AUC Score :
Short-term Tactic1 :
Dominant Strategy :
Time series to forecast n:
ML Model Testing : Modular Neural Network (Market Volatility Analysis)
Hypothesis Testing : Polynomial Regression
Surveillance : Major exchange and OTC
1Short-term revised.
2Time series is updated based on short-term trends.
Key Points
The TR/CC CRB ex Energy ER index is anticipated to exhibit moderate volatility, with a potential for modest gains driven by increased demand in industrial commodities and recovering global economic activity, particularly in developing markets. However, this outlook is tempered by several risks. A potential slowdown in major economies, rising interest rates, and persistent inflationary pressures could negatively impact commodity demand and pricing, leading to corrections. Furthermore, geopolitical instability, particularly in regions crucial for commodity production or transit, poses a significant threat to supply chains and could trigger price volatility. Increased regulatory scrutiny on commodity trading and environmental concerns, such as the transition to renewable energy, could also exert downward pressure on fossil fuel-related components of the index.About TR/CC CRB ex Energy ER Index
The Thomson Reuters/CoreCommodity CRB (TR/CC CRB) ex Energy ER Index is a benchmark that tracks the price movements of a diverse range of commodities. This index, excluding energy components, provides investors with a broader perspective on commodity market performance, focusing on agricultural products, precious metals, industrial metals, and livestock. It serves as a tool for understanding inflation trends, assessing the performance of non-energy commodity sectors, and evaluating the diversification benefits of commodity investments.
As an Excess Return (ER) index, the TR/CC CRB ex Energy ER Index reflects the returns an investor would receive by holding a portfolio of commodity futures contracts, reinvesting the collateral, and accounting for rolling the contracts. The index's methodology incorporates rules for selecting and weighting the commodities, typically based on liquidity and global economic significance. This structure enables the index to reflect fluctuations in the price of commodities and to allow investors to diversify their investment portfolios beyond energy.
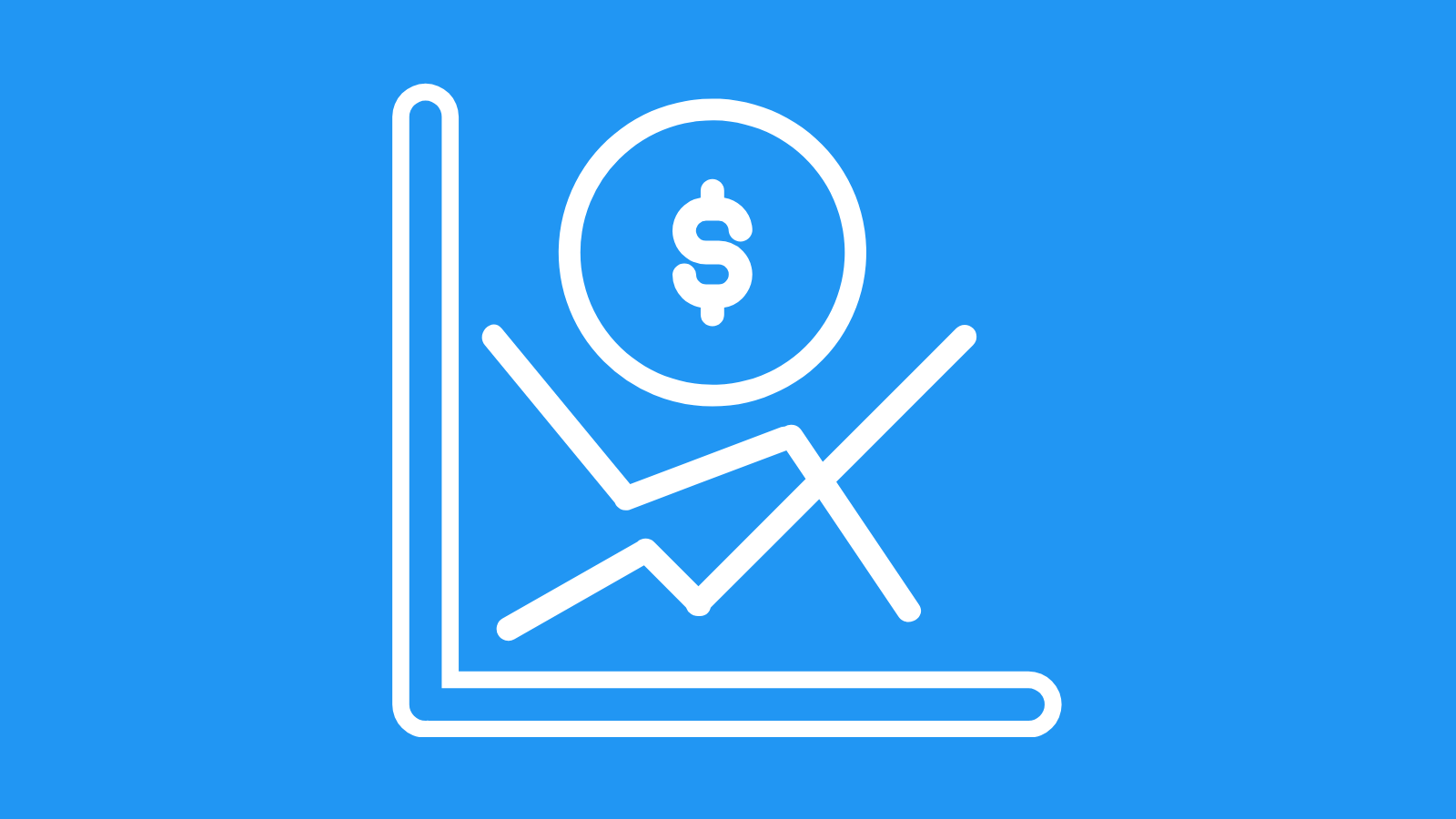
TR/CC CRB ex Energy ER Index Forecast Model
Our approach to forecasting the TR/CC CRB ex Energy ER index leverages a combination of time series analysis and machine learning techniques. Given the inherent volatility and multi-faceted influences on this commodity-focused index, we employ a hybrid model to capture both short-term fluctuations and long-term trends. Initially, we conduct extensive data preprocessing, including cleaning and handling missing values within the historical dataset, and then employ feature engineering to extract relevant information from our existing data set. Specifically, we consider economic indicators such as global GDP growth, inflation rates (e.g., CPI), and industrial production indices, along with financial indicators, like interest rates, exchange rates, and stock market performance. We also integrate sector-specific data pertaining to agriculture, metals, and other commodity-producing industries, to provide a deeper context for the model.
