AUC Score :
Short-term Tactic1 :
Dominant Strategy :
Time series to forecast n:
ML Model Testing : Modular Neural Network (Speculative Sentiment Analysis)
Hypothesis Testing : Pearson Correlation
Surveillance : Major exchange and OTC
1Short-term revised.
2Time series is updated based on short-term trends.
Key Points
The VN30 index is expected to exhibit moderate volatility in the near term, driven by fluctuating global economic sentiment and domestic investor confidence. Predictions suggest a consolidation phase with potential for a gradual upward trend, contingent upon positive developments in key sectors like banking and real estate. Risks include heightened sensitivity to global market corrections, rising inflation impacting corporate earnings, and the potential for regulatory changes to negatively affect investor sentiment and trigger significant sell-offs. Further risks are associated with unforeseen geopolitical events and the pace of economic recovery, which could lead to sharper declines than anticipated, alongside a significant decline in market liquidity.About VN 30 Index
The VN30 Index is a capitalization-weighted index representing the performance of the 30 largest and most actively traded companies listed on the Ho Chi Minh Stock Exchange (HOSE) in Vietnam. These companies are selected based on free-float market capitalization, trading liquidity, and other qualitative factors, providing a benchmark for the overall performance of the Vietnamese equity market's most significant participants. The VN30 is frequently used as a benchmark for Vietnamese investment funds and a basis for derivatives products such as futures contracts, thus playing a crucial role in the country's financial landscape. It's considered a leading indicator of market sentiment and economic health.
Regularly reviewed and rebalanced, the VN30 ensures that its constituents reflect the evolving dynamics of the Vietnamese economy and market. The index's composition is subject to changes, typically occurring quarterly, based on predefined criteria related to liquidity, market capitalization, and free-float ratios. Investors and analysts closely monitor these revisions, as they can signal shifts in the market's focus and influence investment strategies. Its performance serves as a key indicator for international investors assessing investment opportunities within Vietnam.
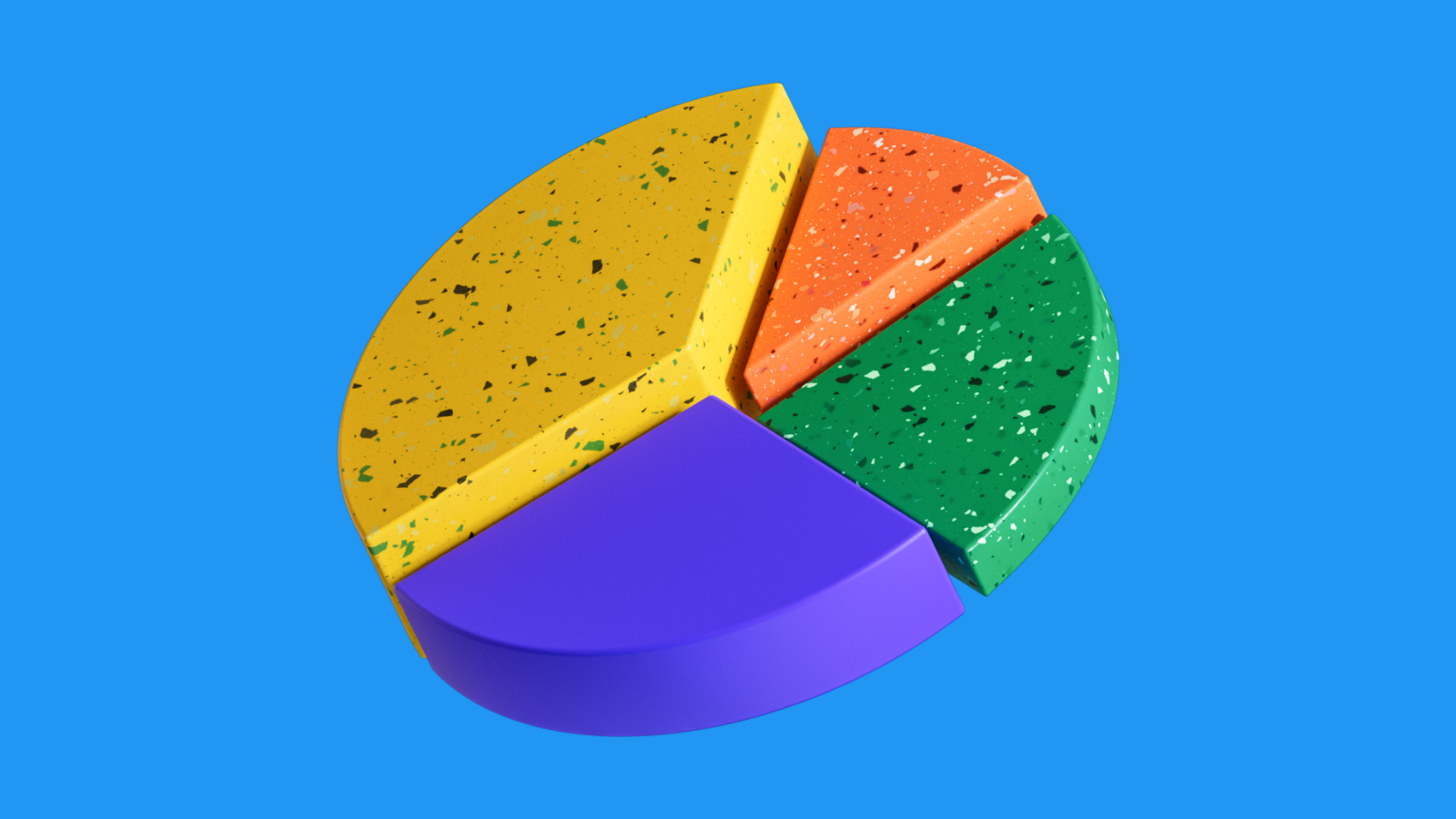
VN30 Index Forecasting Model
Our team proposes a machine learning model for forecasting the VN30 index, a crucial benchmark reflecting the performance of the largest and most liquid stocks on the Ho Chi Minh City Stock Exchange. The model will leverage a comprehensive dataset encompassing both technical indicators and fundamental economic factors. Technical indicators will include moving averages, Relative Strength Index (RSI), Moving Average Convergence Divergence (MACD), and trading volume, offering insights into market trends and momentum. Fundamental economic data will incorporate key macroeconomic indicators relevant to the Vietnamese economy, such as Gross Domestic Product (GDP) growth rate, inflation rate, interest rates, exchange rates, and industrial production. Data will be sourced from reputable financial data providers and governmental statistical agencies, ensuring data integrity and reliability. Feature engineering will be applied to create lagged variables and derive additional insights from the raw data. The model will be trained using historical data, with the timeframe and the specific configuration to be determined and refined through rigorous validation and cross-validation. The dataset will be split into training, validation, and testing sets to ensure the model's generalizability and robustness.
