AUC Score :
Short-term Tactic1 :
Dominant Strategy :
Time series to forecast n:
ML Model Testing : Inductive Learning (ML)
Hypothesis Testing : Ridge Regression
Surveillance : Major exchange and OTC
1Short-term revised.
2Time series is updated based on short-term trends.
Key Points
ST's future appears promising, fueled by its leading position in the home services software market and consistent revenue growth. The company is likely to continue expanding its customer base and increasing its ARPU through product enhancements and market penetration. Potential risks include intense competition from both established players and emerging startups, economic downturns affecting home service spending, and challenges in integrating acquired companies. Furthermore, evolving cybersecurity threats pose a risk to the data and reputation of the company. Also, investor sentiment shifts and valuation adjustments could occur if growth expectations are not met.About ServiceTitan
ServiceTitan, Inc. is a prominent software company specializing in business management solutions for the home and commercial service industries. Founded in 2012, the company provides a cloud-based platform designed to streamline operations, improve customer experience, and enhance profitability for businesses involved in areas such as plumbing, HVAC, electrical, and other field services. Its software encompasses scheduling, dispatching, customer relationship management (CRM), marketing, accounting, and other essential functionalities tailored to the unique needs of service businesses.
The company's platform empowers contractors to efficiently manage their entire workflow, from initial customer contact to job completion and invoicing. By leveraging technology, ServiceTitan aims to help its clients optimize resource allocation, reduce administrative overhead, and make data-driven decisions. ServiceTitan has experienced significant growth and secured substantial funding from investors, reflecting its strong position within the home services software market. The company's success is largely attributed to its specialized focus and commitment to providing comprehensive solutions for a specific sector.
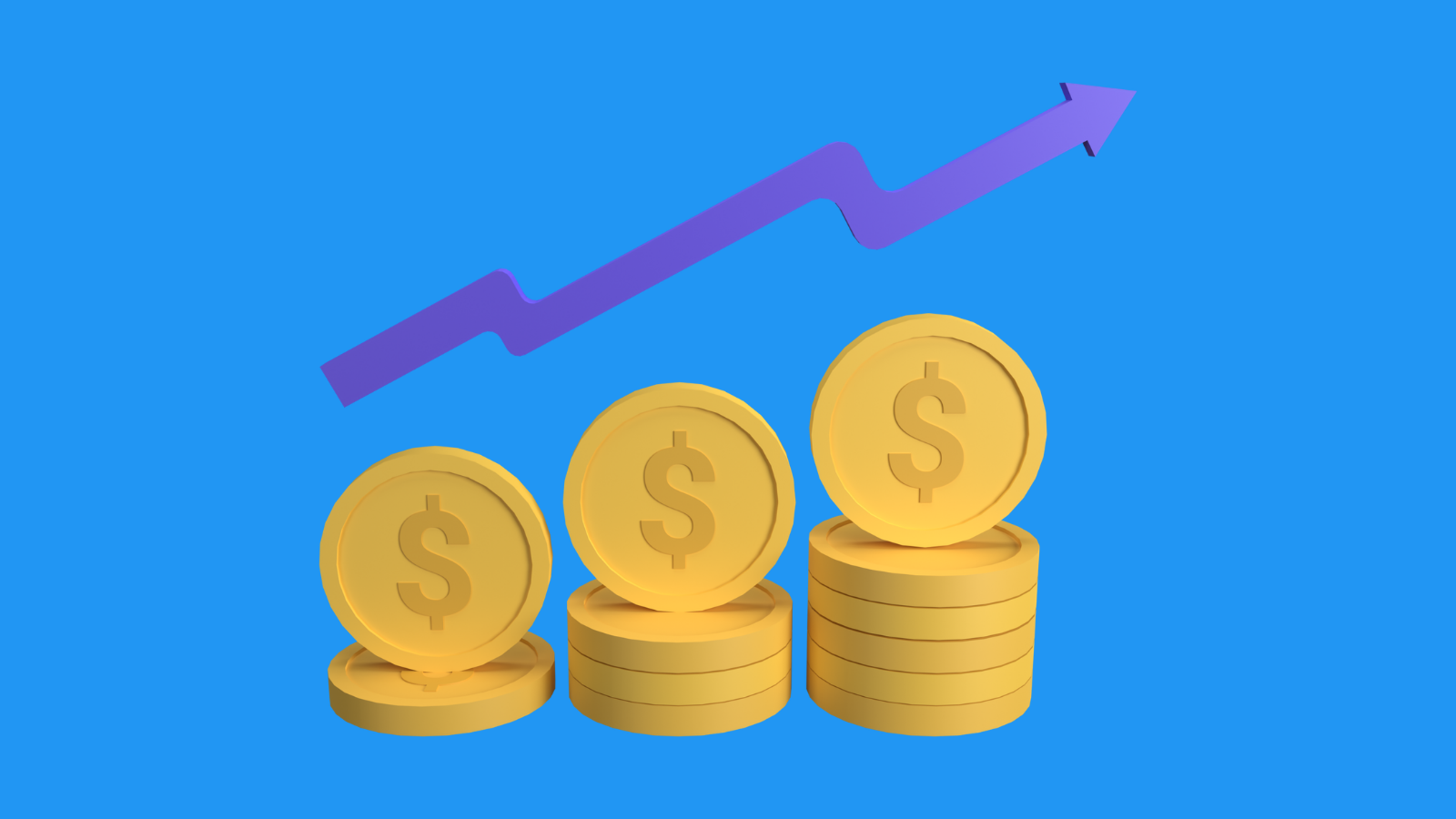
TTAN Stock Forecast Model
Our team, comprising data scientists and economists, has developed a machine learning model to forecast the performance of ServiceTitan Inc. Class A Common Stock (TTAN). The model leverages a comprehensive dataset, incorporating both internal and external factors. Internal data includes ServiceTitan's financial statements (revenue, expenses, profitability metrics), customer acquisition and retention rates, product development milestones, and operational efficiency indicators. External data encompasses macroeconomic indicators such as GDP growth, inflation rates, interest rates, and industry-specific data like SaaS sector trends, competitive landscape analysis, and investor sentiment captured from news articles and social media. We employ a combination of machine learning techniques, including Time Series Analysis, Regression models (e.g., Gradient Boosting, Random Forest), and Natural Language Processing (NLP) for sentiment analysis from news sources. The selection of the final model is based on rigorous backtesting and evaluation metrics like Mean Squared Error (MSE) and R-squared to ensure robust performance and reliability.
