AUC Score :
Short-term Tactic1 :
Dominant Strategy :
Time series to forecast n:
ML Model Testing : Transfer Learning (ML)
Hypothesis Testing : Linear Regression
Surveillance : Major exchange and OTC
1Short-term revised.
2Time series is updated based on short-term trends.
Key Points
The TR/CC CRB Sugar index is projected to experience moderate volatility. This suggests the potential for both gains and losses over the short to medium term. A likely scenario involves price fluctuations driven by evolving supply chain dynamics and unexpected shifts in global demand influenced by climatic events. Risks include unexpected weather patterns impacting key sugar-producing regions, leading to supply disruptions, or changes in government policies affecting trade or subsidies. Furthermore, geopolitical instability in major trading partners could also negatively impact sugar prices.About TR/CC CRB Sugar Index
The Thomson Reuters/CoreCommodity CRB (TR/CC CRB) Index, formerly known as the Commodity Research Bureau Index, is a benchmark designed to reflect the overall price movements of a basket of globally significant commodities. It is widely recognized as a leading indicator of inflation and economic activity, providing insights into supply and demand dynamics across various sectors. The index encompasses a diverse range of commodities, including energy products, agricultural goods, precious metals, and industrial raw materials. This broad scope allows the TR/CC CRB to offer a comprehensive perspective on commodity market trends.
The TR/CC CRB Index is constructed using a weighted methodology, with each commodity assigned a specific weight based on its economic significance and liquidity. These weights are periodically reviewed and adjusted to ensure the index accurately represents the current market landscape. Investors and financial analysts frequently use the TR/CC CRB as a reference point for gauging commodity market performance, constructing investment strategies, and assessing the impact of commodity price fluctuations on broader financial markets and economic conditions.
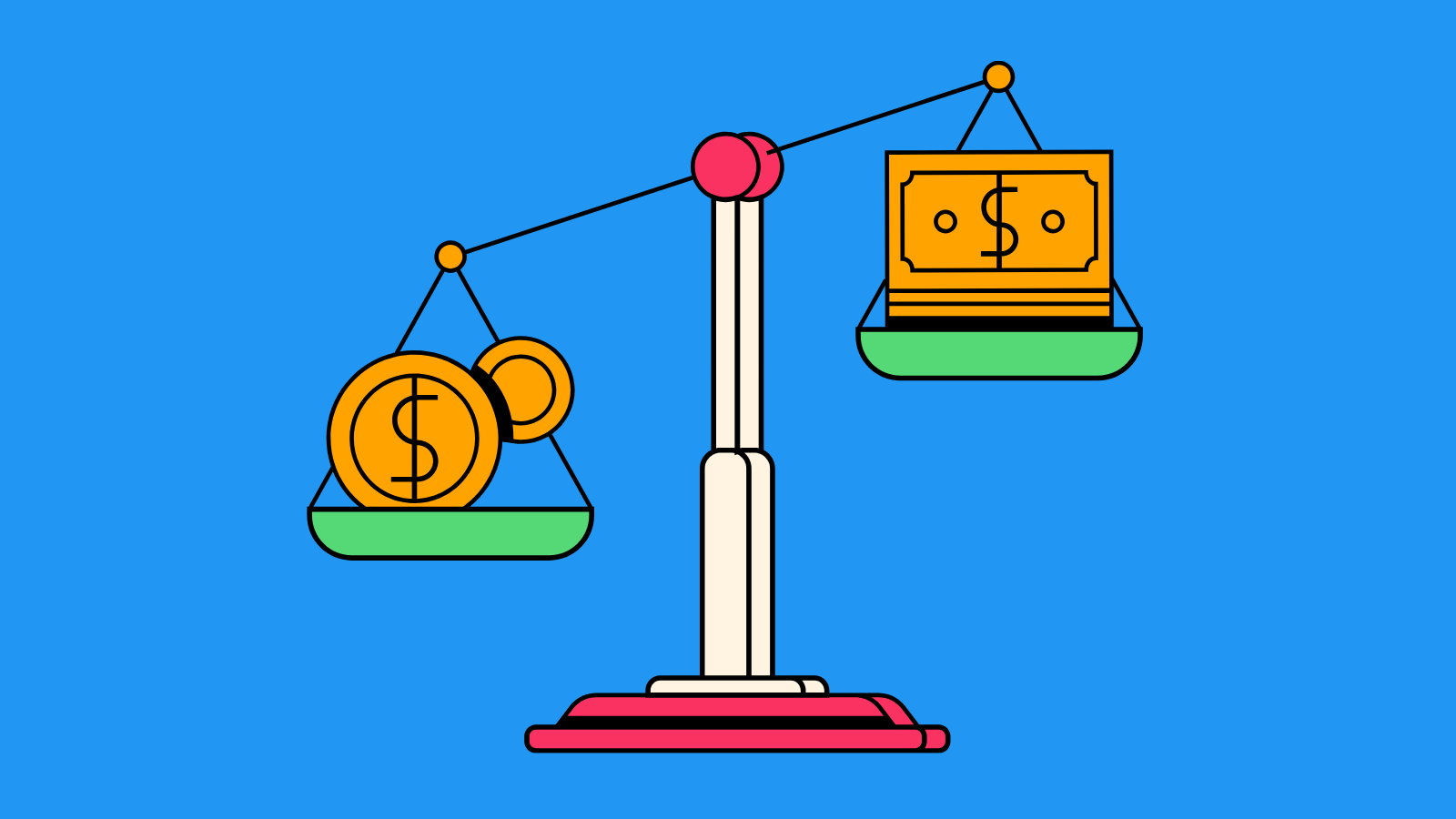
Machine Learning Model for TR/CC CRB Sugar Index Forecast
Our team of data scientists and economists has developed a machine learning model designed to forecast the TR/CC CRB Sugar Index. The model leverages a diverse set of predictor variables categorized into fundamental, technical, and macroeconomic factors. Fundamental indicators include global sugar production, consumption, and inventory levels, gleaned from reputable sources such as the USDA and industry reports. Technical indicators incorporated in the model encompass historical price trends, moving averages, volume data, and volatility measures, derived from time-series analysis of the index's past performance. Furthermore, macroeconomic factors are integrated, encompassing exchange rates (particularly of currencies of major sugar-producing and consuming nations), global economic growth indicators (like GDP), and inflation rates to capture the broader economic environment's influence on sugar prices. Data preprocessing involves cleaning, imputing missing values, and scaling the variables for optimal model performance. This preprocessing ensures data consistency and prepares the data for training the machine learning algorithms.
