AUC Score :
Short-term Tactic1 :
Dominant Strategy :
Time series to forecast n:
ML Model Testing : Supervised Machine Learning (ML)
Hypothesis Testing : Statistical Hypothesis Testing
Surveillance : Major exchange and OTC
1Short-term revised.
2Time series is updated based on short-term trends.
Key Points
The Dow Jones U.S. Technology Index is anticipated to exhibit moderate growth, fueled by continued advancements in artificial intelligence, cloud computing, and cybersecurity sectors. However, this positive outlook faces significant risks, including potential regulatory scrutiny impacting major tech companies and the possibility of increased interest rates affecting valuation multiples. Furthermore, geopolitical instability and macroeconomic uncertainties, such as inflation and recessionary pressures, could trigger market volatility and dampen investor sentiment, resulting in slowed or stagnant growth.About Dow Jones U.S. Technology Index
The Dow Jones U.S. Technology Index represents a diverse group of companies within the technology sector, reflecting the performance of leading businesses involved in software, hardware, semiconductors, internet services, and related fields. This index is designed to provide a benchmark for investors seeking exposure to the rapidly evolving technology industry. It offers a broad overview of the sector's health and growth potential, encompassing companies of varying sizes and specializations.
The composition of the Dow Jones U.S. Technology Index is subject to periodic review and adjustments. The index is market capitalization weighted, meaning that companies with larger market values have a greater impact on the index's overall performance. This approach aligns the index's movements closely with overall market trends and allows investors to track the evolving landscape of technological innovation within the United States.
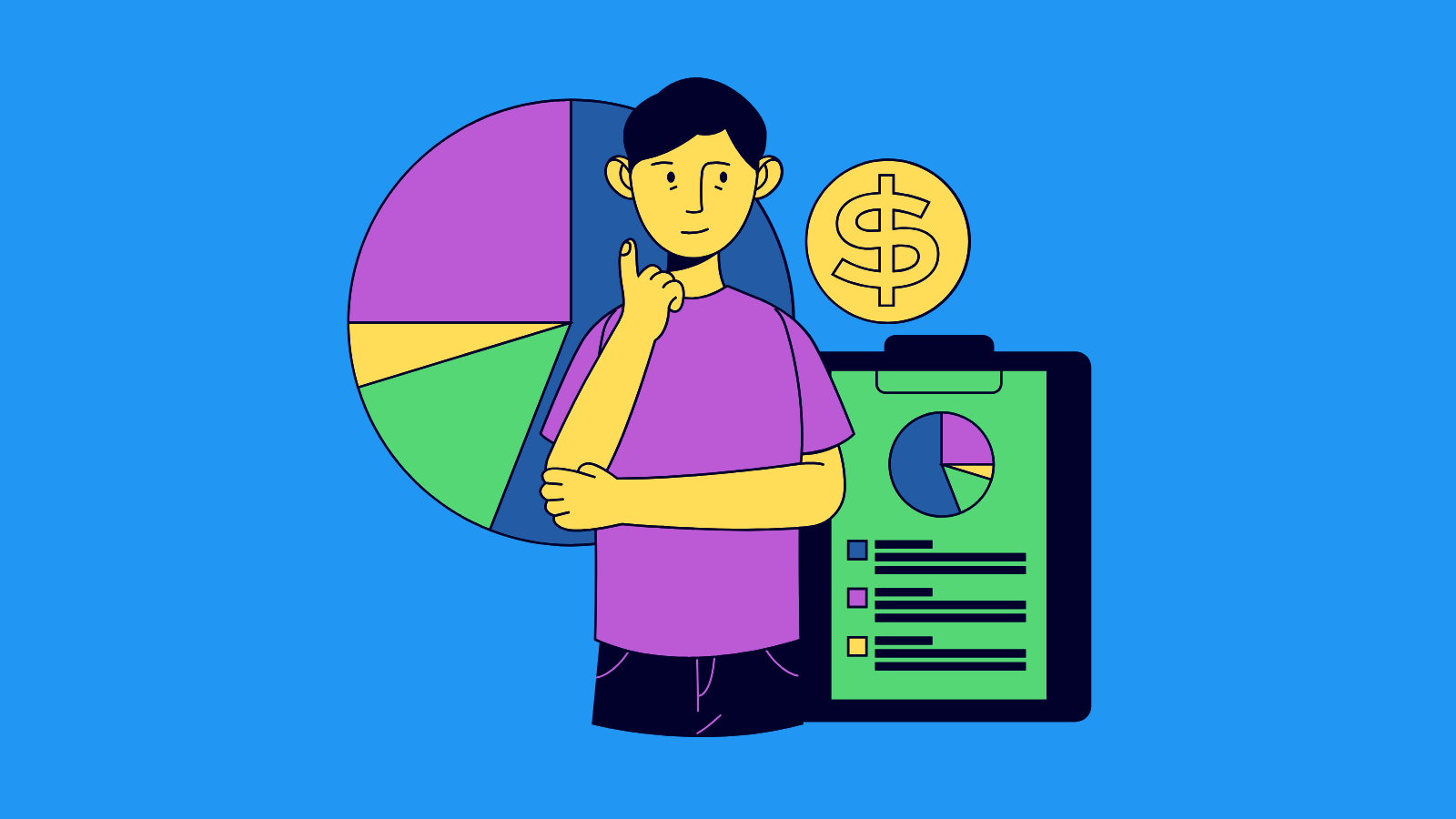
Dow Jones U.S. Technology Index Forecast Model
Our team of data scientists and economists proposes a robust machine learning model for forecasting the Dow Jones U.S. Technology Index. The model will employ a hybrid approach, leveraging the strengths of both time series analysis and advanced machine learning techniques. We will begin by gathering a comprehensive dataset encompassing both internal and external factors. Internally, we will collect historical index values, trading volumes, and volatility measures. Externally, we will incorporate macroeconomic indicators such as GDP growth, inflation rates, interest rates, and unemployment figures. Furthermore, we will integrate sentiment analysis from news articles, social media feeds, and financial reports to capture market sentiment and investor behavior. The data will be cleaned, preprocessed, and feature engineered to optimize model performance. This includes handling missing values, scaling features, and creating lagged variables to capture temporal dependencies.
