation for sustained growth, and they give them the financial flexibility to navigate potential economic challenges. Capital allocation decisions, including investments in innovation and strategic acquisitions, will be critical in shaping long-term value creation.
In conclusion, the financial forecast for APOG is positive, underpinned by the expected recovery in the construction market, strategic cost management initiatives, and continued innovation. The prediction is for modest revenue growth and improved profitability margins in the upcoming periods. However, the company faces risks, including the cyclical nature of the construction industry, fluctuations in raw material costs, and competitive pressures. The ability of APOG to navigate these risks effectively, manage its cost structure, and capitalize on growth opportunities in the architecture and construction industries will ultimately determine its financial success. Although there is some level of risk involved, the overall outlook is cautiously optimistic, contingent on the company's ability to navigate market dynamics and effectively implement its strategic plans.
```
Rating | Short-Term | Long-Term Senior |
---|---|---|
Outlook | Baa2 | B2 |
Income Statement | Baa2 | Baa2 |
Balance Sheet | Ba2 | Ba3 |
Leverage Ratios | Ba1 | B1 |
Cash Flow | B2 | C |
Rates of Return and Profitability | Baa2 | C |
*Financial analysis is the process of evaluating a company's financial performance and position by neural network. It involves reviewing the company's financial statements, including the balance sheet, income statement, and cash flow statement, as well as other financial reports and documents.
How does neural network examine financial reports and understand financial state of the company?
References
- G. Theocharous and A. Hallak. Lifetime value marketing using reinforcement learning. RLDM 2013, page 19, 2013
- Athey S, Imbens GW. 2017a. The econometrics of randomized experiments. In Handbook of Economic Field Experiments, Vol. 1, ed. E Duflo, A Banerjee, pp. 73–140. Amsterdam: Elsevier
- Imai K, Ratkovic M. 2013. Estimating treatment effect heterogeneity in randomized program evaluation. Ann. Appl. Stat. 7:443–70
- T. Morimura, M. Sugiyama, M. Kashima, H. Hachiya, and T. Tanaka. Nonparametric return distribution ap- proximation for reinforcement learning. In Proceedings of the 27th International Conference on Machine Learning, pages 799–806, 2010
- D. S. Bernstein, S. Zilberstein, and N. Immerman. The complexity of decentralized control of Markov Decision Processes. In UAI '00: Proceedings of the 16th Conference in Uncertainty in Artificial Intelligence, Stanford University, Stanford, California, USA, June 30 - July 3, 2000, pages 32–37, 2000.
- Chow, G. C. (1960), "Tests of equality between sets of coefficients in two linear regressions," Econometrica, 28, 591–605.
- Armstrong, J. S. M. C. Grohman (1972), "A comparative study of methods for long-range market forecasting," Management Science, 19, 211–221.