The model's architecture centers around a combination of machine learning techniques chosen for their suitability in time-series forecasting. We employ a hybrid approach that integrates a Long Short-Term Memory (LSTM) network, a type of recurrent neural network (RNN) particularly effective in capturing sequential dependencies inherent in financial time series, with an ensemble method, specifically a Gradient Boosting Regressor. The LSTM component excels at modeling complex, non-linear relationships and capturing long-term trends, while the Gradient Boosting Regressor handles potentially noisy or less predictable components of the sugar index's fluctuations and enhances the model's overall predictive power by combining multiple weaker models. The model is trained on a comprehensive historical dataset spanning several decades, using cross-validation techniques to ensure robustness and generalization to unseen data. The data is split into training, validation, and testing sets, enabling rigorous evaluation of the model's performance.
Model evaluation is conducted using a suite of metrics, including Mean Absolute Error (MAE), Root Mean Squared Error (RMSE), and the R-squared coefficient, to assess the forecast accuracy. We also utilize backtesting on historical data to simulate real-world trading scenarios and validate the model's performance in different market conditions. The model output provides a point forecast of the TR/CC CRB Sugar Index, along with a confidence interval to quantify the uncertainty associated with the prediction. Regular monitoring and retraining of the model are essential, incorporating the most recent data and periodically updating the model with new information. The model will be continuously refined to incorporate changing market dynamics and improve predictive accuracy. This iterative approach and continuous monitoring will ensure that the model remains a valuable tool for understanding and anticipating sugar price movements.
ML Model Testing
n:Time series to forecast
p:Price signals of TR/CC CRB Sugar index
j:Nash equilibria (Neural Network)
k:Dominated move of TR/CC CRB Sugar index holders
a:Best response for TR/CC CRB Sugar target price
For further technical information as per how our model work we invite you to visit the article below:
How do KappaSignal algorithms actually work?
TR/CC CRB Sugar Index Forecast Strategic Interaction Table
Strategic Interaction Table Legend:
X axis: *Likelihood% (The higher the percentage value, the more likely the event will occur.)
Y axis: *Potential Impact% (The higher the percentage value, the more likely the price will deviate.)