The core of our model will consist of a combination of algorithms. We intend to use a Recurrent Neural Network (RNN), specifically a Long Short-Term Memory (LSTM) network, for its ability to handle sequential data and capture complex patterns within the time series. To enhance the model's predictive power, we will incorporate an ensemble method by combining the LSTM with a Gradient Boosting Machine (GBM) to address the complexities of economic factors. The LSTM will primarily focus on capturing the intricate patterns from financial time series data, while the GBM will focus on the economic data. We will optimize the model's hyperparameters using techniques such as cross-validation and grid search, evaluating performance using metrics such as Mean Squared Error (MSE), Root Mean Squared Error (RMSE), and Mean Absolute Percentage Error (MAPE). Rigorous backtesting will be conducted on historical data, using various time horizons to assess the model's accuracy and robustness in different market conditions.
The final model will provide forecasts with confidence intervals, allowing for an understanding of the potential range of index values. We will also develop a user-friendly interface for visualizing the forecasts and assessing the impact of different input variables. The model's output will provide valuable insights for investment decisions, risk management, and portfolio optimization. The model will be regularly updated and refined with new data and algorithmic improvements to maintain its accuracy and relevance over time. Finally, we acknowledge that financial markets are inherently volatile, and the forecasts are inherently uncertain; therefore, we will include the model's limitations. We will emphasize the significance of incorporating the model results within a comprehensive investment strategy that considers the potential risk.
ML Model Testing
n:Time series to forecast
p:Price signals of Dow Jones U.S. Technology index
j:Nash equilibria (Neural Network)
k:Dominated move of Dow Jones U.S. Technology index holders
a:Best response for Dow Jones U.S. Technology target price
For further technical information as per how our model work we invite you to visit the article below:
How do KappaSignal algorithms actually work?
Dow Jones U.S. Technology Index Forecast Strategic Interaction Table
Strategic Interaction Table Legend:
X axis: *Likelihood% (The higher the percentage value, the more likely the event will occur.)
Y axis: *Potential Impact% (The higher the percentage value, the more likely the price will deviate.)
Z axis (Grey to Black): *Technical Analysis%
Dow Jones U.S. Technology Index: Financial Outlook and Forecast
The Dow Jones U.S. Technology Index, encompassing a diverse range of companies at the forefront of technological advancement, presents a complex financial outlook. The sector's performance is intrinsically linked to several key drivers, including innovation cycles, consumer adoption rates, global economic conditions, and regulatory landscapes. Ongoing advancements in areas such as artificial intelligence (AI), cloud computing, cybersecurity, and the Internet of Things (IoT) are expected to fuel sustained growth. Furthermore, increased spending on digital transformation initiatives across various industries is anticipated to provide significant tailwinds. However, this positive trajectory is counterbalanced by various challenges, including heightened competition, rapid technological obsolescence, and the potential for macroeconomic headwinds such as rising interest rates and inflation to impact consumer spending and business investment. The index's performance will also be influenced by shifts in investor sentiment, which can be volatile, especially in response to unforeseen geopolitical events or changes in market dynamics.
