AUC Score :
Short-term Tactic1 :
Dominant Strategy :
Time series to forecast n:
ML Model Testing : Modular Neural Network (Market News Sentiment Analysis)
Hypothesis Testing : Spearman Correlation
Surveillance : Major exchange and OTC
1Short-term revised.
2Time series is updated based on short-term trends.
Key Points
Streamline Health Solutions (STRM) faces potential volatility due to its position in the healthcare IT sector. STRM is likely to experience moderate growth driven by increasing demand for its solutions, particularly in revenue cycle management, as healthcare providers seek to improve efficiency and reduce costs. However, the company's dependence on the healthcare industry exposes it to regulatory changes, payer dynamics, and economic cycles, which could adversely impact its financial performance. Competition from larger, established players in the industry presents a substantial risk. Successful expansion and the ability to innovate and adapt to evolving technologies are vital. Another risk lies in the company's ability to secure and retain large contracts and its dependence on a few key clients.About Streamline Health Solutions
Streamline Health Solutions (STRM) is a healthcare information technology company specializing in solutions for revenue cycle management, focusing on improving financial performance and operational efficiency for healthcare providers. The company's offerings are primarily software-based, helping hospitals and healthcare systems manage patient data, streamline administrative processes, and optimize revenue recovery. Streamline Health aims to address the complex challenges healthcare organizations face in navigating the financial intricacies of patient care, including claim denials, revenue leakage, and regulatory compliance.
STRM develops and markets a suite of products designed to integrate with existing healthcare infrastructure. These solutions often include modules for data analytics, charge capture optimization, and workflow automation. The company's solutions are intended to provide actionable insights, helping clients make informed decisions to enhance financial outcomes and improve the patient experience. They primarily focus on improving the financial health of healthcare providers in an evolving and heavily regulated market.
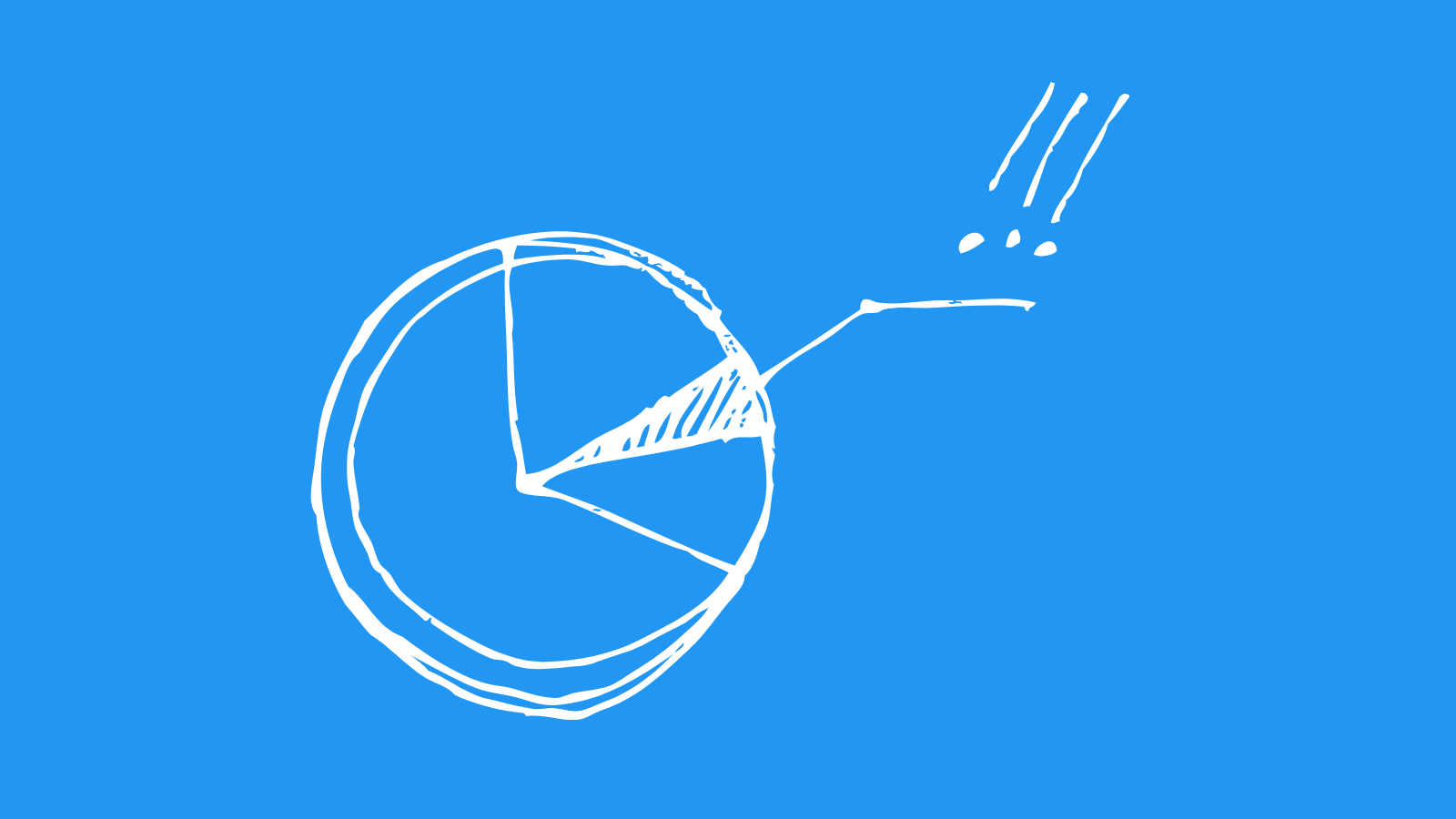
STRM Stock Forecast Model
Our data science and economics team has developed a machine learning model to forecast the performance of Streamline Health Solutions Inc. (STRM) common stock. The model employs a combination of time-series analysis and regression techniques. Crucially, it considers a range of factors, including financial performance metrics such as revenue growth, profitability margins, and debt levels, extracted from Streamline Health's quarterly and annual reports. Additionally, the model incorporates macroeconomic indicators like industry growth rates, healthcare spending trends, and relevant policy changes within the healthcare IT sector. We've integrated sentiment analysis of news articles and social media data, which potentially signals shifts in investor confidence and market perception, to identify patterns and relationships. This allows our model to capture both fundamental business drivers and external market dynamics.