The model's architecture includes several key components. First, data preprocessing involves cleaning, transforming, and normalizing the raw data to ensure consistency and address missing values. Feature engineering is crucial, creating new variables from existing ones, such as calculating growth rates, profitability ratios, and market share. The time series component is used to capture historical trends and seasonality in TTAN's performance. The regression models incorporate both financial and macroeconomic variables to forecast the stock's behavior based on internal strengths and external changes. Sentiment analysis results extracted from NLP analysis, also included as features, capture the overall investor optimism or pessimism regarding the company. The model provides probabilistic forecasts, including the predicted value along with confidence intervals, allowing for a nuanced understanding of the potential range of outcomes.
The model is designed to generate forecasts over multiple time horizons (e.g., daily, weekly, and monthly). Regular model retraining is integral, done as new data becomes available, and incorporates feedback from ongoing performance evaluations. Model validation includes holdout testing, cross-validation, and out-of-sample testing to ensure that performance metrics consistently meet the required targets. Further enhancement includes exploring advanced techniques like deep learning, incorporating alternative data sources (e.g., web traffic, employee reviews), and refining feature selection through iterative experimentation. Finally, the model's output will be presented as informative insights to the stakeholders, allowing a better understanding of the company's situation and performance.
ML Model Testing
n:Time series to forecast
p:Price signals of ServiceTitan stock
j:Nash equilibria (Neural Network)
k:Dominated move of ServiceTitan stock holders
a:Best response for ServiceTitan target price
For further technical information as per how our model work we invite you to visit the article below:
How do KappaSignal algorithms actually work?
ServiceTitan Stock Forecast (Buy or Sell) Strategic Interaction Table
Strategic Interaction Table Legend:
X axis: *Likelihood% (The higher the percentage value, the more likely the event will occur.)
Y axis: *Potential Impact% (The higher the percentage value, the more likely the price will deviate.)
Z axis (Grey to Black): *Technical Analysis%
ServiceTitan Inc. Class A Common Stock: Financial Outlook and Forecast
Servicetitan, a leading software provider for the trades, demonstrates a promising financial outlook underpinned by several key factors. The company is positioned within a high-growth market, driven by the increasing adoption of technology in home services, which includes plumbing, HVAC, electrical, and other trades. This transition from traditional, paper-based operations to digital platforms like Servicetitan's is a significant driver of revenue expansion. Servicetitan's platform offers a comprehensive suite of tools, including scheduling, dispatching, customer management, and accounting features, making it an attractive solution for trade businesses seeking to improve efficiency, profitability, and customer satisfaction. The company's focus on a specific niche allows for deep specialization and targeted marketing efforts, which could lead to higher customer acquisition and retention rates. Servicetitan has consistently shown strong revenue growth, indicating its ability to capture market share and capitalize on the digital transformation trend within the trades industry. Their commitment to innovation further enhances its appeal, offering new functionalities and integrations, ensuring that their platform adapts to the evolving needs of their customer base.