We will employ a hybrid modeling approach to enhance forecast accuracy and capture the complex interplay of market dynamics. The core of our model will be a Recurrent Neural Network (RNN) architecture, specifically Long Short-Term Memory (LSTM) networks, due to their ability to effectively handle sequential data and capture temporal dependencies inherent in financial time series. LSTM networks excel at remembering patterns over extended periods, enabling them to learn from historical trends and market cycles. To further improve the model's predictive power, we will incorporate ensemble methods, such as stacking or blending, to combine the outputs of multiple base learners. This allows us to leverage the strengths of different algorithms. For instance, we might combine the LSTM's time-series forecasting abilities with the predictive power of a gradient boosting regressor, or other statistical method. The model's performance will be evaluated using a combination of metrics, including Mean Squared Error (MSE), Root Mean Squared Error (RMSE), and Mean Absolute Error (MAE), to measure prediction accuracy. Moreover, we will assess the model's profitability through backtesting on historical data, including transaction costs and other real-world considerations.
Model validation and refinement will be an iterative process. Regular model retraining, incorporating the latest available data, is crucial for maintaining performance. We will employ a rolling window approach, where the training set is shifted forward in time. Sensitivity analysis will be conducted to understand how changes in the model's parameters, input variables, or market conditions impact forecasts. We will conduct a thorough investigation into the model's limitations and potential biases. Furthermore, the model will be continuously monitored for anomalies or performance degradation, which would trigger further investigation and refinement. Risk management strategies, such as stop-loss orders, should always be implemented alongside the model's output. The team will create a visualization and communication plan to ensure the model's outputs are clearly interpreted by stakeholders, enabling informed decision-making in investment and risk management activities.
ML Model Testing
n:Time series to forecast
p:Price signals of VN 30 index
j:Nash equilibria (Neural Network)
k:Dominated move of VN 30 index holders
a:Best response for VN 30 target price
For further technical information as per how our model work we invite you to visit the article below:
How do KappaSignal algorithms actually work?
VN 30 Index Forecast Strategic Interaction Table
Strategic Interaction Table Legend:
X axis: *Likelihood% (The higher the percentage value, the more likely the event will occur.)
Y axis: *Potential Impact% (The higher the percentage value, the more likely the price will deviate.)