The forecast for the Dow Jones U.S. Technology Index hinges on the continued ability of companies to adapt and innovate. Strong financial performance is contingent on robust revenue growth, margin expansion, and effective capital allocation. Companies that can successfully navigate the evolving technological landscape by investing in research and development, acquiring strategic assets, and building strong competitive advantages are most likely to thrive. Specific sub-sectors, such as semiconductors and software, may experience varying growth rates based on their respective market dynamics and demand patterns. For example, the semiconductor industry, while facing cyclical downturns, remains crucial for advancements in computing and electronics, with potential long-term growth tied to rising demand across diverse sectors. Software companies benefit from a shift to cloud-based solutions, offering subscription services and recurring revenue streams. Understanding and anticipating changes in user demand, regulatory requirements, and emerging technologies will determine the long-term success of these sub-sectors.
Significant risks cloud the outlook for the Dow Jones U.S. Technology Index. Geopolitical tensions, supply chain disruptions, and inflationary pressures could negatively impact the sector's growth trajectory. In particular, global competition, especially from China, will continue to put pressure on profit margins. Increased scrutiny from regulatory bodies regarding data privacy, antitrust practices, and other legal issues poses a constant threat. Furthermore, the industry is heavily reliant on attracting and retaining skilled talent. Labor shortages and rising employee costs could affect operational efficiency and stifle innovation. Cybersecurity threats are an ongoing concern, requiring significant investment in security infrastructure and constant vigilance. External factors, such as shifts in consumer preference or unexpected shifts in the market, also introduce volatility. All these factors are inter-linked and can produce chain reactions that could potentially weaken the market.
The overall outlook for the Dow Jones U.S. Technology Index is cautiously positive. The long-term growth prospects for the technology sector remain favorable due to continuing technological innovation and the increasing reliance on technology across diverse sectors. However, this prediction is coupled with significant risks. The index faces potential headwinds from geopolitical instability, economic uncertainty, and regulatory challenges. Increased competition, rapid technological changes, and the vulnerability of the sector to external economic conditions will require continued agility and adaptation from its constituent companies. A recession and major financial setback might lead to drastic shifts in investor behaviour and market valuations. Therefore, although the long-term outlook is generally favorable, investors should exercise careful risk management and monitor market dynamics to mitigate potential downside risks.
Rating | Short-Term | Long-Term Senior |
---|---|---|
Outlook | B3 | Ba3 |
Income Statement | C | Baa2 |
Balance Sheet | Caa2 | B2 |
Leverage Ratios | Caa2 | B2 |
Cash Flow | Baa2 | B2 |
Rates of Return and Profitability | C | Ba1 |
*An aggregate rating for an index summarizes the overall sentiment towards the companies it includes. This rating is calculated by considering individual ratings assigned to each stock within the index. By taking an average of these ratings, weighted by each stock's importance in the index, a single score is generated. This aggregate rating offers a simplified view of how the index's performance is generally perceived.
How does neural network examine financial reports and understand financial state of the company?
References
- Matzkin RL. 2007. Nonparametric identification. In Handbook of Econometrics, Vol. 6B, ed. J Heckman, E Learner, pp. 5307–68. Amsterdam: Elsevier
- Jorgenson, D.W., Weitzman, M.L., ZXhang, Y.X., Haxo, Y.M. and Mat, Y.X., 2023. Can Neural Networks Predict Stock Market?. AC Investment Research Journal, 220(44).
- C. Wu and Y. Lin. Minimizing risk models in Markov decision processes with policies depending on target values. Journal of Mathematical Analysis and Applications, 231(1):47–67, 1999
- Van der Vaart AW. 2000. Asymptotic Statistics. Cambridge, UK: Cambridge Univ. Press
- Barrett, C. B. (1997), "Heteroscedastic price forecasting for food security management in developing countries," Oxford Development Studies, 25, 225–236.
- T. Shardlow and A. Stuart. A perturbation theory for ergodic Markov chains and application to numerical approximations. SIAM journal on numerical analysis, 37(4):1120–1137, 2000
- Bai J, Ng S. 2002. Determining the number of factors in approximate factor models. Econometrica 70:191–221