The company's financial performance indicates a positive trajectory, with growing subscription revenues and a steadily expanding customer base. Recurring revenue streams, characteristic of a software-as-a-service (SaaS) business model, provide stability and predictability, facilitating long-term financial planning. Servicetitan's ability to upsell and cross-sell additional features and services contributes to its revenue growth. Strategic partnerships and integrations with other industry players enhance its platform's value and expand its reach. By building strong customer relationships and offering robust support services, Servicetitan has cultivated customer loyalty, leading to increased customer lifetime value. In addition, the company's focus on operational efficiency, especially as it expands its platform, can lead to enhanced profitability. Management's strategic choices in areas like sales and marketing, product development, and geographical expansion will play an important role in shaping future financial outcomes.
Looking ahead, Servicetitan's growth prospects are contingent on a number of factors. Expansion into new geographical markets, both domestically and internationally, presents considerable opportunities for customer base growth. The company's ongoing investment in research and development and product enhancement is critical to maintain a competitive edge and adapt to evolving market demands. Effective sales and marketing strategies will be crucial for attracting new customers and expanding market share. Moreover, their ability to integrate their platform with new third-party tools, such as AI assistants, may generate interest and new sales. Servicetitan could also become more prominent, with opportunities for further acquisitions, providing them with the ability to expand both their capabilities and addressable market. Careful management of its resources, including cost control and capital allocation, will be critical for sustaining long-term financial performance. Overall, Servicetitan's success will depend on its ability to scale its operations effectively while maintaining its focus on product innovation and customer satisfaction.
In conclusion, the financial outlook for Servicetitan appears positive. The company is well-positioned to benefit from the continued adoption of technology in the trades sector, supported by its strong market position, recurring revenue model, and a growing customer base. A positive outlook is projected, assuming the company can effectively execute its growth strategies and adapt to the evolving competitive landscape. However, the outlook also carries risks. Increased competition from existing and emerging players could potentially reduce market share and margins. Economic downturns or fluctuations in construction activity could impact demand for its services. The pace of technological change and the need for continuous innovation also pose risks. Successfully mitigating these risks through effective management and strategic planning is essential for the long-term success of the company.
Rating | Short-Term | Long-Term Senior |
---|---|---|
Outlook | Ba3 | B1 |
Income Statement | Baa2 | Baa2 |
Balance Sheet | Baa2 | Baa2 |
Leverage Ratios | C | Caa2 |
Cash Flow | Ba1 | C |
Rates of Return and Profitability | Ba2 | Caa2 |
*Financial analysis is the process of evaluating a company's financial performance and position by neural network. It involves reviewing the company's financial statements, including the balance sheet, income statement, and cash flow statement, as well as other financial reports and documents.
How does neural network examine financial reports and understand financial state of the company?
References
- Mnih A, Hinton GE. 2007. Three new graphical models for statistical language modelling. In International Conference on Machine Learning, pp. 641–48. La Jolla, CA: Int. Mach. Learn. Soc.
- Athey S, Imbens GW. 2017b. The state of applied econometrics: causality and policy evaluation. J. Econ. Perspect. 31:3–32
- J. Filar, D. Krass, and K. Ross. Percentile performance criteria for limiting average Markov decision pro- cesses. IEEE Transaction of Automatic Control, 40(1):2–10, 1995.
- Chen X. 2007. Large sample sieve estimation of semi-nonparametric models. In Handbook of Econometrics, Vol. 6B, ed. JJ Heckman, EE Learner, pp. 5549–632. Amsterdam: Elsevier
- J. Ott. A Markov decision model for a surveillance application and risk-sensitive Markov decision processes. PhD thesis, Karlsruhe Institute of Technology, 2010.
- Chernozhukov V, Chetverikov D, Demirer M, Duflo E, Hansen C, et al. 2016a. Double machine learning for treatment and causal parameters. Tech. Rep., Cent. Microdata Methods Pract., Inst. Fiscal Stud., London
- E. Altman, K. Avrachenkov, and R. N ́u ̃nez-Queija. Perturbation analysis for denumerable Markov chains with application to queueing models. Advances in Applied Probability, pages 839–853, 2004