Z axis (Grey to Black): *Technical Analysis%
VN30 Index: Financial Outlook and Forecast
The VN30 Index, representing the top 30 largest and most liquid companies listed on the Ho Chi Minh Stock Exchange (HOSE) in Vietnam, offers a valuable barometer of the overall health and trajectory of the Vietnamese economy. Its financial outlook is intricately linked to several key drivers, including domestic economic growth, foreign direct investment (FDI) inflows, global economic conditions, and government policies. The Vietnamese economy has demonstrated resilience and sustained growth in recent years, primarily fueled by a robust manufacturing sector, strong domestic consumption, and a growing middle class. FDI continues to play a crucial role, providing capital, technological know-how, and creating employment opportunities. Furthermore, Vietnam's strategic location within Southeast Asia and its trade agreements, such as the Comprehensive and Progressive Agreement for Trans-Pacific Partnership (CPTPP), enhance its attractiveness to both domestic and international investors. The performance of sectors such as banking, real estate, consumer discretionary, and manufacturing will significantly impact the index's future performance. Effective management of inflation, interest rates, and exchange rates will be essential to maintain a favorable economic environment and support corporate profitability.
Several macroeconomic factors will continue to shape the VN30's financial outlook. Global economic trends, particularly those affecting major trading partners such as the United States, China, and the European Union, will exert a significant influence. Increased global demand for Vietnamese goods, along with stability in global supply chains, will contribute positively. The development of infrastructure, including upgrades to transportation networks, will facilitate trade and investment. Prudent fiscal management by the government, including efforts to reduce public debt and enhance transparency, can boost investor confidence. The Vietnamese government's commitment to economic reforms, improving the regulatory environment, and fighting corruption is also crucial. Digitalization and technological advancements, particularly in the financial and e-commerce sectors, hold significant potential for driving growth. Furthermore, the banking sector, a critical component of the VN30, requires continued strengthening, including addressing potential risks related to non-performing loans and capital adequacy.
The future performance of the VN30 Index will also be influenced by company-specific factors. Strong corporate governance practices, including transparency in financial reporting and effective risk management, are essential for attracting and retaining investors. Companies that are adaptable to changing consumer preferences and invest in research and development will be well-positioned to succeed. Technological innovation and adoption of modern business practices can enhance efficiency and competitiveness. Diversification across sectors and geographies can provide resilience during periods of economic volatility. Additionally, successful companies will need to manage environmental, social, and governance (ESG) factors, which are becoming increasingly important to investors globally. Careful assessment of specific industries, particularly the sectors that make up a significant portion of the VN30, is also essential. Ongoing financial performance, earnings growth, and profitability will be key determinants of index movement.
Based on current trends and anticipated developments, the VN30 Index is predicted to exhibit a positive outlook over the medium to long term. The underlying drivers of economic growth, coupled with structural reforms and continued inflows of foreign investment, support a favorable trajectory. However, this prediction carries certain risks. Global economic slowdown or recession could negatively impact export demand and FDI inflows. Rising inflation could erode corporate profits and consumer spending. Geopolitical instability could disrupt supply chains and create market volatility. Domestic challenges, such as regulatory hurdles, infrastructure bottlenecks, and skill gaps, could also hinder progress. Prudent risk management, proactive policy responses, and effective monitoring of macroeconomic indicators will be necessary to navigate potential challenges and ensure sustained positive performance for the VN30 Index.
Rating | Short-Term | Long-Term Senior |
---|---|---|
Outlook | B2 | Ba3 |
Income Statement | Caa2 | Baa2 |
Balance Sheet | Ba3 | Baa2 |
Leverage Ratios | B3 | Caa2 |
Cash Flow | B3 | C |
Rates of Return and Profitability | B2 | B1 |
*An aggregate rating for an index summarizes the overall sentiment towards the companies it includes. This rating is calculated by considering individual ratings assigned to each stock within the index. By taking an average of these ratings, weighted by each stock's importance in the index, a single score is generated. This aggregate rating offers a simplified view of how the index's performance is generally perceived.
How does neural network examine financial reports and understand financial state of the company?
References
- Breiman L. 2001b. Statistical modeling: the two cultures (with comments and a rejoinder by the author). Stat. Sci. 16:199–231
- M. Benaim, J. Hofbauer, and S. Sorin. Stochastic approximations and differential inclusions, Part II: Appli- cations. Mathematics of Operations Research, 31(4):673–695, 2006
- Cheung, Y. M.D. Chinn (1997), "Further investigation of the uncertain unit root in GNP," Journal of Business and Economic Statistics, 15, 68–73.
- R. Rockafellar and S. Uryasev. Optimization of conditional value-at-risk. Journal of Risk, 2:21–42, 2000.
- Candès E, Tao T. 2007. The Dantzig selector: statistical estimation when p is much larger than n. Ann. Stat. 35:2313–51
- Pennington J, Socher R, Manning CD. 2014. GloVe: global vectors for word representation. In Proceedings of the 2014 Conference on Empirical Methods on Natural Language Processing, pp. 1532–43. New York: Assoc. Comput. Linguist.
- M. Petrik and D. Subramanian. An approximate solution method for large risk-averse Markov decision processes. In Proceedings of the 28th International Conference on Uncertainty in Artificial Intelligence, 2012.