Z axis (Grey to Black): *Technical Analysis%
TR/CC CRB Sugar Index: Financial Outlook and Forecast
The TR/CC CRB Sugar Index, a benchmark reflecting the price movements of raw sugar futures contracts traded on the Intercontinental Exchange (ICE), is subject to a complex interplay of global supply and demand dynamics. The financial outlook for this index hinges on several critical factors. Production levels in key sugar-producing regions, particularly Brazil, India, and Thailand, hold significant sway. Weather patterns, including droughts, floods, and temperature fluctuations, directly impact sugarcane yields and processing efficiency. Furthermore, government policies such as export subsidies, import tariffs, and biofuel mandates significantly influence the overall supply landscape. Demand for sugar is primarily driven by consumption in food and beverage industries, with global population growth and evolving dietary preferences playing a key role. Economic conditions in major sugar-consuming countries, particularly emerging markets, also influence demand patterns.
Forecasting the future performance of the TR/CC CRB Sugar Index requires a thorough assessment of the forces shaping supply and demand. On the supply side, Brazilian sugarcane production is particularly crucial, as it accounts for a large percentage of global sugar exports. A favorable climate and efficient harvesting could lead to a surge in supply, potentially exerting downward pressure on the index. Conversely, adverse weather conditions, such as the El NiƱo phenomenon, could damage crops, leading to production shortfalls and upward price pressure. The Indian monsoon season and the subsequent cane harvest are other important factors. On the demand side, the growth in the global population and rising incomes in emerging economies, especially in Asia, are anticipated to drive sugar consumption higher, supporting the index. Meanwhile, health-conscious consumers and the ongoing debate on sugar consumption may moderate this effect. The growth in the biofuel sector could also impact the sugar market.
Several economic and geopolitical developments may potentially affect the sugar market. Changes in energy prices, particularly for crude oil, can influence the profitability of sugarcane-based ethanol production, which can, in turn, shift the allocation of sugarcane between sugar and ethanol production. International trade policies and any tariff or quota changes could impact sugar flows and prices. Geopolitical instability in major sugar-producing regions or disruptions to shipping routes could also introduce supply chain risks, leading to price volatility. Currency fluctuations can also influence the competitiveness of sugar exports and imports, affecting the market equilibrium. Furthermore, the evolving regulatory landscape, concerning sugar labeling, consumer protection, and sustainability practices, could influence consumer behavior and the demand for sugar, thus indirectly impacting the index.
The overall outlook for the TR/CC CRB Sugar Index appears moderately positive. Assuming stable weather patterns in major sugar-producing regions and continued global economic growth, the index is projected to experience a gradual upward trend over the next few years. Supportive factors include the increasing consumption in emerging markets and the potential for increased demand due to biofuel production. However, this prediction faces significant risks. Extreme weather events, unexpected changes in government policies, and global economic downturns could trigger significant price volatility or even a decline. The emergence of new sugar substitutes or significant changes in consumer dietary habits also pose a threat. Investors in this index should remain vigilant to monitor the impact of global events, weather patterns and production forecasts, to adjust their portfolios and manage potential risks effectively.
Rating | Short-Term | Long-Term Senior |
---|---|---|
Outlook | Baa2 | B2 |
Income Statement | Ba3 | C |
Balance Sheet | Baa2 | C |
Leverage Ratios | B1 | C |
Cash Flow | Baa2 | Baa2 |
Rates of Return and Profitability | Baa2 | Ba2 |
*An aggregate rating for an index summarizes the overall sentiment towards the companies it includes. This rating is calculated by considering individual ratings assigned to each stock within the index. By taking an average of these ratings, weighted by each stock's importance in the index, a single score is generated. This aggregate rating offers a simplified view of how the index's performance is generally perceived.
How does neural network examine financial reports and understand financial state of the company?
References
- Akgiray, V. (1989), "Conditional heteroscedasticity in time series of stock returns: Evidence and forecasts," Journal of Business, 62, 55–80.
- Hastie T, Tibshirani R, Friedman J. 2009. The Elements of Statistical Learning. Berlin: Springer
- Christou, C., P. A. V. B. Swamy G. S. Tavlas (1996), "Modelling optimal strategies for the allocation of wealth in multicurrency investments," International Journal of Forecasting, 12, 483–493.
- Jiang N, Li L. 2016. Doubly robust off-policy value evaluation for reinforcement learning. In Proceedings of the 33rd International Conference on Machine Learning, pp. 652–61. La Jolla, CA: Int. Mach. Learn. Soc.
- Byron, R. P. O. Ashenfelter (1995), "Predicting the quality of an unborn grange," Economic Record, 71, 40–53.
- P. Marbach. Simulated-Based Methods for Markov Decision Processes. PhD thesis, Massachusetts Institute of Technology, 1998
- L. Panait and S. Luke. Cooperative multi-agent learning: The state of the art. Autonomous Agents and Multi-Agent Systems, 11(3):387–434, 2005.