The model architecture is built on a foundation of recurrent neural networks (RNNs), specifically Long Short-Term Memory (LSTM) layers, which are adept at processing sequential data like stock prices and financial time series. These LSTM layers are trained on historical STRM stock data, macroeconomic indicators, and sentiment scores. A regression layer at the end predicts future stock performance indicators. We have implemented several validation techniques, including cross-validation and out-of-sample testing, to rigorously assess the model's performance and minimize overfitting. This iterative process involves comparing the model's forecasts against actual market outcomes, allowing us to refine and improve the model's accuracy over time.
Our initial model outputs a probabilistic forecast, providing a range of potential outcomes rather than a single fixed point. This approach reflects the inherent uncertainty in financial markets. The results generated from the model are intended for informational purposes only and should not be considered as financial advice. Further, we emphasize that the model is dynamic. We are continuously monitoring its performance, updating it with the latest data, and refining its parameters. Regular model review and periodic recalibration are essential. The model's forecasts and their interpretations will be provided to stakeholders, who are encouraged to cross-reference our findings with independent financial analysis.
ML Model Testing
n:Time series to forecast
p:Price signals of Streamline Health Solutions stock
j:Nash equilibria (Neural Network)
k:Dominated move of Streamline Health Solutions stock holders
a:Best response for Streamline Health Solutions target price
For further technical information as per how our model work we invite you to visit the article below:
How do KappaSignal algorithms actually work?
Streamline Health Solutions Stock Forecast (Buy or Sell) Strategic Interaction Table
Strategic Interaction Table Legend:
X axis: *Likelihood% (The higher the percentage value, the more likely the event will occur.)
Y axis: *Potential Impact% (The higher the percentage value, the more likely the price will deviate.)
Z axis (Grey to Black): *Technical Analysis%
Streamline Health Solutions Inc. (STRM) Financial Outlook and Forecast
The financial outlook for Streamline Health (STRM) presents a mixed picture, largely hinging on its ability to effectively execute its strategic initiatives within the complex healthcare technology market. Recent performance indicates potential for growth, but also highlights challenges. The company's focus on revenue cycle management (RCM) solutions, particularly its enterprise performance analytics platform, aims to improve healthcare provider efficiency and financial outcomes. STRM's revenue streams primarily come from software licensing, related professional services, and ongoing maintenance and support. The demand for such solutions is expected to remain robust, driven by the industry's continuous need to optimize operations, address regulatory complexities, and reduce costs. Factors such as the increasing adoption of value-based care models, which emphasizes outcomes over volume, further support the need for advanced analytics and RCM tools. However, achieving consistent profitability has been an ongoing concern. STRM's ability to scale its operations efficiently, manage its cost structure, and secure larger, longer-term contracts will be critical to achieving sustainable financial success.