Our core model architecture incorporates a stacked ensemble approach. We use a variety of individual models. Initially, a recurrent neural network (RNN), such as a Long Short-Term Memory (LSTM) or a Gated Recurrent Unit (GRU), is used. They will be employed to capture temporal dependencies. Furthermore, we employ a Gradient Boosting Machine (GBM) and a Random Forest model to capture non-linear relationships within the broader feature space. The outputs of these individual models are then fed into a meta-learner, such as a stacked generalized linear model. This layer learns how to weight and combine the predictions from the base models, which results in the final forecast. We leverage cross-validation techniques to optimize hyperparameter settings, specifically utilizing walk-forward validation to train and evaluate the model's performance. Model validation will be done with robust statistical metrics, such as Mean Absolute Error (MAE), Root Mean Squared Error (RMSE), and Mean Absolute Percentage Error (MAPE), to assess predictive accuracy.
To ensure model robustness and adaptation to changing market conditions, we implement continuous monitoring and model retraining protocols. We will utilize a rolling window methodology to retrain the model at regular intervals, incorporating the most recent data. This helps to mitigate the risk of model degradation over time, as it is able to adapt to evolving market dynamics. Moreover, regular model performance reviews are conducted, comparing forecast results with the actual index values. This iterative process enables us to identify potential biases, adjust model parameters, and improve the model's accuracy and reliability. Finally, we evaluate the model's performance with consideration of potential external events and economic trends to ensure its robustness and predictive power under various market circumstances.
ML Model Testing
n:Time series to forecast
p:Price signals of TR/CC CRB ex Energy ER index
j:Nash equilibria (Neural Network)
k:Dominated move of TR/CC CRB ex Energy ER index holders
a:Best response for TR/CC CRB ex Energy ER target price
For further technical information as per how our model work we invite you to visit the article below:
How do KappaSignal algorithms actually work?
TR/CC CRB ex Energy ER Index Forecast Strategic Interaction Table
Strategic Interaction Table Legend:
X axis: *Likelihood% (The higher the percentage value, the more likely the event will occur.)
Y axis: *Potential Impact% (The higher the percentage value, the more likely the price will deviate.)
Z axis (Grey to Black): *Technical Analysis%
TR/CC CRB ex Energy ER Index: Financial Outlook and Forecast
The Thomson Reuters/CoreCommodity CRB ex Energy Excess Return (ER) Index is designed to track the performance of a broad basket of commodities, excluding the highly volatile energy sector. This index provides investors with exposure to a diverse range of raw materials, including agricultural products, industrial metals, and precious metals. The "Excess Return" component reflects the performance of the underlying commodity futures contracts after accounting for the return of the collateral invested in the underlying assets. The ER structure means the index benefits from the reinvestment of collateral income, potentially enhancing overall returns compared to a simple price return index. This index serves as a barometer of commodity market sentiment, particularly reflecting global manufacturing and consumer demand, with inflation pressures being another significant influence. Understanding the dynamics of this index is crucial for investors seeking diversification and hedging strategies within the broader financial markets.
The financial outlook for the TR/CC CRB ex Energy ER Index is significantly influenced by several interconnected factors. Firstly, the health of the global economy, particularly in emerging markets like China and India, plays a pivotal role. Strong economic growth typically fuels demand for industrial metals and agricultural commodities. Secondly, supply-side disruptions, such as adverse weather patterns affecting agricultural yields, or geopolitical events impacting mining operations, can lead to price increases. Furthermore, the strength of the US dollar has an inverse relationship with commodity prices; a weaker dollar generally supports higher commodity valuations. Finally, changes in investor sentiment, driven by shifts in risk appetite, inflationary expectations, and interest rate policies, can significantly impact the index's performance. Examining these multifaceted variables is key to assessing the future trajectory of the index.
Analyzing the current macroeconomic landscape suggests a somewhat mixed outlook. Positive signals come from ongoing infrastructure spending globally and the potential for increased consumer demand as economies recover from the impact of previous global events. These factors may support higher prices for industrial metals like copper and aluminum and for agricultural products. Conversely, concerns regarding inflationary pressures and potential interest rate hikes by central banks could weigh on commodity prices. The expectation of a continued strong dollar could limit gains, as commodities become more expensive for buyers holding other currencies. Also, the global supply chain pressures and geopolitical risks may create volatility in the market. Therefore, a balanced assessment is critical to understanding the outlook of the index.
The forecast for the TR/CC CRB ex Energy ER Index in the near to mid-term is cautiously optimistic. The expectation is for moderate gains, driven by sustained, albeit slowing, global economic growth and supply-side constraints in certain commodity markets. The index's performance will be subject to volatility due to unexpected events, and supply chain pressures, but the underlying demand will remain. Key risks include a sharper-than-anticipated economic slowdown in major economies, a more aggressive tightening of monetary policy by central banks, and further escalations of geopolitical tensions, all of which could negatively impact the index. Additionally, the index can face significant volatility due to its sensitivity to external macroeconomic factors, specifically inflation rates, which can create unpredictability in the future for this index.
Rating | Short-Term | Long-Term Senior |
---|---|---|
Outlook | Ba3 | B2 |
Income Statement | B3 | Baa2 |
Balance Sheet | Baa2 | Caa2 |
Leverage Ratios | B2 | B2 |
Cash Flow | Baa2 | Caa2 |
Rates of Return and Profitability | Ba2 | Caa2 |
*An aggregate rating for an index summarizes the overall sentiment towards the companies it includes. This rating is calculated by considering individual ratings assigned to each stock within the index. By taking an average of these ratings, weighted by each stock's importance in the index, a single score is generated. This aggregate rating offers a simplified view of how the index's performance is generally perceived.
How does neural network examine financial reports and understand financial state of the company?
References
- E. Altman, K. Avrachenkov, and R. N ́u ̃nez-Queija. Perturbation analysis for denumerable Markov chains with application to queueing models. Advances in Applied Probability, pages 839–853, 2004
- F. A. Oliehoek and C. Amato. A Concise Introduction to Decentralized POMDPs. SpringerBriefs in Intelligent Systems. Springer, 2016
- S. Devlin, L. Yliniemi, D. Kudenko, and K. Tumer. Potential-based difference rewards for multiagent reinforcement learning. In Proceedings of the Thirteenth International Joint Conference on Autonomous Agents and Multiagent Systems, May 2014
- K. Tuyls and G. Weiss. Multiagent learning: Basics, challenges, and prospects. AI Magazine, 33(3): 41–52, 2012
- Wan M, Wang D, Goldman M, Taddy M, Rao J, et al. 2017. Modeling consumer preferences and price sensitiv- ities from large-scale grocery shopping transaction logs. In Proceedings of the 26th International Conference on the World Wide Web, pp. 1103–12. New York: ACM
- V. Borkar. Stochastic approximation: a dynamical systems viewpoint. Cambridge University Press, 2008
- Lai TL, Robbins H. 1985. Asymptotically efficient adaptive allocation rules. Adv. Appl. Math. 6:4–22