STRM's future financial performance is expected to be significantly influenced by its ability to win new business, retain existing clients, and expand its market share within the competitive healthcare IT landscape. Expansion of its client base, especially among large hospitals and integrated healthcare systems, is key to driving revenue growth. The effectiveness of its sales and marketing efforts, along with its ability to differentiate its offerings from competitors through technological innovation and superior customer service, will be vital. Partnerships and strategic acquisitions could play a role in accelerating growth and expanding market reach. The ability to successfully integrate any acquired entities will also be important. Furthermore, the company's investments in research and development will be essential to keep pace with evolving industry needs and technology advancements. Continued focus on cloud-based solutions and leveraging data analytics to improve service offerings is anticipated.
Key financial metrics to monitor include revenue growth, gross margins, operating expenses as a percentage of revenue, and cash flow generation. Improvement in gross margins would suggest greater operational efficiency, and revenue growth provides a strong signal for long-term financial health. Managing operating expenses, particularly sales and marketing and research and development costs, will be essential for achieving profitability. Consistent positive cash flow is crucial for funding operations, investments, and any potential strategic initiatives. Investors and analysts should pay close attention to the company's backlog of contracted revenue, which provides insight into future revenue streams, and the effectiveness of its customer retention strategies. These metrics will offer valuable insights into the company's financial trajectory.
Overall, the financial forecast for STRM is cautiously optimistic. Assuming the company successfully executes its growth strategy, navigates the competitive landscape, and continues to innovate, it has the potential for moderate revenue growth and improved profitability over the next few years. However, there are significant risks to this prediction. These include: the potential for delays in sales cycles due to the complexity of healthcare IT decisions, the intensity of competition from larger, more established players, and the risk of increased operating costs associated with scaling its business. Macroeconomic factors, regulatory changes within the healthcare industry, and changes in technology may also play a role in the company's financial health. Therefore, while there is potential for upside, investors should remain vigilant and carefully monitor the company's progress against its strategic objectives.
```
Rating | Short-Term | Long-Term Senior |
---|---|---|
Outlook | B3 | B2 |
Income Statement | Caa2 | B3 |
Balance Sheet | Baa2 | B2 |
Leverage Ratios | C | C |
Cash Flow | C | Baa2 |
Rates of Return and Profitability | C | C |
*Financial analysis is the process of evaluating a company's financial performance and position by neural network. It involves reviewing the company's financial statements, including the balance sheet, income statement, and cash flow statement, as well as other financial reports and documents.
How does neural network examine financial reports and understand financial state of the company?
References
- J. Ott. A Markov decision model for a surveillance application and risk-sensitive Markov decision processes. PhD thesis, Karlsruhe Institute of Technology, 2010.
- Hastie T, Tibshirani R, Wainwright M. 2015. Statistical Learning with Sparsity: The Lasso and Generalizations. New York: CRC Press
- Chipman HA, George EI, McCulloch RE. 2010. Bart: Bayesian additive regression trees. Ann. Appl. Stat. 4:266–98
- Jorgenson, D.W., Weitzman, M.L., ZXhang, Y.X., Haxo, Y.M. and Mat, Y.X., 2023. Can Neural Networks Predict Stock Market?. AC Investment Research Journal, 220(44).
- Jorgenson, D.W., Weitzman, M.L., ZXhang, Y.X., Haxo, Y.M. and Mat, Y.X., 2023. MRNA: The Next Big Thing in mRNA Vaccines. AC Investment Research Journal, 220(44).
- Nie X, Wager S. 2019. Quasi-oracle estimation of heterogeneous treatment effects. arXiv:1712.04912 [stat.ML]
- Athey S. 2019. The impact of machine learning on economics. In The Economics of Artificial Intelligence: An Agenda, ed. AK Agrawal, J Gans, A Goldfarb. Chicago: Univ. Chicago Press. In press